Using Deep Learning to Nowcast the Spatial Coverage of Convection from Himawari-8 Satellite Data
MONTHLY WEATHER REVIEW(2021)
摘要
Predicting the timing and location of thunderstorms ("convection'') allows for preventive actions that can save both lives and property. We have applied U-nets, a deep-learning-based type of neural network, to forecast convection on a grid at lead times up to 120 min. The goal is to make skillful forecasts with only present and past satellite data as predictors. Specifically, predictors are multispectral brightness-temperature images from the Himawari-8 satellite, while targets (ground truth) are provided by weather radars in Taiwan. U-nets are becoming popular in atmospheric science due to their advantages for gridded prediction. Furthermore, we use three novel approaches to advance U-nets in atmospheric science. First, we compare three architectures-vanilla, temporal, and U-net++-and find that vanilla U-nets are best for this task. Second, we train U-nets with the fractions skill score, which is spatially aware, as the loss function. Third, because we do not have adequate ground truth over the full Himawari-8 domain, we train the U-nets with small radar-centered patches, then apply trained U-nets to the full domain. Also, we find that the best predictions are given by U-nets trained with satellite data from multiple lag times, not only the present. We evaluate U-nets in detail-by time of day, month, and geographic location-and compare them to persistence models. The U-nets outperform persistence at lead times >= 60 min, and at all lead times the U-nets provide a more realistic climatology than persistence. Our code is available publicly.
更多查看译文
关键词
Deep convection, Satellite observations, Time series, Nowcasting, Deep learning, Neural networks
AI 理解论文
溯源树
样例
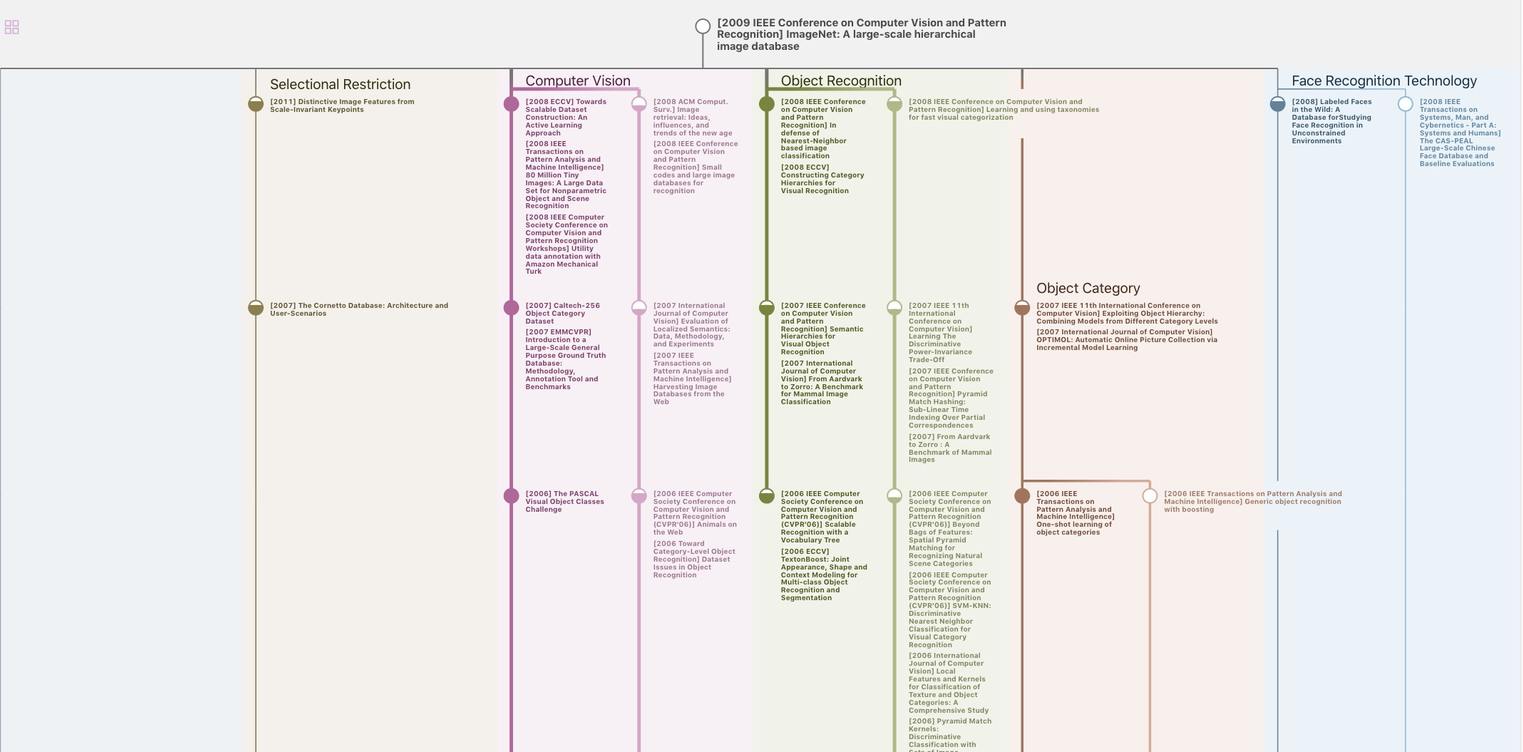
生成溯源树,研究论文发展脉络
Chat Paper
正在生成论文摘要