Multi-stage part-aware graph convolutional network for skeleton-based action recognition
IET IMAGE PROCESSING(2022)
摘要
Recently, graph convolutional networks have shown excellent results in skeleton-based action recognition. This paper presents a multi-stage part-aware graph convolutional network for the problems of model over complication, parameter redundancy and lack of long-dependence feature information. The structure of this network has a multi-stream input and two-stream output, which can greatly reduce the complexity and improve the accuracy of the model without losing sequence information. The two branches of the network have the same backbone, which includes 6 multi-order feature extraction blocks and 3 temporal attention calibration blocks, and the outputs of the two branches are fused together. In multi-order feature extraction block, a channel-spatial attention mechanism and a graph condensation module are proposed, which can extract more distinguishable feature and identify the relationship between parts. In temporal attention calibration block, the temporal dependencies between frames in the skeleton sequence are modeled. Experimental results show that the proposed network outperforms many mainstream methods on NTU and Kinetics datasets, for example, it achieves 92.4% accuracy on the cross-subject benchmark of NTU-RGBD60 dataset.
更多查看译文
AI 理解论文
溯源树
样例
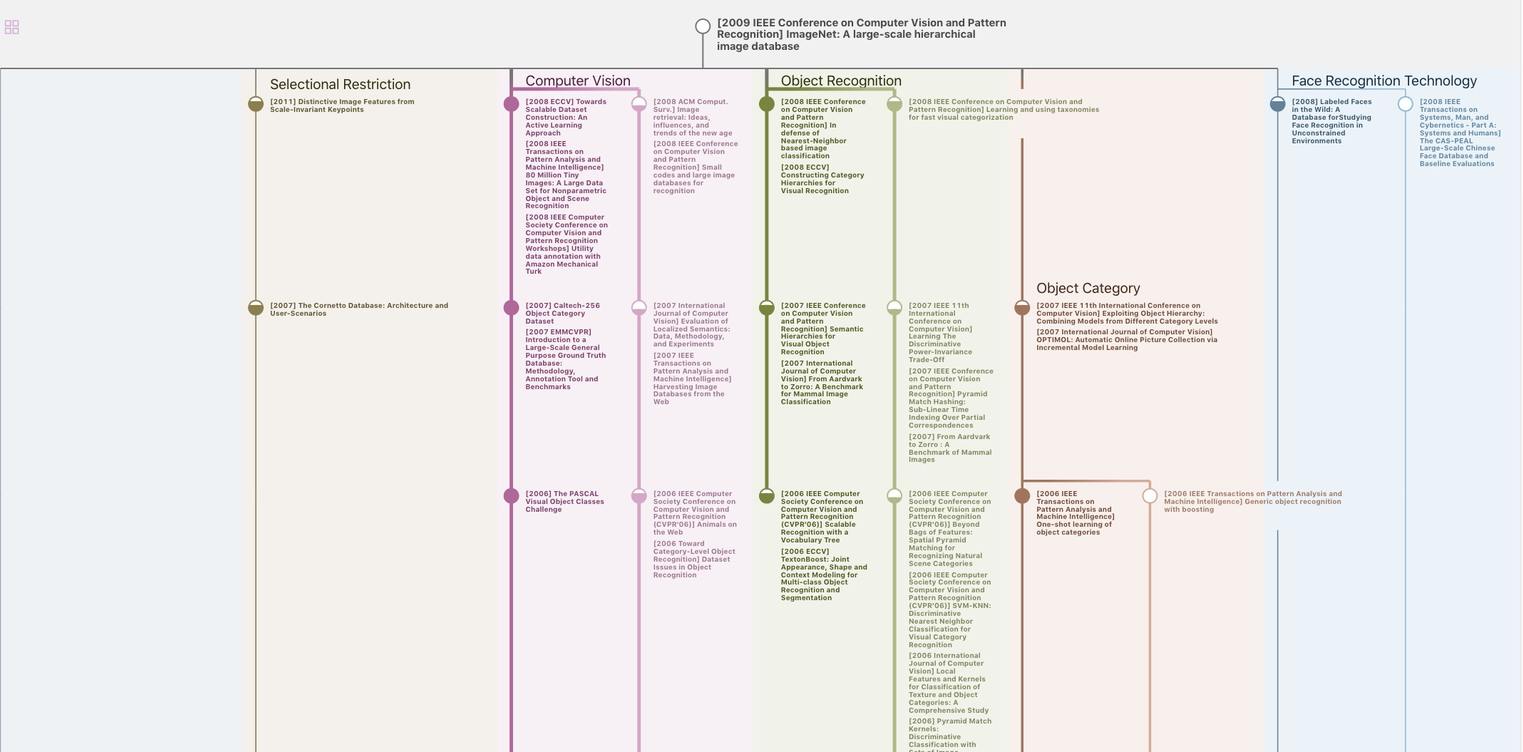
生成溯源树,研究论文发展脉络
Chat Paper
正在生成论文摘要