Dealing with data intrinsic difficulties by learning an interPretable Ensemble Rule Learning (PERL) model
Information Sciences(2022)
摘要
One of the challenges of learning algorithms is data imbalance, especially data intrinsic difficulties such as borderline, rare, and outlier examples. In order to improve the performance of learning algorithms in such cases, various solutions have been proposed, one of which is ensemble learning. Despite the good results of ensemble learning, their results are not interpretable. In some domains, such as medicine, banking, and telecommunications, the interpretability of the final model results is very important. For this purpose, rule-based learning algorithms and decision trees are two appropriate approaches. Therefore, an interPretable Ensemble Rule Learning (PERL) model is proposed in this paper. PERL has three main phases: sampling, rule learning, and rule processing. We reduce the negative effects of data intrinsic difficulties in each phase by an appropriate solution. PERL is compared with several interpretable ensemble models on ten imbalanced real-world datasets. According to the obtained results, PERL outperforms significantly the other models based on the f1-score measure.
更多查看译文
关键词
Data intrinsic difficulties,Imbalanced dataset,SMOTE,Ensemble learning,Rule learning,Interpretability
AI 理解论文
溯源树
样例
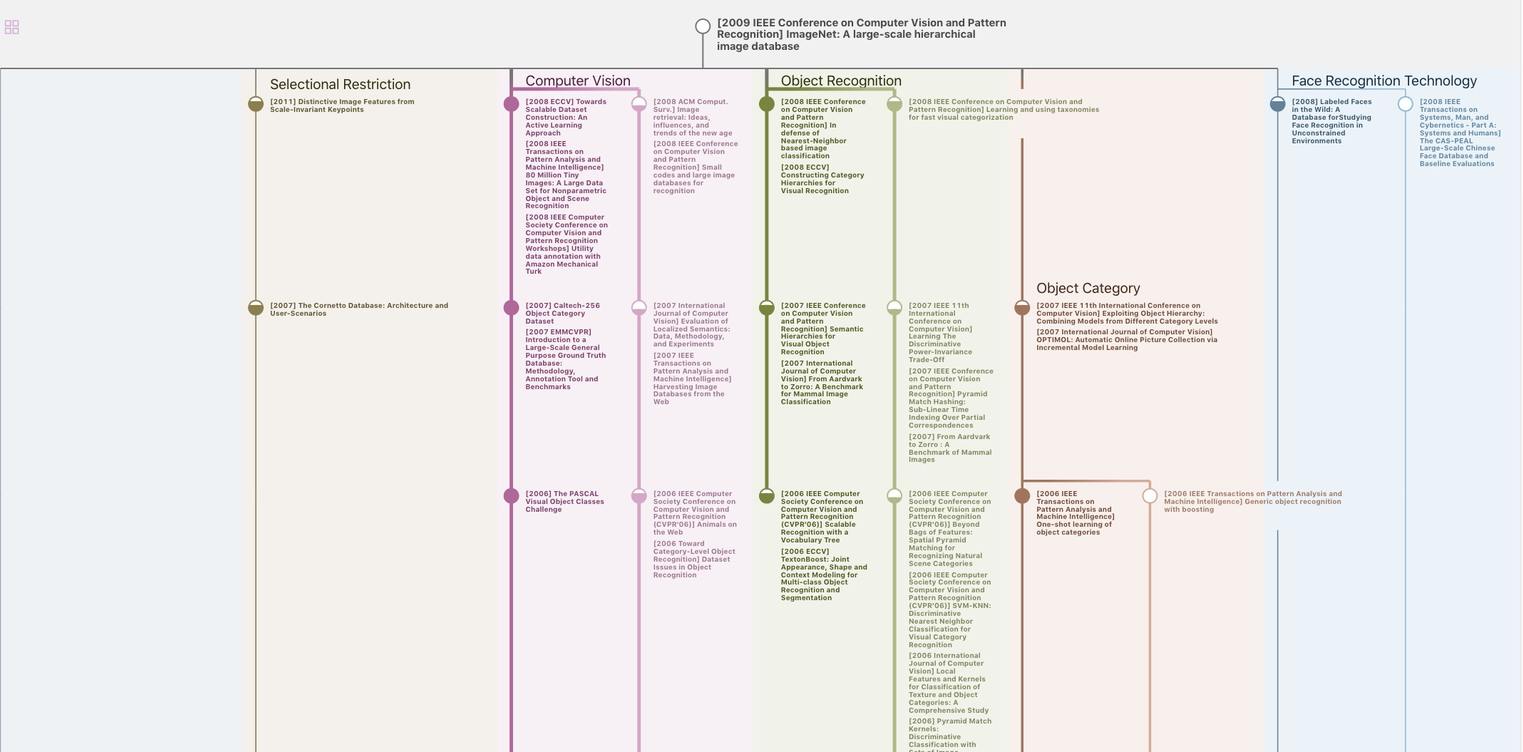
生成溯源树,研究论文发展脉络
Chat Paper
正在生成论文摘要