A Novel Multi-Stage Ensemble Model With a Hybrid Genetic Algorithm for Credit Scoring on Imbalanced Data
IEEE ACCESS(2021)
摘要
Credit scoring models are the cornerstone of the modern financial industry. After years of development, artificial intelligence and machine learning have led to the transformation of traditional credit scoring models based on statistics. In this study, a novel multi-stage ensemble model with a hybrid genetic algorithm is proposed to achieve accurate and stable credit prediction. To alleviate the adverse effects of imbalanced data in credit scoring models, the Instance Hardness Threshold method is extended using a majority voting strategy to deal with data imbalance. To eliminate redundant and irrelevant features in the dataset and select well-performing base classifiers, a new hybrid genetic algorithm is proposed to obtain the optimal feature subset and base classifier subset. To aggregate the predictive power of the base classifiers, a stacking approach is adopted to integrate the optimal base classifiers into the ensemble model. The proposed model is tested on three standard imbalanced credit scoring datasets, compared with similar state-of-the-art approaches, and evaluated using four well-known evaluation indicators. The experimental results prove the effectiveness of the proposed model and demonstrate its superiority.
更多查看译文
关键词
Data models,Genetic algorithms,Predictive models,Feature extraction,Stacking,Training,Sociology,Credit scoring,imbalanced data,genetic algorithm,ensemble model
AI 理解论文
溯源树
样例
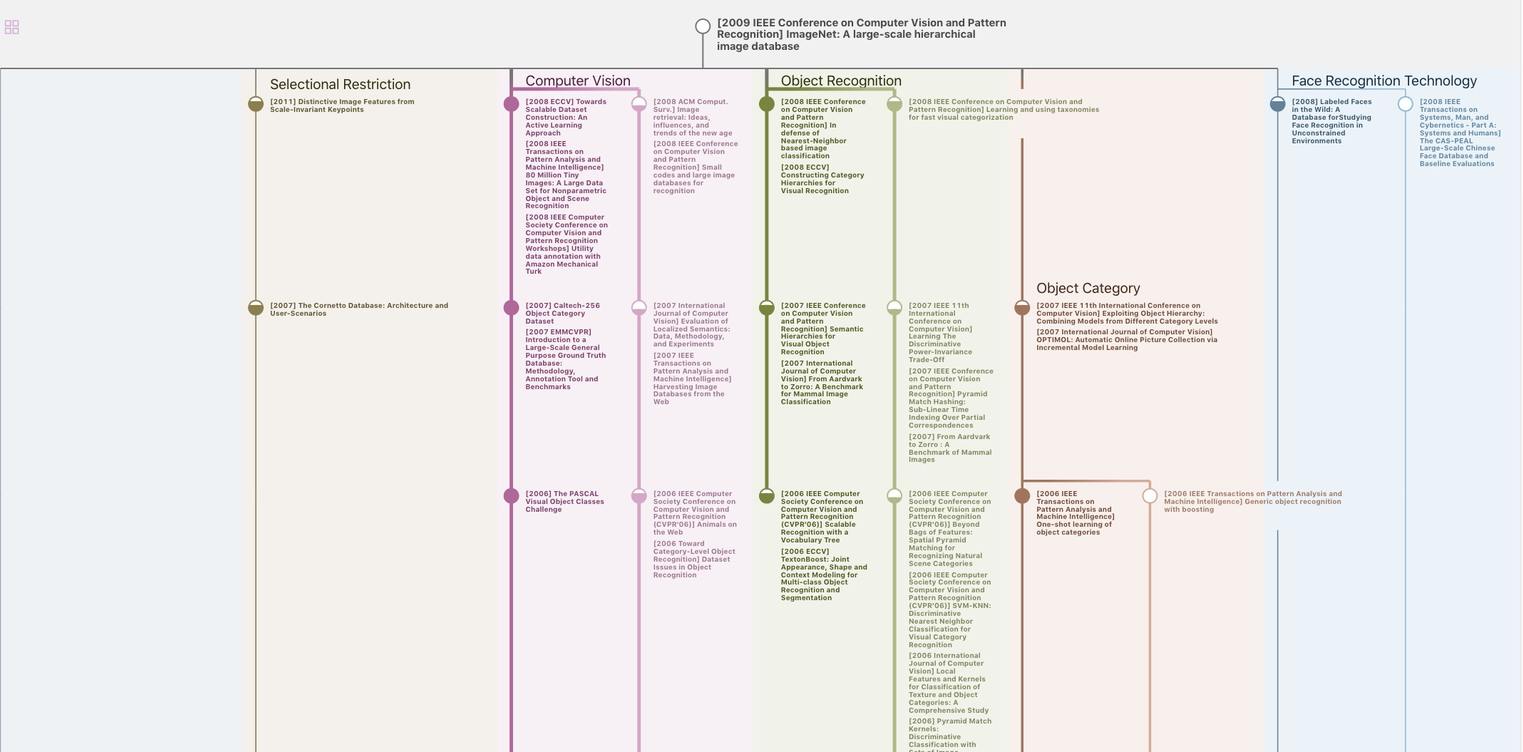
生成溯源树,研究论文发展脉络
Chat Paper
正在生成论文摘要