Machine Learning-Based Automated Selection of Regions for Analysis on Bone Marrow Aspirate Smears Abstracts
AMERICAN JOURNAL OF CLINICAL PATHOLOGY(2021)
摘要
Abstract Manual microscopic examination of bone marrow aspirate (BMA) smears and counting of cell populations remains the standard of practice for accurate assessment of benign and neoplastic bone marrow disorders. While automated cell classification software using machine learning models has been developed and applied to BMAs, current systems nonetheless require manual identification of optimal regions within the slide that are rich in marrow hematopoietic cells. To address this issue, we have developed a machine learning-based platform for automated identification of optimal regions in whole-slide images of BMA smears. A training dataset was developed by manual annotation of 53 BMA slides across biopsy diagnoses including unremarkable trilineal hematopoiesis, acute leukemia, and plasma cell neoplasms, as well as across differences in total cellularity represented by a spectrum of marrow nucleated cell content and white blood cell counts. 10,537 regions among these 53 slides were manually annotated as either “optimal” (regions near aspirate particles with high proportions of marrow nucleated cells), “particle” (aspirate particles), or “hemodilute” (blood-rich regions with high proportions of red blood cells). Training of a neural network-based classifier on 10x magnification slides with region cropping and image augmentation resulted in a classifier with substantial accuracy on new testing-set BMA slides (one-vs-rest AUROC > 0.999 across 10 training/testing splits for all 3 region classes), with very few particle and hemodilute regions being classified as optimal (particle: 0.83%, hemodilute: 0.39%). Additionally, this classifier accurately classifies BMA regions on slides from hematological disorders not represented in the training data, including Burkitt lymphoma (AUROC > 0.999 across region classes), chronic myeloid leukemia (AUROC > 0.999 across region classes), and diffuse large B-cell lymphoma (AUROC = 1 across region classes), demonstrating the broad applicability of our approach. To assess the performance of our classifier on whole-slide images, tiles from 10x magnification slides were manually annotated by three participants with notable concordance (Krippendorff’s alpha = 0.424); substantial agreement was found between manual annotations and model predictions within whole-slide images (optimal AUROC = 0.958, particle AUROC = 1.0, hemodilute AUROC = 0.947). Based on these promising results, this machine learning-based region classification model is being connected to a previously-developed bone marrow cell classifier to fully automate differential cell counting in whole-slide images. The development of this novel automated pipeline has potential to streamline the diagnostic process for hematological disorders while enhancing accuracy and replicability, as well as decreasing diagnostic turnaround time for improving patient care.
更多查看译文
AI 理解论文
溯源树
样例
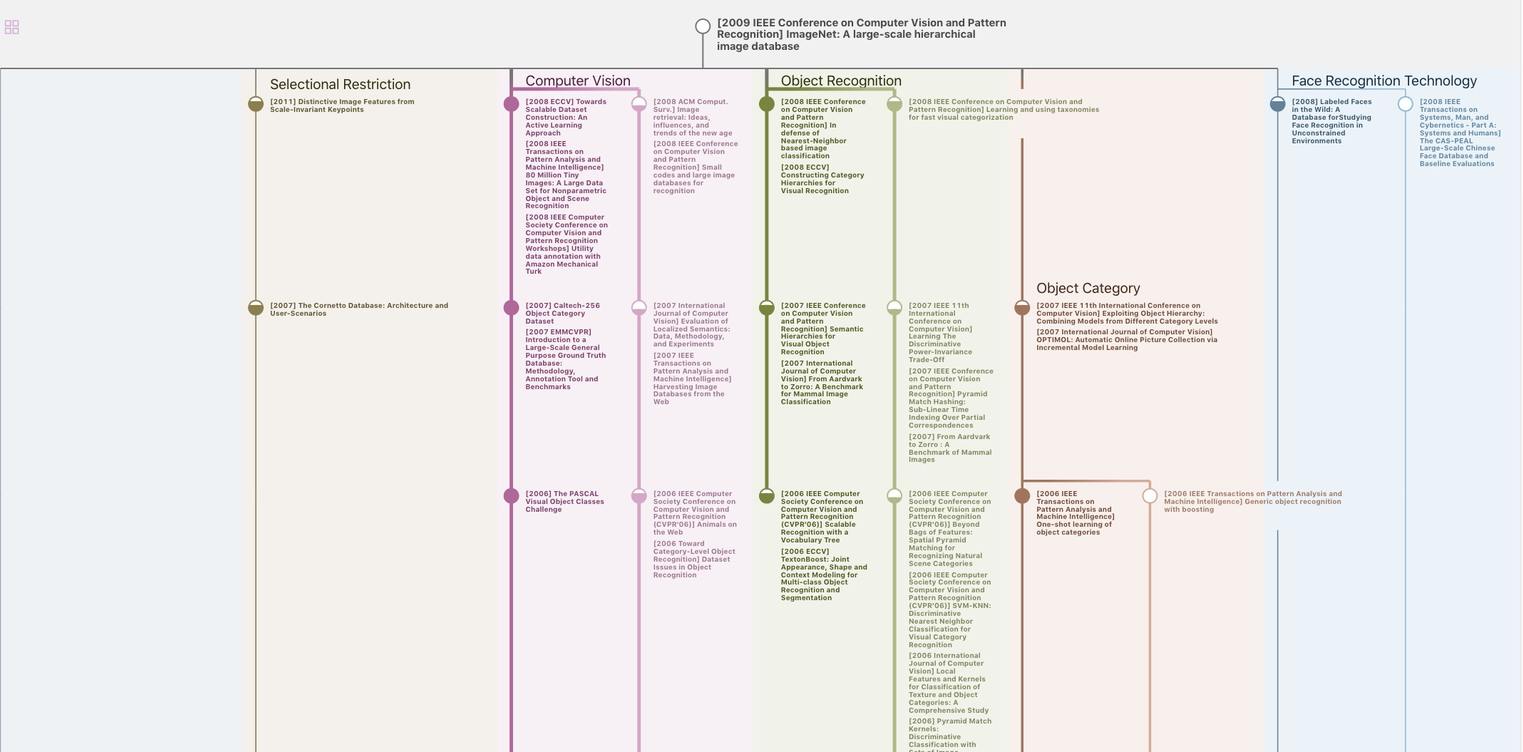
生成溯源树,研究论文发展脉络
Chat Paper
正在生成论文摘要