Optimal subsampling for large-sample quantile regression with massive data
CANADIAN JOURNAL OF STATISTICS-REVUE CANADIENNE DE STATISTIQUE(2023)
摘要
To balance the explosive growth of data volume and limited budgets for computational resources, one of the popular methods is downscaling the data volume by subsampling a subdataset that inherits the relevant property of the full data. As an alternative to the mean regression model, the quantile regression model has been studied extensively when the data are independent and the data scale is medium. This article focuses on quantile regression with massive data where the sample size n (greater than 106 in general) is extraordinarily large but the dimension d (smaller than 20 in general) is small. We first formulate the general subsampling procedure and establish the asymptotic property of the resultant estimator. Then, with the help of optimality criteria in experimental design, we derive two subsampling probabilities that are optimal in the sense of smallest asymptotic mean square error. Since the optimal subsampling probabilities depend on the full data estimator, we develop a two-step optimal subsampling algorithm and study the consistency and asymptotic normality of the resultant estimator. The empirical performance of the optimal subsampling algorithm is evaluated with synthetic and real datasets.
更多查看译文
关键词
Massive data,optimal subsampling,quantile regression
AI 理解论文
溯源树
样例
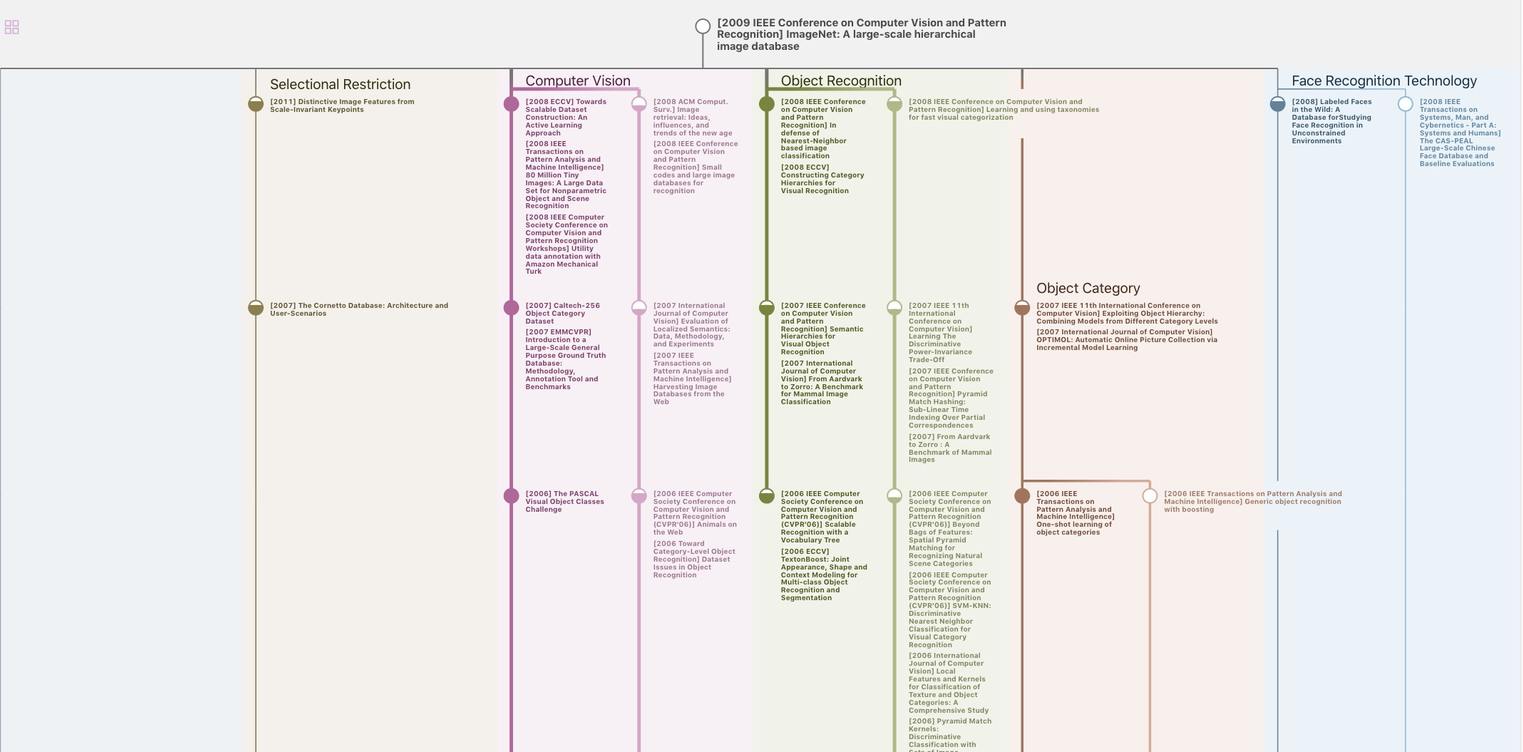
生成溯源树,研究论文发展脉络
Chat Paper
正在生成论文摘要