EEG based automatic multi-class classification of epileptic seizure types using recurrence plots
EXPERT SYSTEMS(2022)
摘要
There is an urgent need to develop an efficient system for accurate recognition of epileptic seizure type that could play a significant role in reducing the adversial effects of the disease. A lot of work is available for EEG based automatic seizure detection but very less attempts have been made towards the classification of variants of seizures. Moreover, none of the authors have included the EEG signals for myoclonic seizure type in their classification studies. Our study aims to propose the automatic machine learning based multi-class classification system for classifying six types of epileptic seizures (such as absence, focal non-specific, complex-partial, generalized, tonic-clonic and including myoclonic too) through the use of recurrence plots (RPs). In our study, we have collected 19-channel EEG data from a huge database of Temple University Hospital. Two classification modalities have been proposed depending upon the feature extraction approach followed for RPs- (a) Traditional approach using the recurrence quantification analysis (RQA) method; (b) Texture based approach using the hybrid method named as Unthresholded Recurrence Plot with Fractal Weighted Local Binary Pattern (URP-FWLBP), that has been proposed in our work using a combination of Unthresholded RPs (URPs) and Fractal Weighted Local Binary Pattern (FWLBP) method. Thereby, an indirect variant of support vector machine (one-vs-rest approach) has been used as the multi-class classifier in both the approaches. The performance of the proposed modalities has been validated using five-fold cross-validation method in the light of seven metrics- accuracy, sensitivity, specificity, Matthews correlation coefficient, geometric-mean, precision and f1-score. The experimental results show the effectiveness of the proposed system following hybrid URP-FWLBP method in performing multi-class epileptic seizure type classification with 100% efficiency, thereby outperforming the traditional RQA method based system as well the existing state-of-the-art systems.
更多查看译文
关键词
electroencephalogram,fractal weighted local binary pattern,multi-class seizure type classification,recurrence plots,recurrence quantification analysis,support vector machine
AI 理解论文
溯源树
样例
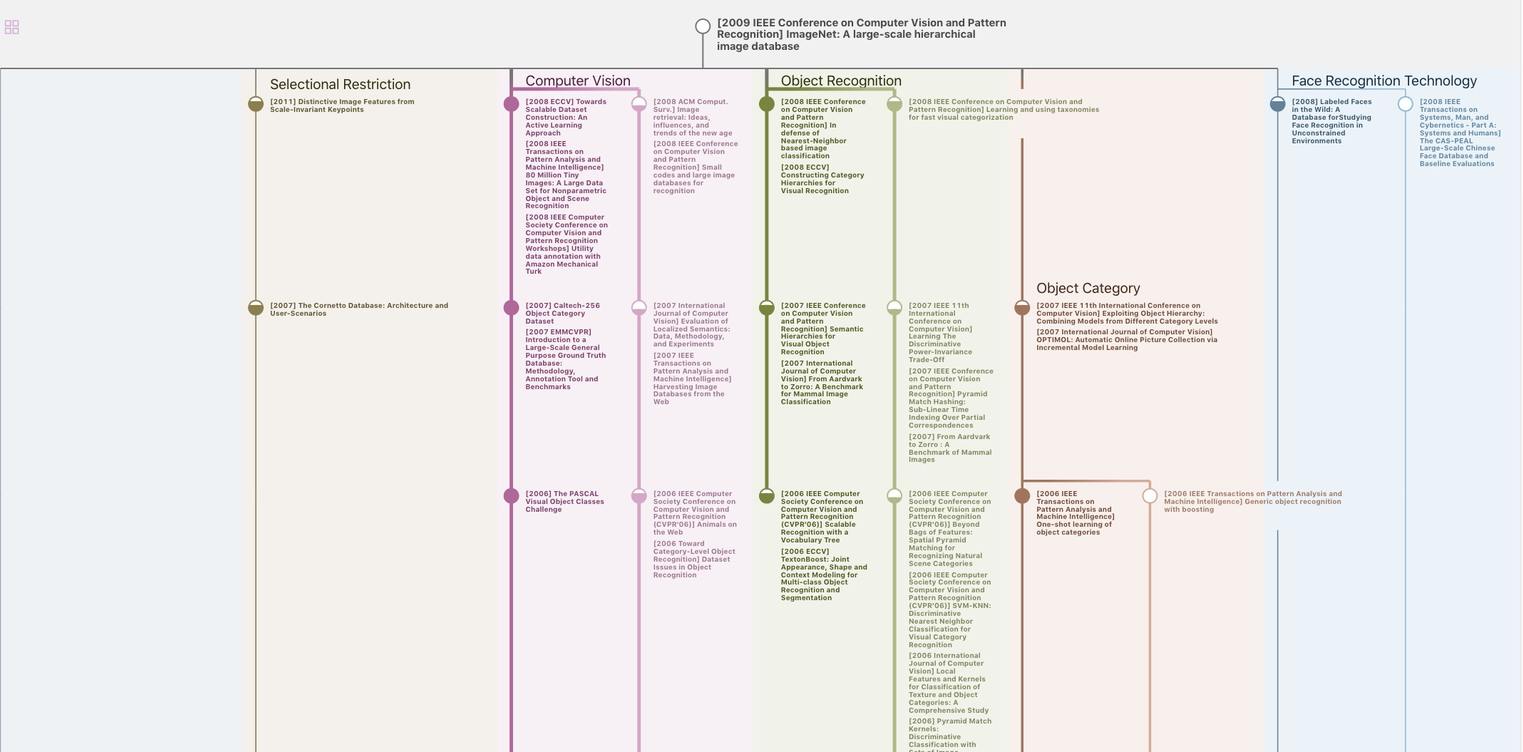
生成溯源树,研究论文发展脉络
Chat Paper
正在生成论文摘要