Whole constraint and partial triplet-center loss for infrared-visible re-identification
Neural Computing and Applications(2022)
摘要
In this paper, we propose a hybrid cross-dual-path feature learning network (HCDFL) to learn local discriminative characteristics and exploit a novel loss function called whole constraint loss and partial triplet-center loss (WCPTL) to narrow the discrepancy between cross-modality. HCDFL firstly extracts person features of two modalities, respectively, then horizontally slices the extracted features into p stripes and maps them into the shared space, finally utilizes modality-specific identity loss, cross-entropy and WCPTL proposed in this paper to improve the overall performance. Within WCPTL, whole constraint loss is utilized to narrow the discrepancy between two modalities, and partial triplet-center loss is utilized to ensure that samples from the same category can be close to the category center and away from other categories center. Experimental results demonstrate that the proposed method achieves better performance than other methods on two public datasets SYSU-MM01 and RegDB.
更多查看译文
关键词
Person re-identification, Infrared, Dual-path, Triplet loss, Center loss
AI 理解论文
溯源树
样例
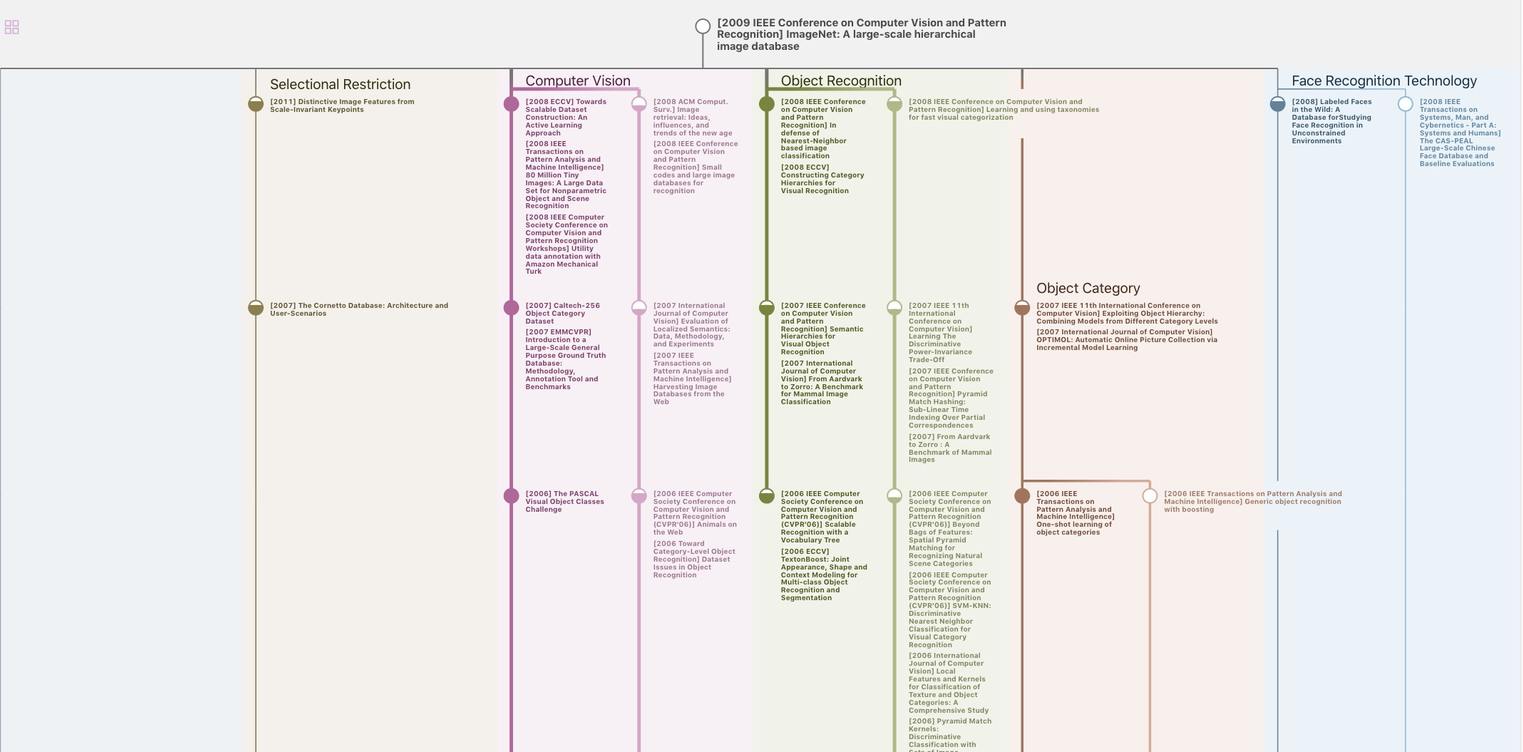
生成溯源树,研究论文发展脉络
Chat Paper
正在生成论文摘要