Scalable Tabular Metadata Location and Classification in Large-Scale Structured Datasets
DATABASE AND EXPERT SYSTEMS APPLICATIONS, DEXA 2021, PT I(2021)
摘要
Tabular metadata (i.e. attribute names) location and classification is a fundamental problem for large-scale structured corpora. Web tables [24], CORD-19 [35], have thousands to millions of tables, but often have missing or incorrect labels for rows (or columns) with attribute names (e.g. Last Name). Missing or incorrect metadata labels [19] prevent or at least significantly complicate the fundamental data management tasks such as query processing, data integration, indexing, and many other. Different sources position metadata rows/columns differently inside a table, which makes its reliable identification challenging. In this work we describe a scalable, hybrid two-layer Deep- and Machine-learning based ensemble, combining Long Short Term Memory (LSTM) and Naive Bayes Classifier to accurately identify Metadata-containing rows or columns in a table. We have performed an extensive evaluation on several structures datasets, including an ultra large-scale dataset containing more than 15 million tables coming from more than 26 thousands of sources to justify scalability and resistance to heterogeneity, stemming from a large number of sources. We observed superiority of this two-layer ensemble, compared to the recent previous approaches and report an impressive 81.53% accuracy at scale.
更多查看译文
关键词
Hierarchical metadata, Metadata classification, Web table
AI 理解论文
溯源树
样例
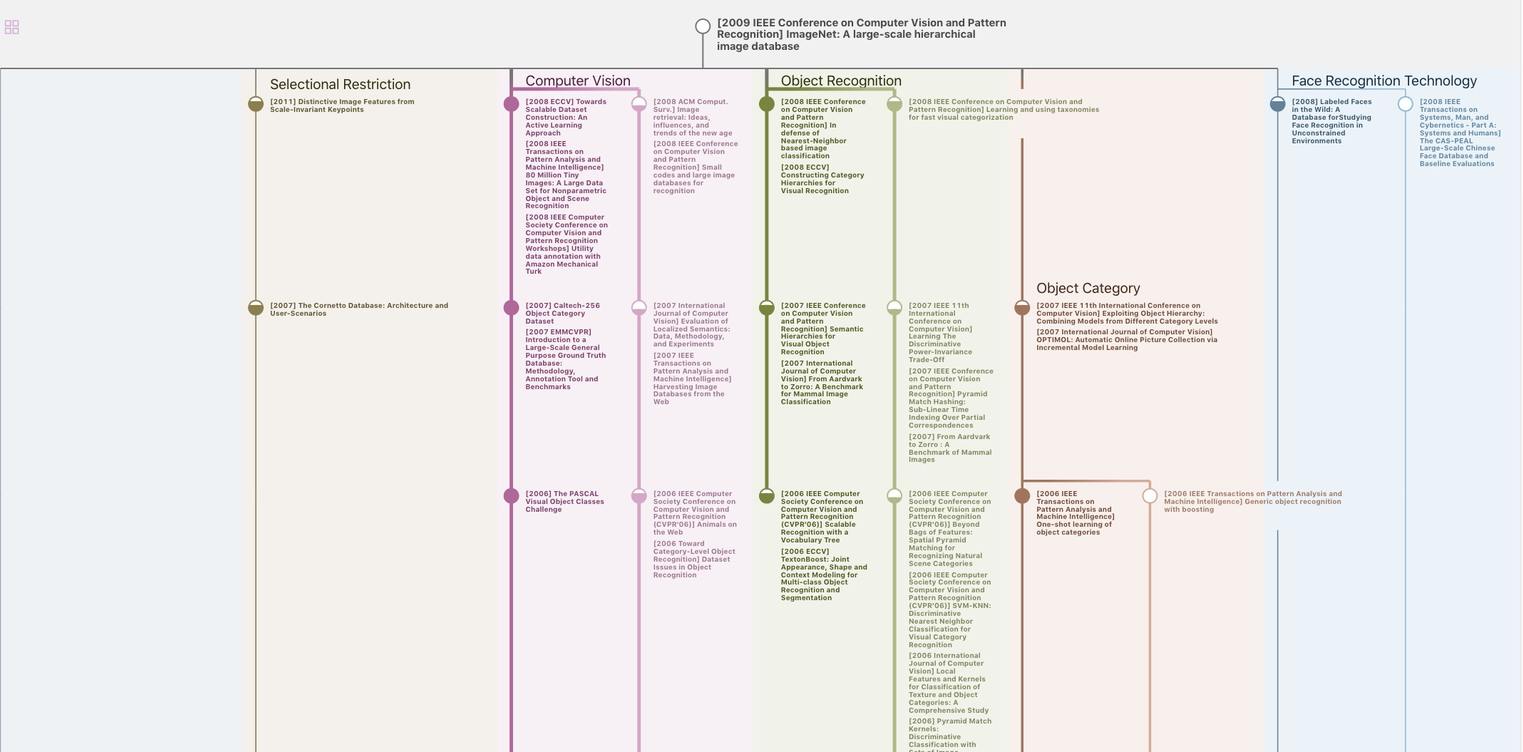
生成溯源树,研究论文发展脉络
Chat Paper
正在生成论文摘要