Impact of Two Lung Elastance Identification Methods on Pulmonary Mechanics Prediction
IFAC-PapersOnLine(2021)
摘要
Positive-end-expiratory-pressure (PEEP) have proved effective in recruiting lung volume and keeping alveoli open. However, there is no standard means to find an optimal patient-specific PEEP, creating variability in care and outcomes. There is thus a need for personalized approaches to find the best PEEP and optimise care. This research extends a well-validated virtual patient model with a newly proposed function to predict lung distension, while the impact on outcome of two different elastance identification strategies are discussed and compared. A prior studied and effective exponential basis function set is used as the general model, while elastance are identified using overlapped and separate methods, respectively. In this approach, model with overlapped elastance identification and proposed distension function yields an absolute median peak inspiratory pressure (PIP) prediction error of 1.50cmH2O for 623 prediction cases. Comparison between clinically measurement and model prediction for PIP yields R2=0.90 across 623 predictions in total, while R2=0.87 with separate elastance identification. Furthermore, both elastance identification methods are an improvement compared to predictions without proposed distension function (R2=0.82). Validation is fulfilled with 18 volume controlled ventilation patients respiratory data at 7 different baseline PEEP levels (0-12cmH2O) with a maximal PEEP prediction interval of 12cmH2O. Overall, the results demonstrate the impact of elastance identification methods, as well as the potential and significance for accurately capturing distension mechanics, which thus providing guidance for clinical care and insights for predictive lung mechanics modelling.
更多查看译文
关键词
Virtual Patient,Digital Twin,Mechanical ventilation,Critical Care,Basis function,Prediction,Elastance identification,Lung distension,VILI,Pressure-Volume loop
AI 理解论文
溯源树
样例
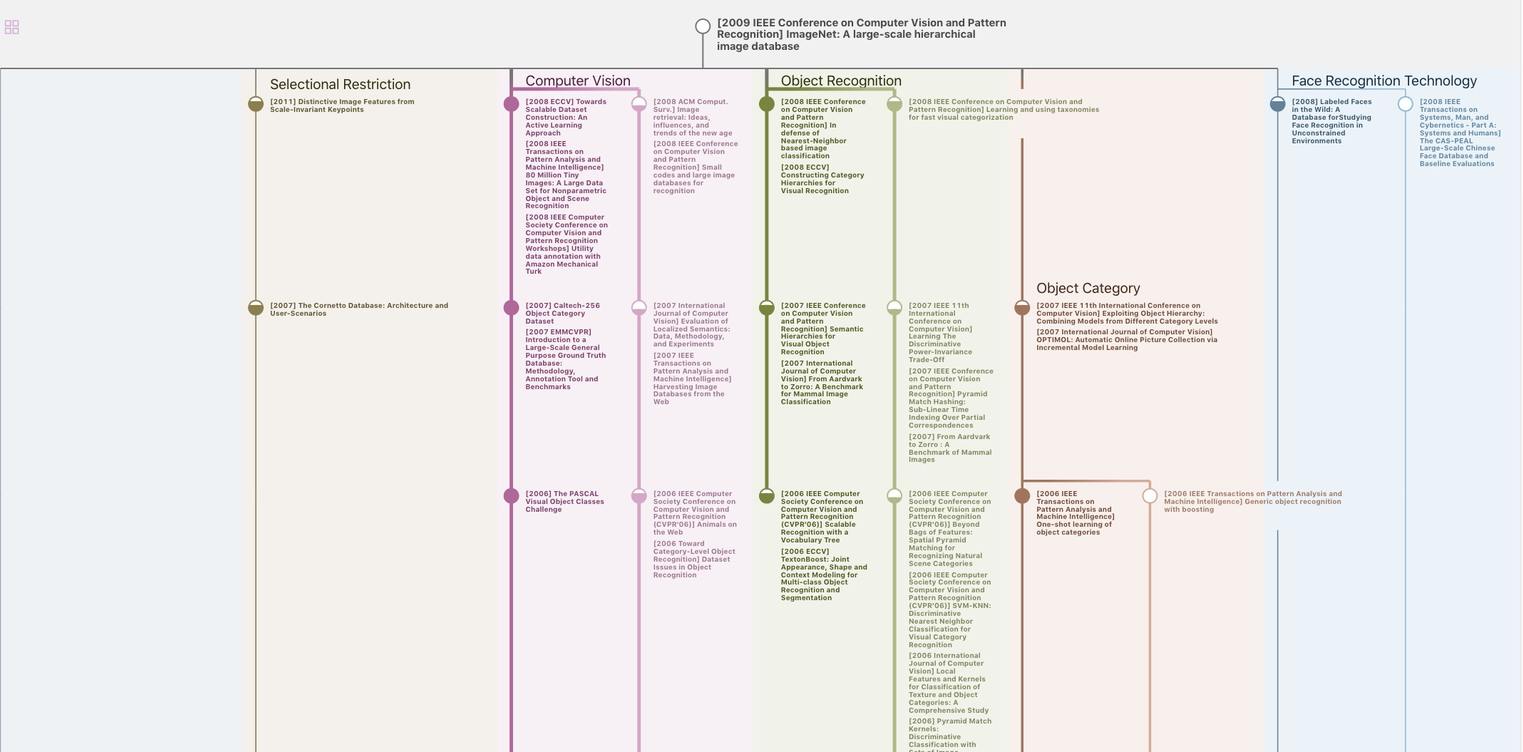
生成溯源树,研究论文发展脉络
Chat Paper
正在生成论文摘要