Robustness of predictive energy harvesting systems: Analysis and adaptive prediction scaling
IET COMPUTERS AND DIGITAL TECHNIQUES(2022)
摘要
Internet of Things (IoT) systems can rely on energy harvesting to extend battery lifetimes or even render batteries obsolete. Such systems employ an energy scheduler to optimise their behaviour and thus performance by adapting the system's operation. Predictive models of harvesting sources, which are inherently non-deterministic and consequently challenging to predict, are often necessary for the scheduler to optimise performance. Because the inaccurate predictions are utilised by the scheduler, the predictive model's accuracy inevitably impacts the scheduler and system performance. This fact has largely been overlooked in the vast amount of available results on energy schedulers and predictors for harvesting-based systems. The authors systematically describe the effect prediction errors have on the scheduler and thus system performance by defining a novel robustness metric. To alleviate the severe impact prediction errors can have on the system performance, the authors propose an adaptive prediction scaling method that learns from the local environment and system behaviour. The authors demonstrate the concept of robustness with datasets from both outdoor and indoor scenarios. In addition, the authors highlight the improvement and overhead of the proposed adaptive prediction scaling method for both scenarios. It improves a non-robust system's performance by up to 13.8 times in a real-world setting.
更多查看译文
关键词
adaptive systems, energy harvesting, internet of things, predictive scheduling, robustness
AI 理解论文
溯源树
样例
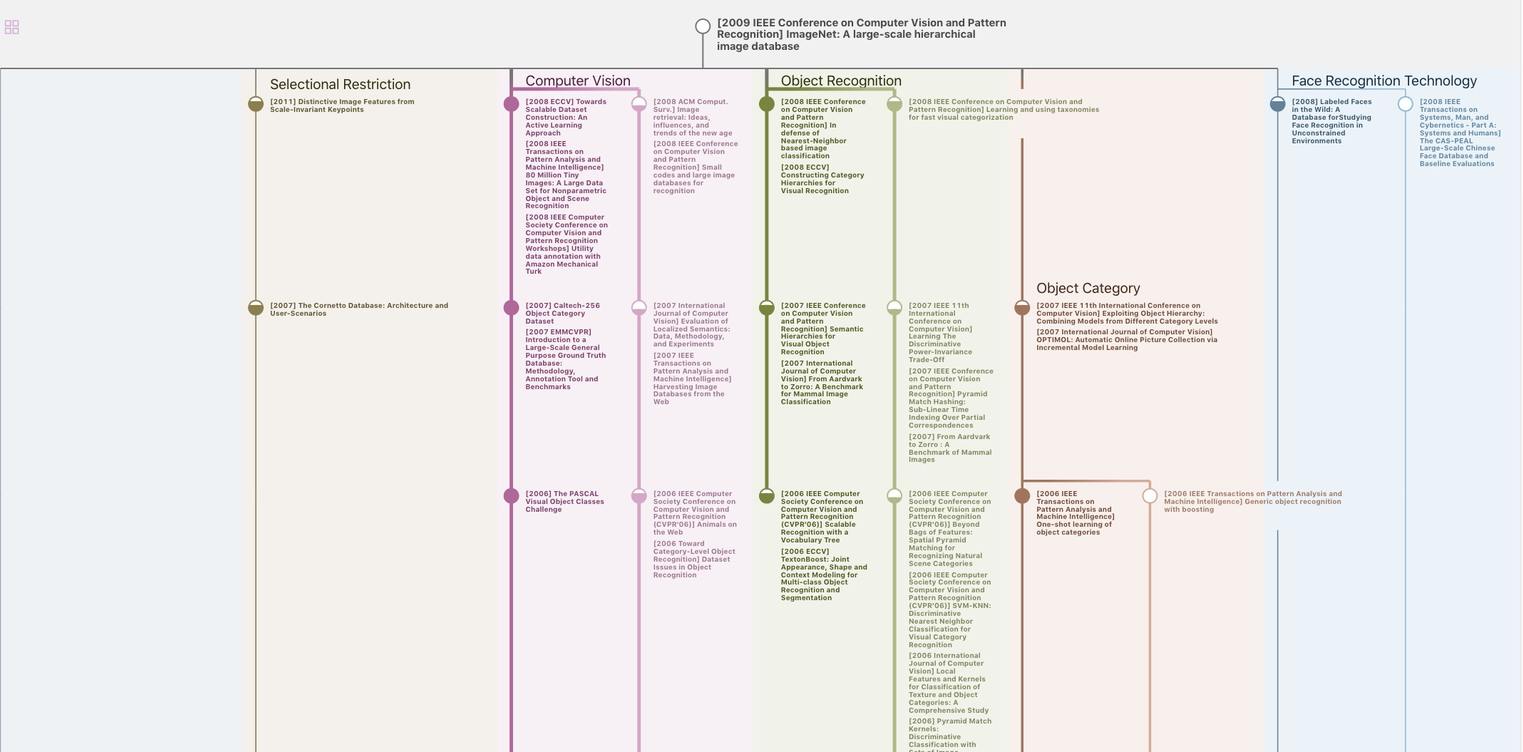
生成溯源树,研究论文发展脉络
Chat Paper
正在生成论文摘要