Secure Collaborative Environment for Seamless Sharing of Scientific Knowledge
DRIVING SCIENTIFIC AND ENGINEERING DISCOVERIES THROUGH THE INTEGRATION OF EXPERIMENT, BIG DATA, AND MODELING AND SIMULATION(2022)
摘要
In a secure collaborative environment, tera-bytes of data generated from powerful scientific instruments are used to train secure machine learning (ML) models on exascale computing systems, which are then securely shared with internal or external collaborators as cloud-based services. Devising such a secure platform is necessary for seamless scientific knowledge sharing without compromising individual, or institute-level, intellectual property and privacy details. By enabling new computing opportunities with sensitive data, we envision a secure collaborative environment that will play a significant role in accelerating scientific discovery. Several recent technological advancements have made it possible to realize these capabilities. In this paper, we present our efforts at ORNL toward developing a secure computation platform. We present a use case where scientific data generated from complex instruments, like those at the Spallation Neutron Source (SNS), are used to train a differential privacy enabled deep learning (DL) network on Summit, which is then hosted as a secure multi-party computation (MPC) service on ORNL's Compute and Data Environment for Science (CADES) cloud computing platform for third-party inference. In this feasibility study, we discuss the challenges involved, elaborate on leveraged technologies, analyze relevant performance results and present the future vision of our work to establish secure collaboration capabilities within and outside of ORNL.
更多查看译文
关键词
Differential privacy, Secure multi-party computation, Secure sharing of scientific knowledge
AI 理解论文
溯源树
样例
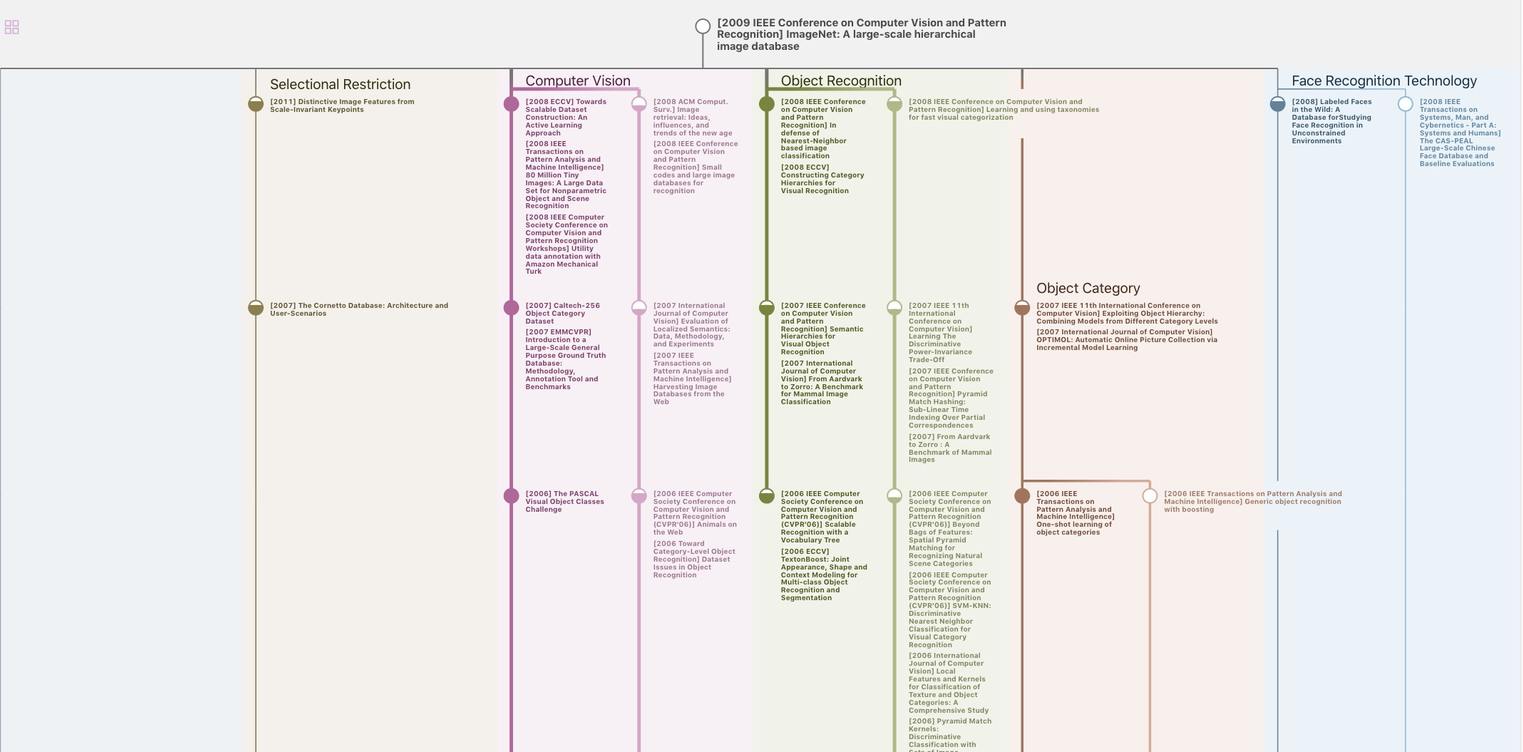
生成溯源树,研究论文发展脉络
Chat Paper
正在生成论文摘要