A Reinforcement Learning Based Adaptive ROI Generation for Video Object Segmentation
IEEE ACCESS(2021)
摘要
Video object segmentation's primary goal is to automatically extract the principal object(s) in the foreground from the background in videos. The primary focus of the current deep learning-based models is to learn the discriminative representations in the foreground over motion and appearance in small-term temporal segments. In the video segmentation process, it is difficult to handle various challenges such as deformation, scale variation, motion blur, and occlusion. Furthermore, relocating the segmentation target in the next frame is difficult if it is lost in the current frame during the segmentation process. This work aims at solving the zero-shot video object segmentation issue in a holistic fashion. We take advantage of the inherent correlations between the video frames by incorporating a global co-attention mechanism to overcome the limitations. We propose a novel reinforcement learning framework that provides competent and fast stages for gathering scene context and global correlations. The agent concurrently calculates and adds the responses of co-attention in the joint feature space. To capture the different aspects of the common feature space, the agent can generate multiple co-attention versions. Our framework is trained using pairs (or groups) of video frames, which adds to the training content, thus increasing the learning capacity. Our approach encodes the important information during the segmentation phase by a simultaneous process of various reference frames that are subsequently utilized to predict the persistent and conspicuous objects in the foreground. The proposed method has been validated using four commonly used video entity segmentation datasets: SegTrack V2, DAVIS 2016, CdNet 2014, and the Youtube-Object dataset. On the DAVIS 2016, the results reveal that the proposed results boost the state-of-the-art techniques on the F1 Measure by 4%, SegTrack V2 by a Jaccard Index of 12.03%, and Youtube Object by a Jaccard Index of 13.11%. Meanwhile, our algorithm improves the accuracy by 8%, F1 Measure by 12.25 %, and precision by 14% on the CdNet 2014, thus ranking higher than the current state-of-the-art methods.
更多查看译文
关键词
Motion segmentation, Correlation, Feature extraction, Object segmentation, Computational modeling, Reinforcement learning, Training, Model adaptation, object detection, object tracking, reinforcement learning, video object segmentation
AI 理解论文
溯源树
样例
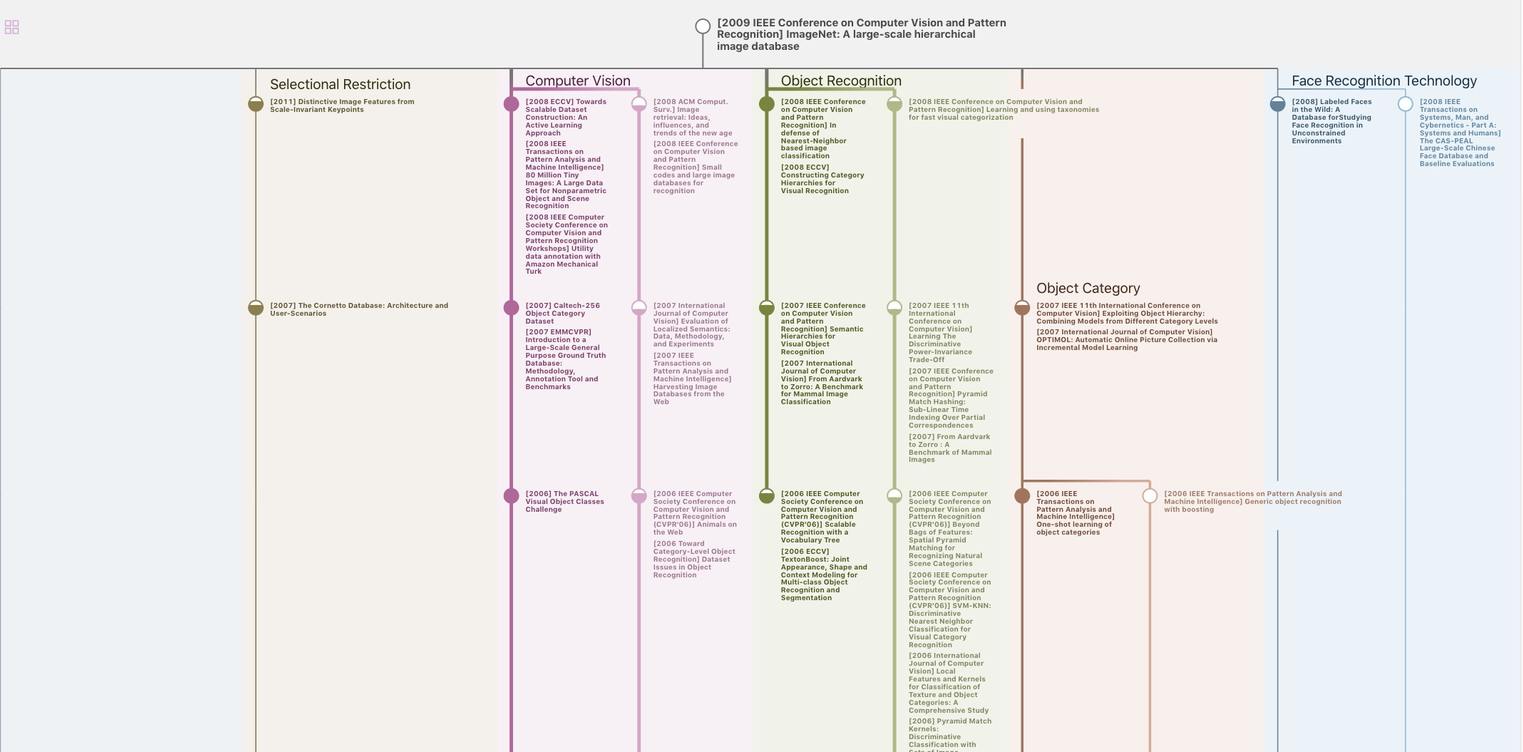
生成溯源树,研究论文发展脉络
Chat Paper
正在生成论文摘要