Trajectories: a framework for detecting temporal clinical event sequences from health data standardized to the Observational Medical Outcomes Partnership (OMOP) Common Data Model
JAMIA OPEN(2022)
摘要
Objective To develop a framework for identifying temporal clinical event trajectories from Observational Medical Outcomes Partnership-formatted observational healthcare data. Materials and Methods A 4-step framework based on significant temporal event pair detection is described and implemented as an open-source R package. It is used on a population-based Estonian dataset to first replicate a large Danish population-based study and second, to conduct a disease trajectory detection study for type 2 diabetes patients in the Estonian and Dutch databases as an example. Results As a proof of concept, we apply the methods in the Estonian database and provide a detailed breakdown of our findings. All Estonian population-based event pairs are shown. We compare the event pairs identified from Estonia to Danish and Dutch data and discuss the causes of the differences. The overlap in the results was only 2.4%, which highlights the need for running similar studies in different populations. Conclusions For the first time, there is a complete software package for detecting disease trajectories in health data. Lay Summary Modern principles for comorbidity studies that take time dimension into account and identify temporal disease trajectories from the data were published in 2014 by Jensen et al. However, the absolute number of such studies has remained small. We believe this is because these principles were not described sufficiently to allow exact replication and have led to variations in the methods of such studies.Based on the previous publications and best practices of that field, this article proposes a 4-step framework for clinical event trajectory studies and introduces an open-source R package that implements this approach. As a proof of concept, we apply the framework for replicating the largest published trajectory study (Danish population) on Estonian population-based data. In addition, we conducted a type 2 diabetes trajectory study on Estonian and Integrated Primary Care Information (IPCI) databases from the Netherlands. To the best of our knowledge, this is the first framework for assessing disease trajectories that can be applied to any data source standardized to the Observational Medical Outcomes Partnership Common Data Model.We show that the results are highly dependent on the dataset. By comparing the results from different datasets, we highlight the opportunities and challenges of these kinds of trajectory studies. These issues have not been thoroughly described before.
更多查看译文
关键词
OMOP,OHDSI,R package,observational data,trajectory
AI 理解论文
溯源树
样例
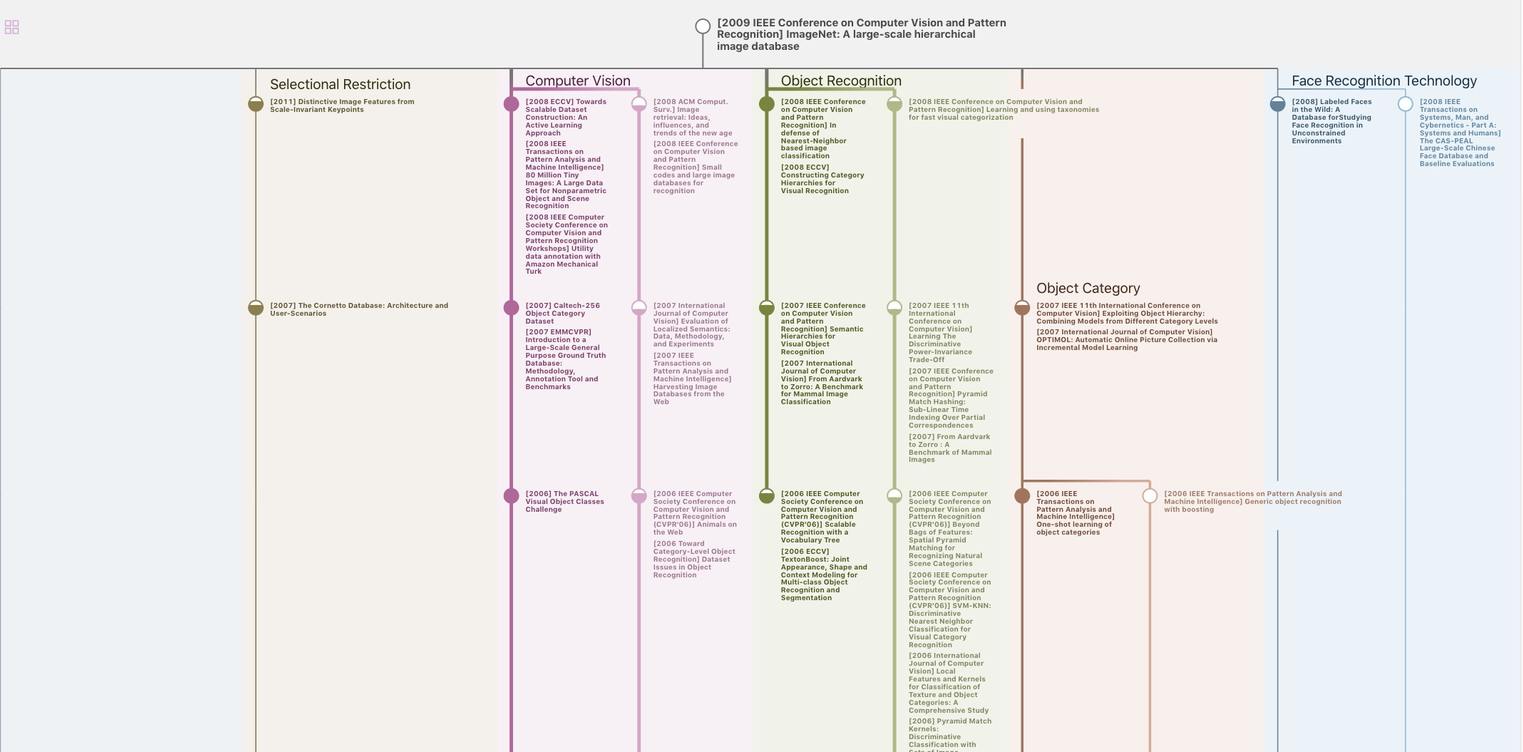
生成溯源树,研究论文发展脉络
Chat Paper
正在生成论文摘要