A deep learning automatic classification method for clogging pervious pavement
CONSTRUCTION AND BUILDING MATERIALS(2021)
摘要
Classification of clogging level of pervious pavement is important to the determination of pavement maintenance methods. Permeability test is the most commonly used method to determine clogging level of pervious pavement, but huge water consumption, stubborn sealant and huge workload restricts the efficiency and popularity of it. In this study, a deep learning automatic classification method for clogging pervious pavement was proposed. 1100 original photos were collected from three pervious pavements with a total length of 4500 m in Jinan. After preparatory work, a dataset containing 35,170 images, was established in this study. To select the best network, five models (Resnet-10, Resnet-12, Resnet-14, Resnet-16, and Resnet-18) based on the Convolutional Neural Networks (CNNs) were used for comparison. Classification accuracy, Macro-F1score, and F1score were used as the performance measurements for the study. Under the condition of four categories, Resnet-12 was the best model with 98.638% training set accuracy, 86.001% validation set accuracy, 84.047% Macro-F1score and 84.060% accuracy value. When the results were examined in terms of the binary classification based on maintenance methods, with 89.728% F1score and 89.476% accuracy score, Resnet-12 was still the best performing model. The experimental results supported that image recognition could be used as an alternative method for clogging level classification, overcome the shortcomings of traditional methods and accelerate the speed of classifying. This study provided a new idea of non-destructive automatic classification method for clogging pervious pavement and a powerful tool for the determination of pervious pavement maintenance methods.
更多查看译文
关键词
Deep learning, Convolutional Neural Networks, Pervious pavement, Clogging levels classification
AI 理解论文
溯源树
样例
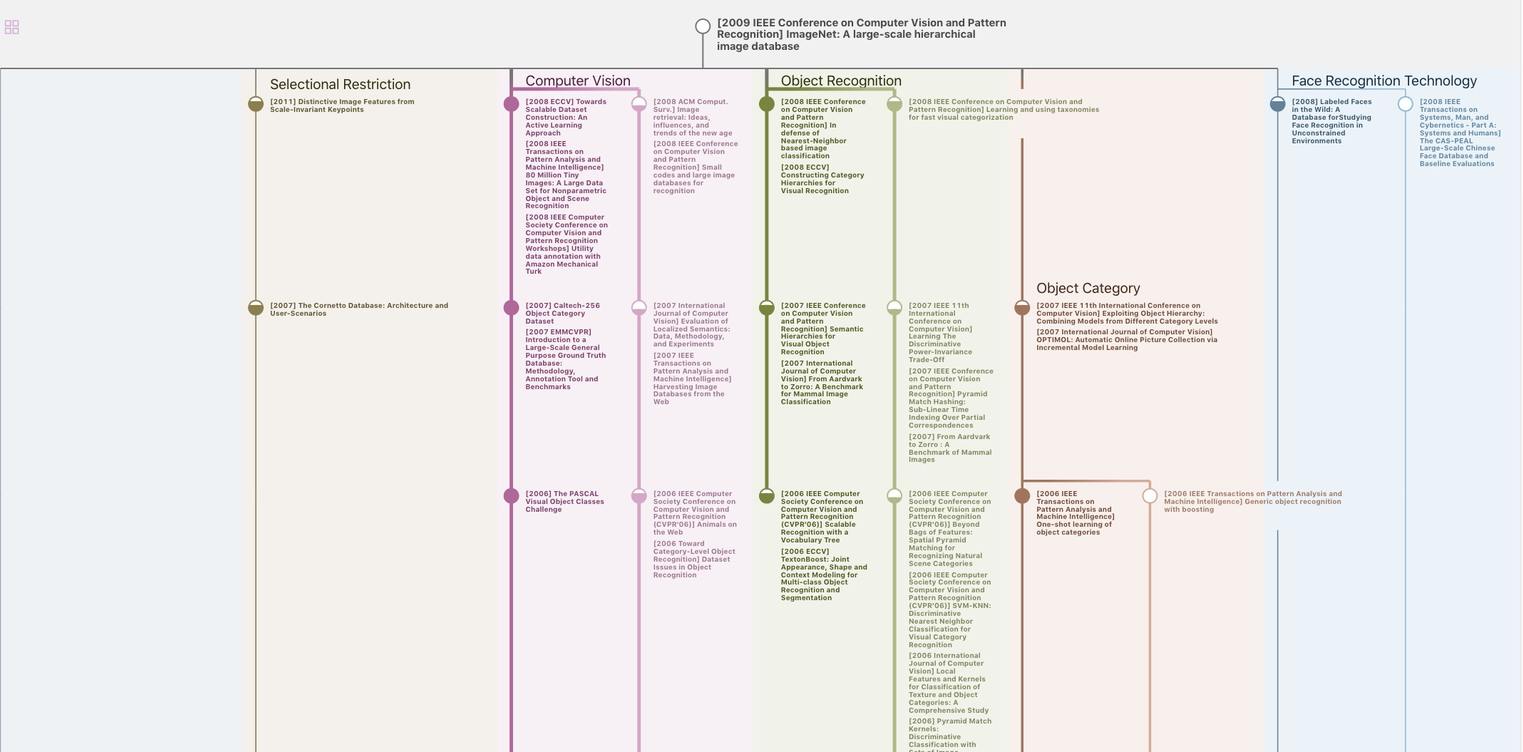
生成溯源树,研究论文发展脉络
Chat Paper
正在生成论文摘要