Unsupervised Adaptation of Deep Speech Activity Detection Models to Unseen Domains
APPLIED SCIENCES-BASEL(2022)
摘要
Speech Activity Detection (SAD) aims to accurately classify audio fragments containing human speech. Current state-of-the-art systems for the SAD task are mainly based on deep learning solutions. These applications usually show a significant drop in performance when test data are different from training data due to the domain shift observed. Furthermore, machine learning algorithms require large amounts of labelled data, which may be hard to obtain in real applications. Considering both ideas, in this paper we evaluate three unsupervised domain adaptation techniques applied to the SAD task. A baseline system is trained on a combination of data from different domains and then adapted to a new unseen domain, namely, data from Apollo space missions coming from the Fearless Steps Challenge. Experimental results demonstrate that domain adaptation techniques seeking to minimise the statistical distribution shift provide the most promising results. In particular, Deep CORAL method reports a 13% relative improvement in the original evaluation metric when compared to the unadapted baseline model. Further experiments show that the cascaded application of Deep CORAL and pseudo-labelling techniques can improve even more the results, yielding a significant 24% relative improvement in the evaluation metric when compared to the baseline system.
更多查看译文
关键词
Speech Activity Detection, unsupervised domain adaptation, Fearless Steps Challenge
AI 理解论文
溯源树
样例
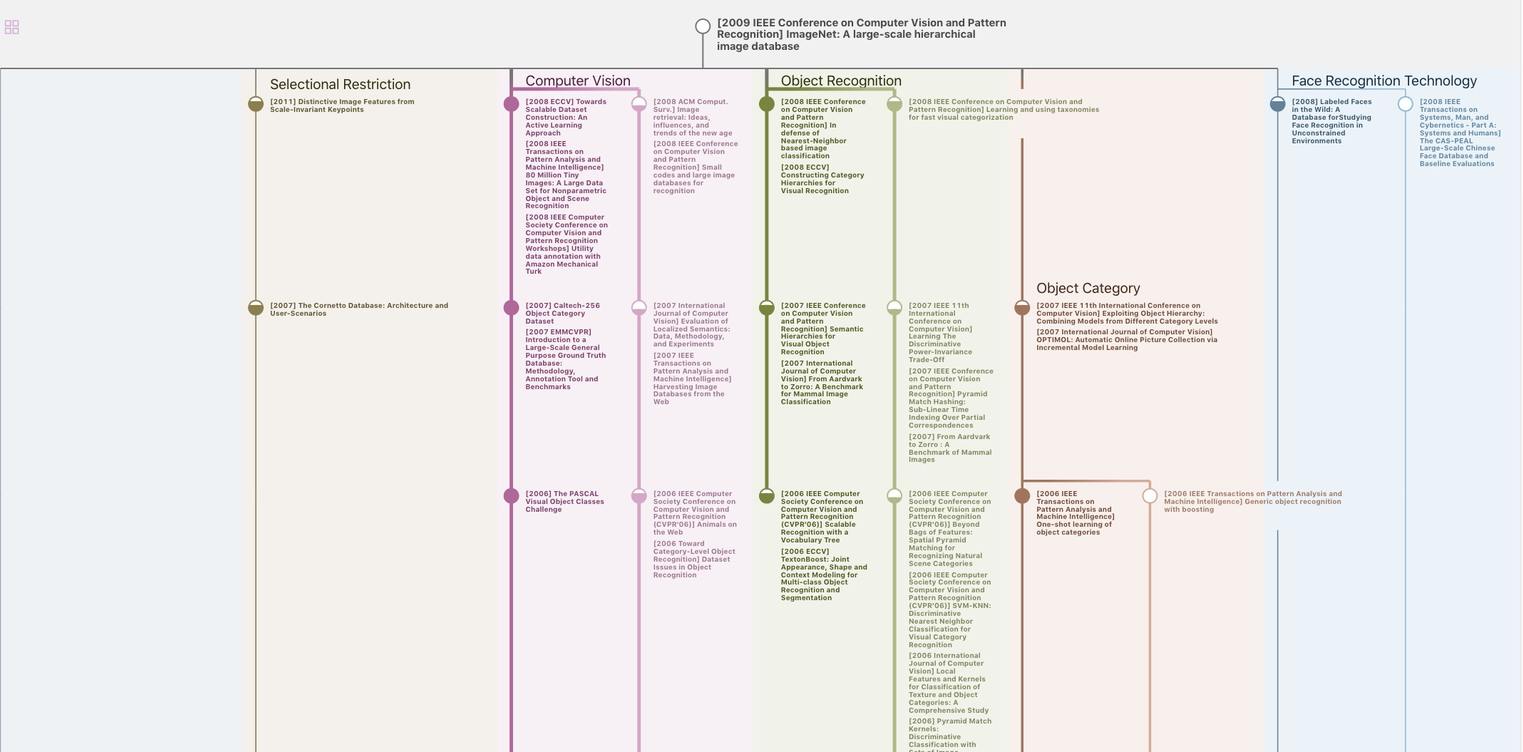
生成溯源树,研究论文发展脉络
Chat Paper
正在生成论文摘要