Balance learning for ship detection from synthetic aperture radar remote sensing imagery
ISPRS Journal of Photogrammetry and Remote Sensing(2021)
摘要
Synthetic aperture radar (SAR) is playing an important role in maritime domain awareness. As a fundamental ocean mission, SAR ship detection can offer high-quality services for marine surveillance. It receives widespread concern in the SAR remote sensing community. Recently, deep learning (DL) has greatly improved SAR ship detection accuracy. However, there are still some unperceived imbalance problems that seriously hinder further accuracy improvements among current DL-based SAR ship detectors. Therefore, we propose a novel concept of balance learning (BL) for high-quality SAR ship detection. We first point out the four unperceived imbalance problems, i.e., image sample scene imbalance, positive negative sample imbalance, ship scale feature imbalance, and classification regression task imbalance. Then, we offer some profound insights into these imbalances. Immediately, we propose four effective solutions to handle the above four imbalances correspondingly, i.e., balance scene learning mechanism (BSLM), balance interval sampling mechanism (BISM), balance feature pyramid network (BFPN), and balance classification regression network (BCRN). Finally, combined with the four solutions, a novel balance learning network (BL-Net) is proposed. Ablation studies can confirm each solution’s effectiveness. Experimental results on five open datasets (SSDD, Gaofen-SSDD, Sentinel-SSDD, SAR-Ship-Dataset, and HRSID) reveal BL-Net’s state-of-the-art SAR ship detection performance compared to the other 30 DL-based SAR ship detectors. Specifically, in contrast to the current most competitive method, the accuracy increase of BL-Net is 2.98% on SSDD, 1.97% on Gaofen-SSDD, 1.49% on Sentinel-SSDD, 0.55% on SAR-Ship-Dataset, and 4.95% on HRSID. Last but not least, the satisfactory ship detection results on another two large-scene Sentinal-1 SAR images confirm BL-Net’s strong migration capability. This indicates BL-Net’s potential value in marine surveillance.
更多查看译文
关键词
Synthetic aperture radar (SAR),Ship detection,Marine surveillance,Imbalance problems and solutions,Balance learning (BL),Balance learning network (BL-Net)
AI 理解论文
溯源树
样例
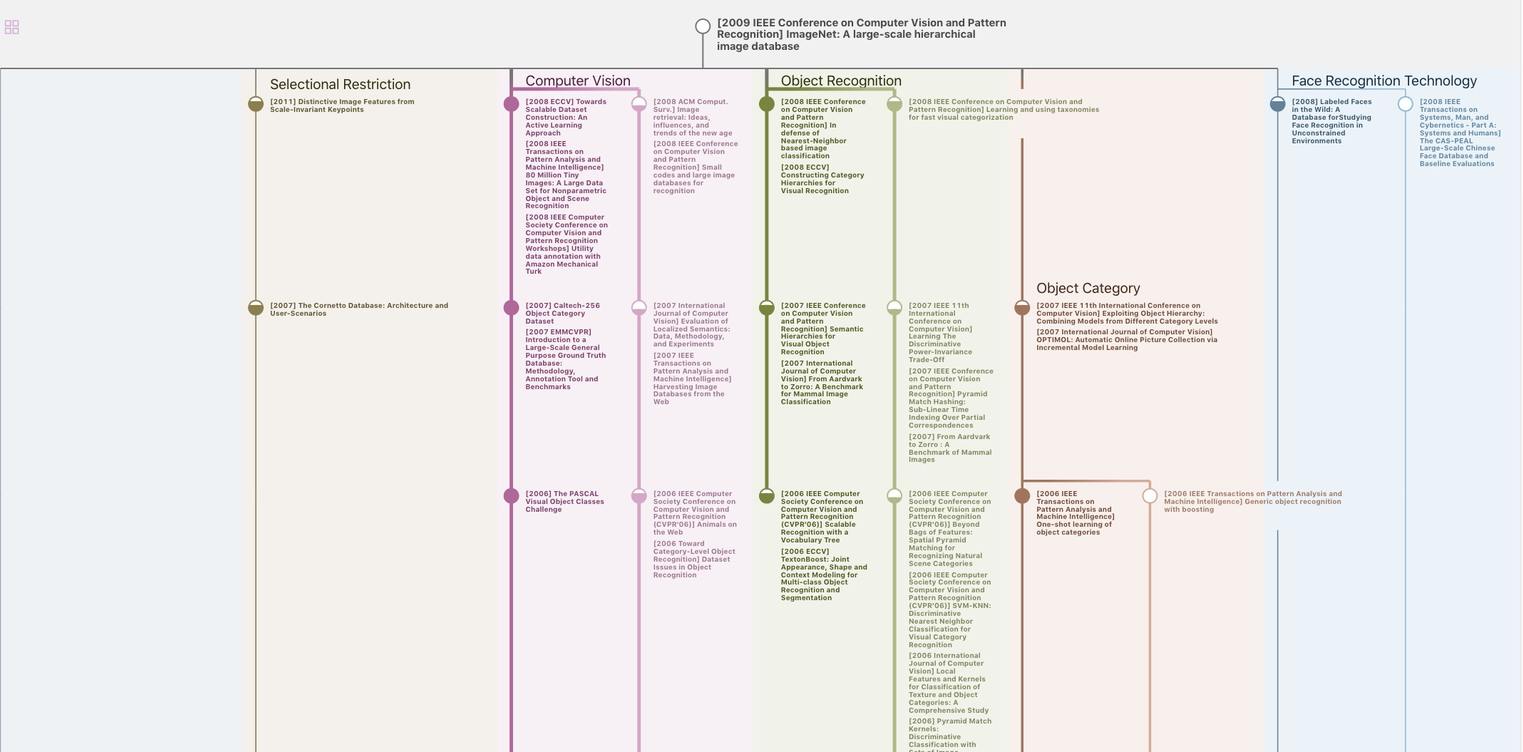
生成溯源树,研究论文发展脉络
Chat Paper
正在生成论文摘要