Real-world malicious event recognition in CCTV recording using Quasi-3D network
JOURNAL OF AMBIENT INTELLIGENCE AND HUMANIZED COMPUTING(2022)
摘要
Identification of exact malicious instant in lengthy CCTV recordings depends solely on Auto activity cognizance. The 3D CNN has previously been explored for the analysis of motion in video streams. Studies exhibit that, using separate filters for encoding spatial and temporal information has the same level of efficiency as that of 3D convolution filters. This study presents a novel approach through introduction of independent filters for event recognition in videos. This aims at learning extended Spatio-temporal features utilizing modified ResNet architecture. A novel 2D block termed as Quasi-3D (Q3D) decouples 3D information by combining 2D filters. The proposed Quasi-3D block encodes not only the spatial information in each frame but also the relative motion of objects along the x -axis and y -axis in a set of frames. Three variations of Quasi-3D block have been introduced to emphasize more on the features for further enhancing performance. A multi-class malicious activity recognition video dataset CrimesScene (drive:google:com/file/d/1omiQG9sxx375HjL97DqXxIX9nnfW3oQ/view?usp=sharing) inclusive of annotated video segments from 4 different classes of volume crimes has been developed. Results exhibit that the proposed Q3D ResNet model outperforms all other variants by achieving the overall detection accuracy of 94.9% and 93.07% on Hockey Fight and CrimesScene datasets, respectively.
更多查看译文
关键词
Spatio-temporal feature extraction,Scene recognition,Video analysis,Deep learning for videos
AI 理解论文
溯源树
样例
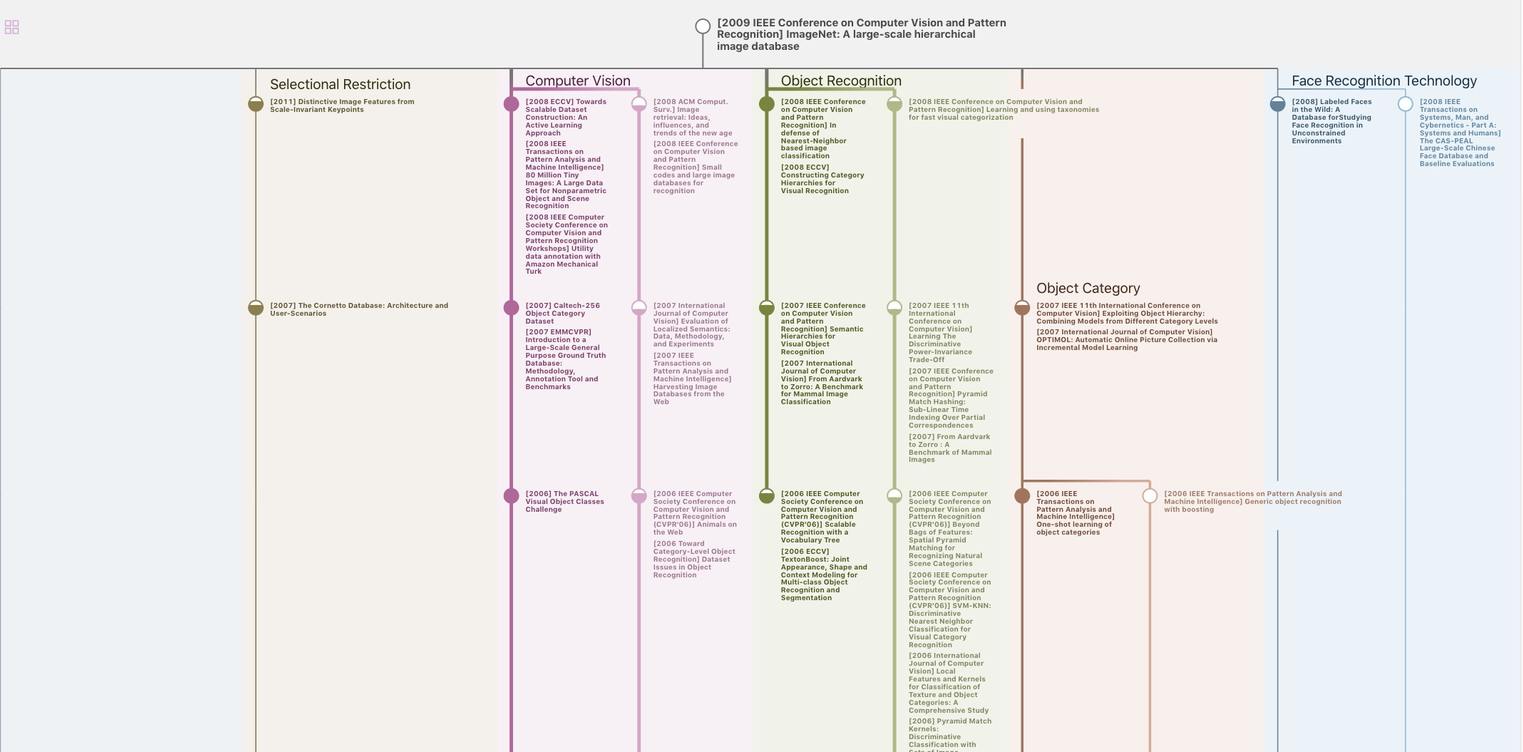
生成溯源树,研究论文发展脉络
Chat Paper
正在生成论文摘要