Projection-based techniques for high-dimensional optimal transport problems
WILEY INTERDISCIPLINARY REVIEWS-COMPUTATIONAL STATISTICS(2023)
摘要
Optimal transport (OT) methods seek a transformation map (or plan) between two probability measures, such that the transformation has the minimum transportation cost. Such a minimum transport cost, with a certain power transform, is called the Wasserstein distance. Recently, OT methods have drawn great attention in statistics, machine learning, and computer science, especially in deep generative neural networks. Despite its broad applications, the estimation of high-dimensional Wasserstein distances is a well-known challenging problem owing to the curse-of-dimensionality. There are some cutting-edge projection-based techniques that tackle high-dimensional OT problems. Three major approaches of such techniques are introduced, respectively, the slicing approach, the iterative projection approach, and the projection robust OT approach. Open challenges are discussed at the end of the review. This article is categorized under: Statistical and Graphical Methods of Data Analysis > Dimension Reduction Statistical Learning and Exploratory Methods of the Data Sciences > Manifold Learning
更多查看译文
关键词
curse of dimensionality,dimension reduction,optimal transport,Wasserstein distance
AI 理解论文
溯源树
样例
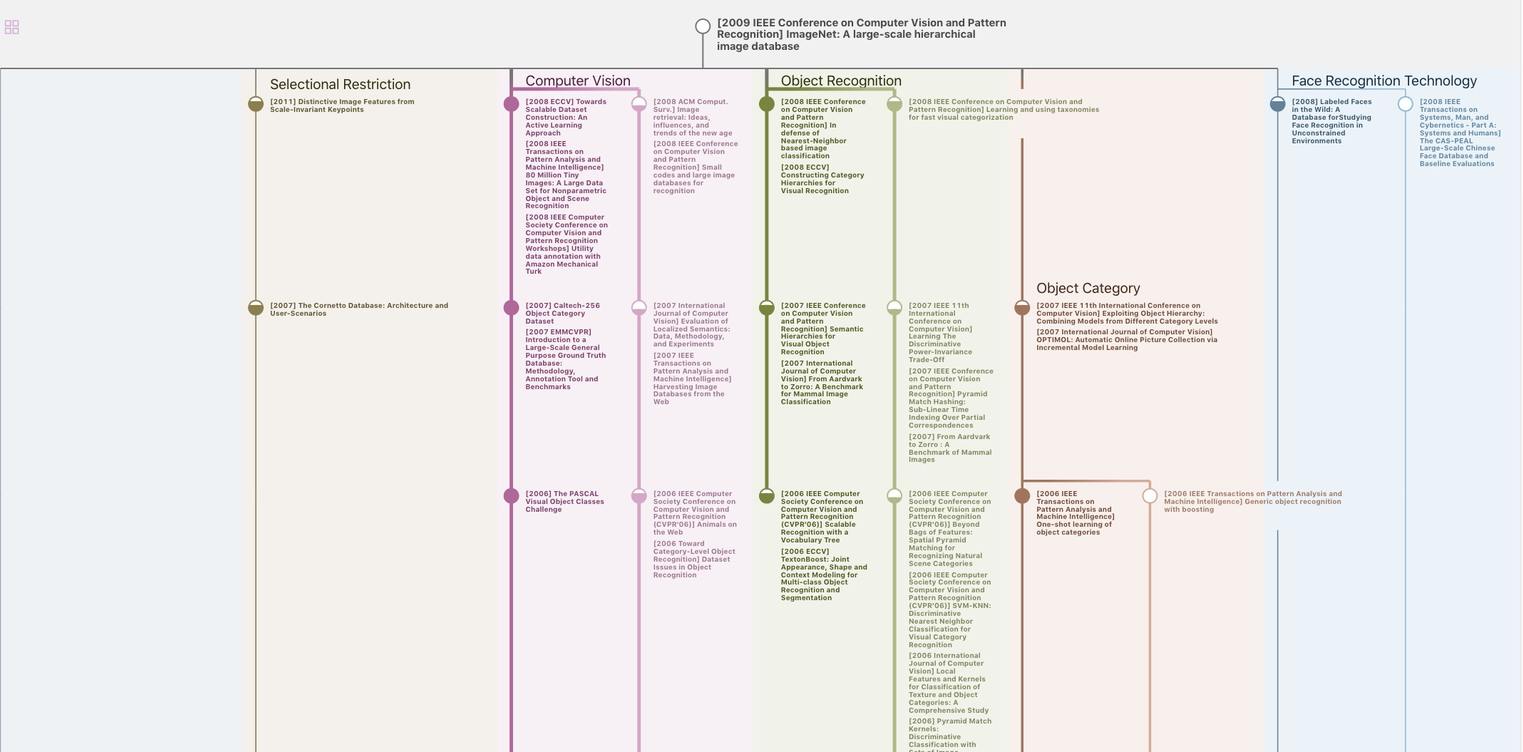
生成溯源树,研究论文发展脉络
Chat Paper
正在生成论文摘要