Feature Selection Pipeline based on Hybrid Optimization Approach with Aggregated Medical Data
INTERNATIONAL JOURNAL OF ADVANCED COMPUTER SCIENCE AND APPLICATIONS(2022)
摘要
For quite some time, the usage of many sources of data (data fusion) and the aggregation of that data have been underappreciated. For the purposes of this study, trials using several medical datasets were conducted, with the results serving as a single aggregated source for identifying eye illnesses. It is proposed in this paper that a diagnostic system that can detect diabetic retinopathy, glaucoma, and cataract can be built as an alternative to current methods. The data fusion and data aggregation techniques used to create this multi-model system made it conceivable. As the name implies, it is a way of compiling data from a large number of legitimate sources. The development of a pipeline of algorithms was accomplished through iterative trials and hyper parameter tweaking. CLARE (Contrast Level Adaptive Histogram Equalization) approaches, which increase the gradient between picture edges, improve segmentation by raising the contrast between picture edges. The Gabor filter has been shown to be the most effective method of selecting features. The Gabor filter was selected using a hybrid optimization method (LION + Cuckoo), which was developed by the author. For automation, the Support Vector Machine (SVM) radial is the most effective method since it delivers excellent stability and accuracy in terms of accuracy and recall, as well as precision and recall. The discoveries and approaches detailed here provide a more solid foundation for future image-based diagnostics researchers to build on in the future. Eventually, the findings of this study will help to improve healthcare workflows and practices.
更多查看译文
关键词
Content-based image retrieval system, CLAHE, Gabor filter, Cuckoo search, LION optimization, support vector machine
AI 理解论文
溯源树
样例
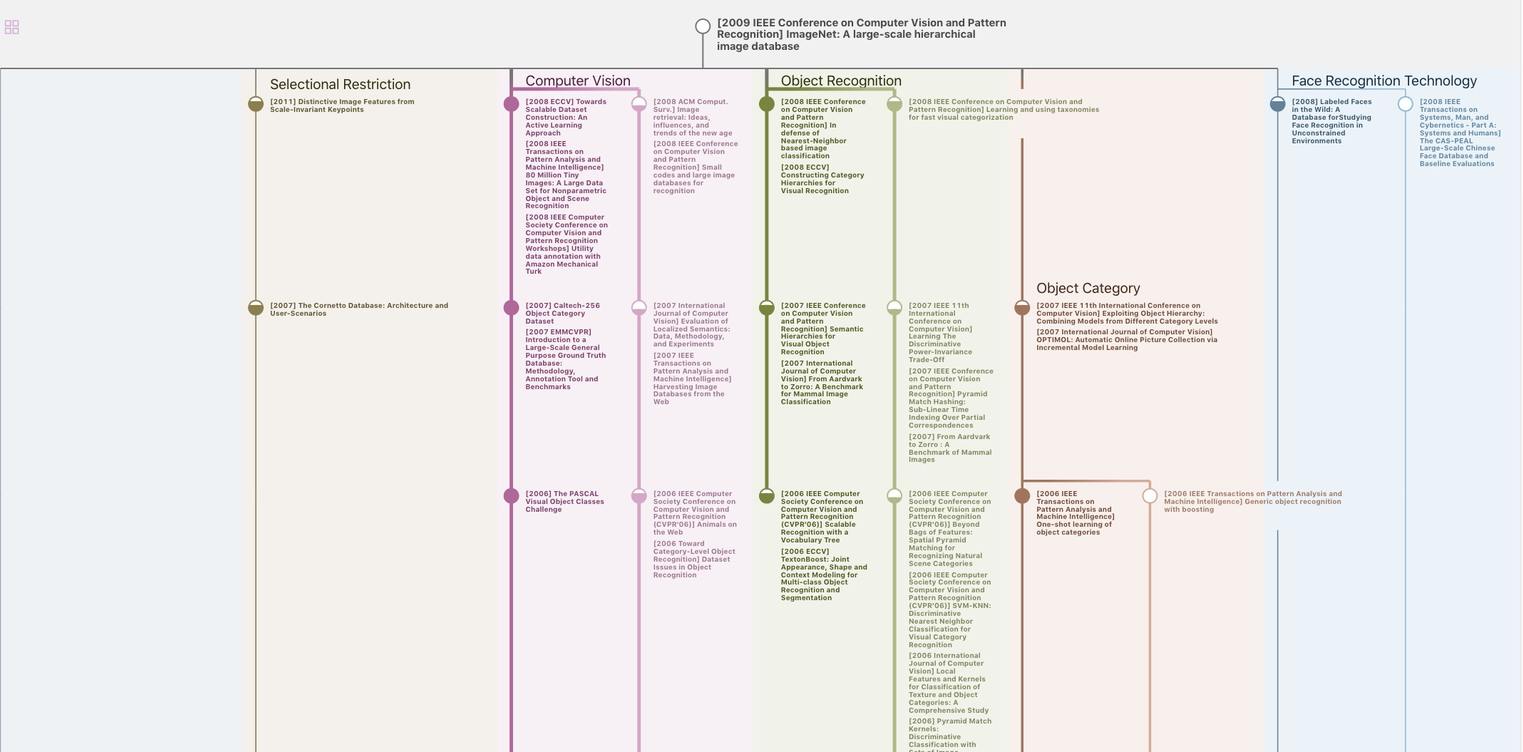
生成溯源树,研究论文发展脉络
Chat Paper
正在生成论文摘要