Groundwater Remediation Design Underpinned By Coupling Evolution Algorithm With Deep Belief Network Surrogate
Water Resources Management(2022)
摘要
Groundwater remediation design is crucial to contemporary water resources management, which is prone to massive computational costs due to the complexity and nonlinearity of the groundwater system. Traditional surrogate methods that can reduce the computational costs tend to encounter barriers of scalability and accuracy when the input–output relationship is highly nonlinear or high-dimensional. To tackle these problems, we herein propose a novel simulation–optimization method that embeds the deep learning deep belief network (DBN) into the particle swarm optimization (PSO) algorithm for groundwater remediation design. Firstly, a numerical simulation model based on MODFLOW and MT3DMS is established to describe the impact on the pollution environmental fate of various implementations of the remediation strategy. The input dataset to train DBN is comprised of various remediation strategies that evolve automatically in the PSO iterations, and the corresponding output dataset constituted of contaminant concentration at observation wells is garnered by executing the simulation model. In the optimization process, the DBN is retrained in an adaptive pattern to enhance prediction accuracy, selectively substituting for the original simulation model to alleviate the computational burden. Additionally, the PSO algorithm undergoes discretization and collision averting within each individual to adapt to the specific remediation task. The results reveal that the proposed method manifests satisfactory convergence behaviour and accuracy, capable of unburdening a considerable proportion (68.8%) of the time consumption for optimal groundwater remediation design.
更多查看译文
关键词
Surrogate model, Deep belief network, Groundwater remediation, Deep learning, PSO algorithm
AI 理解论文
溯源树
样例
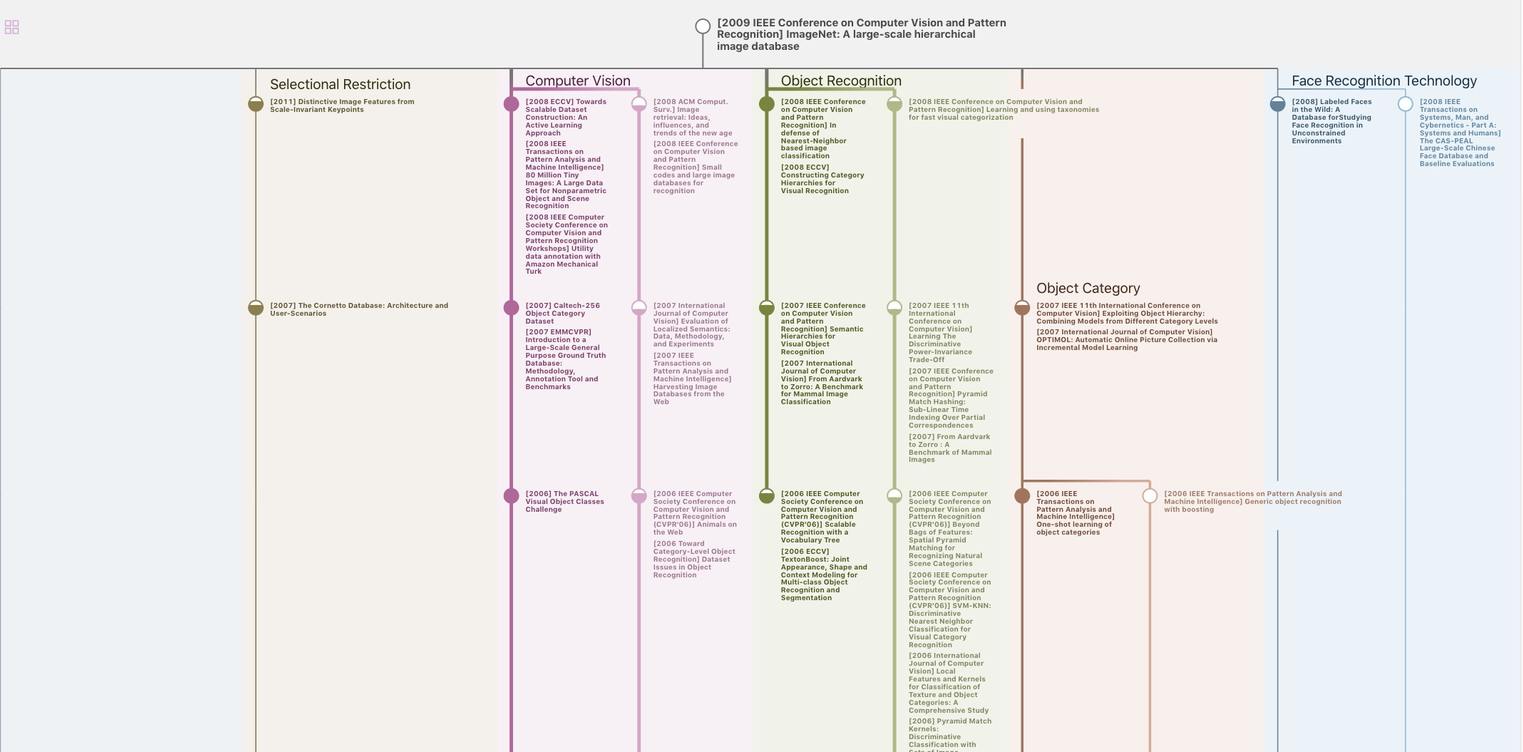
生成溯源树,研究论文发展脉络
Chat Paper
正在生成论文摘要