A New Handwritten Number Recognition Approach Using Typical Testors, Genetic Algorithms, and Neural Networks
SMART TECHNOLOGIES, SYSTEMS AND APPLICATIONS, SMARTTECH-IC 2021(2022)
摘要
In this paper, a method combining three techniques is proposed in order to reduce the amount of features used to train and predict over a handwritten data set of digits. The proposal uses typical testors and searches through evolutionary strategy to find a reduced set of features that preserves essential information of all the classes that compose the data set. Once found it, this reduced subset will be strengthened for classification. To achieve it, the neural network prediction accuracy plays the role of fitness function. Thus, when a subset reaches a threshold prediction accuracy, it is returned as a solution of this step. Evolutionary strategy makes this intense search of features viable in terms of computing complexity and time. The discriminator construction algorithm is proposed as a strategy to achieve a smaller feature subset that preserves the accuracy of the overall data set. The proposed method is tested using the public MNIST data set. The best result found a subset of 171 features out of the 784, which only represents 21.81% of the total number of characteristics. The accuracy average was 97.83% on the testing set. The results are also contrasted with the error rate of other reported classifiers, such as PCA, over the same data set.
更多查看译文
关键词
Evolutionary strategy, Fitness function, Genetic algorithms, Handwritten number classification, Multi-layer neural network, Typical testors
AI 理解论文
溯源树
样例
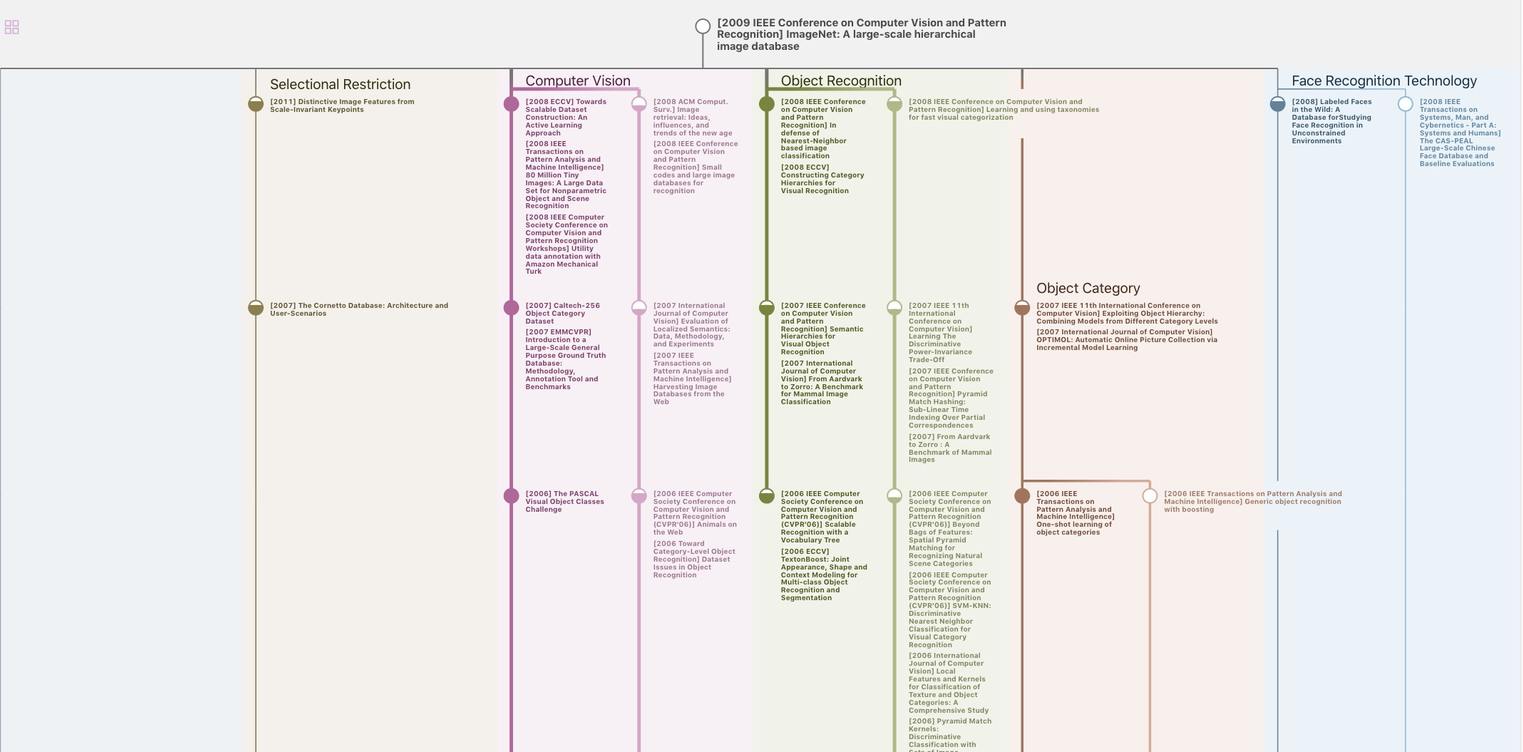
生成溯源树,研究论文发展脉络
Chat Paper
正在生成论文摘要