Solar Irradiance Forecasting Using Dynamic Ensemble Selection
APPLIED SCIENCES-BASEL(2022)
摘要
Solar irradiance forecasting has been an essential topic in renewable energy generation. Forecasting is an important task because it can improve the planning and operation of photovoltaic systems, resulting in economic advantages. Traditionally, single models are employed in this task. However, issues regarding the selection of an inappropriate model, misspecification, or the presence of random fluctuations in the solar irradiance series can result in this approach underperforming. This paper proposes a heterogeneous ensemble dynamic selection model, named HetDS, to forecast solar irradiance. For each unseen test pattern, HetDS chooses the most suitable forecasting model based on a pool of seven well-known literature methods: ARIMA, support vector regression (SVR), multilayer perceptron neural network (MLP), extreme learning machine (ELM), deep belief network (DBN), random forest (RF), and gradient boosting (GB). The experimental evaluation was performed with four data sets of hourly solar irradiance measurements in Brazil. The proposed model attained an overall accuracy that is superior to the single models in terms of five well-known error metrics.
更多查看译文
关键词
solar irradiance forecasting, heterogeneous ensemble dynamic selection model, neural networks
AI 理解论文
溯源树
样例
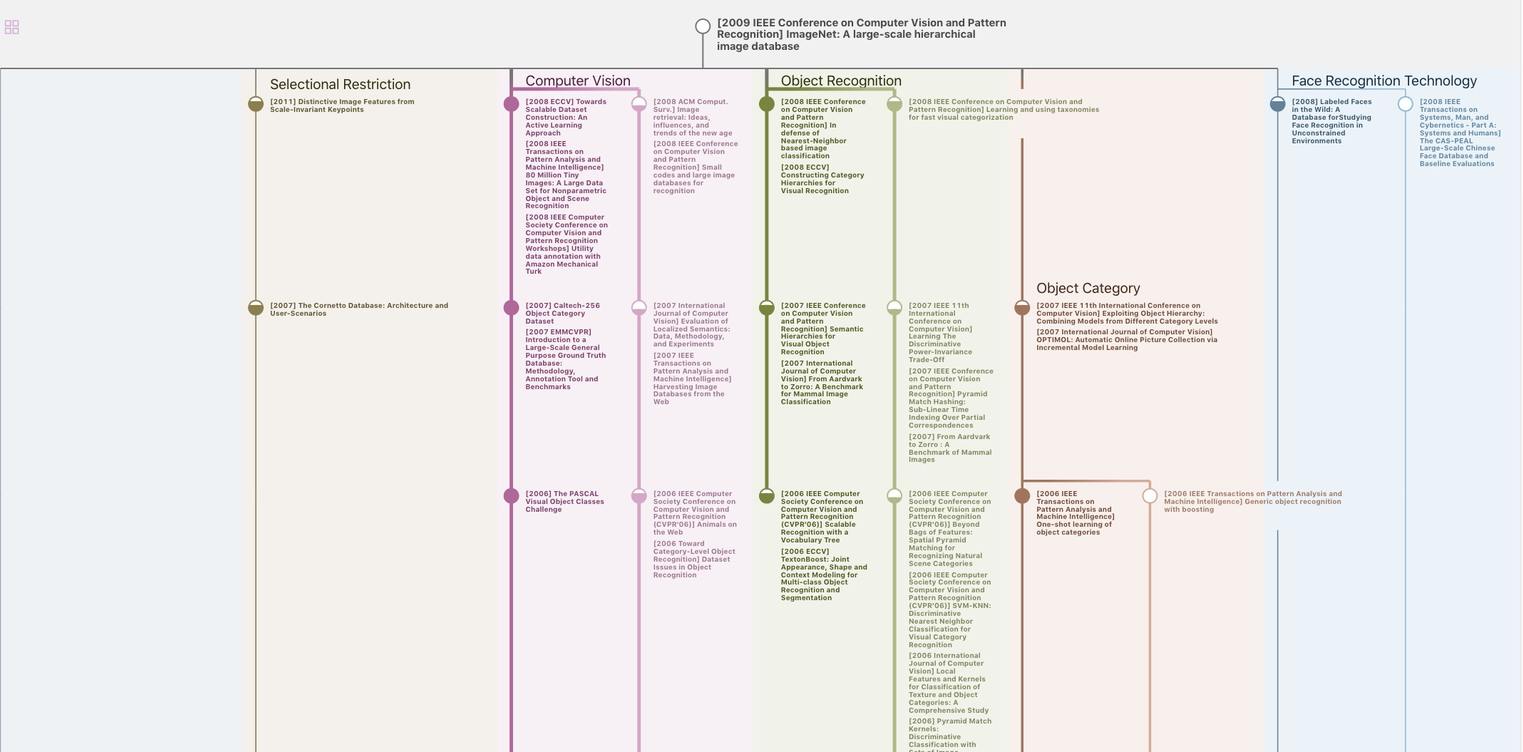
生成溯源树,研究论文发展脉络
Chat Paper
正在生成论文摘要