Rail Steel Health Analysis Based on a Novel Genetic Density-based Clustering Technique and Manifold Representation of Acoustic Emission Signals
APPLIED ARTIFICIAL INTELLIGENCE(2022)
摘要
In order to improve the effectiveness of the acoustic emission (AE) technique in rail health monitoring, a novel genetic clustering technique is proposed to categorize data automatically, integrating density-based clustering and t-distributed stochastic neighbor embedding. A primary problem in optimizing density-based clustering is to accommodate noise, for it explicitly computes the noise subset. Thus, the generalized silhouette index is proposed as a profitable objective to properly tackle noise and arbitrary shapes. The proposed method is initially testified in ten benchmark datasets, which manifests a superiority in handling irregular shape datasets and noise interference. Furthermore, the proposed method is applied in real-world AE signals acquired from tensile tests. The clustering results elucidated that it outperforms the comparative methods in categorizing the fused AE features and remains robust with increasing railway noise interference. In conclusion, the proposed method is validated to discover intrinsic groups of AE data and analyze potential rail health stages.
更多查看译文
AI 理解论文
溯源树
样例
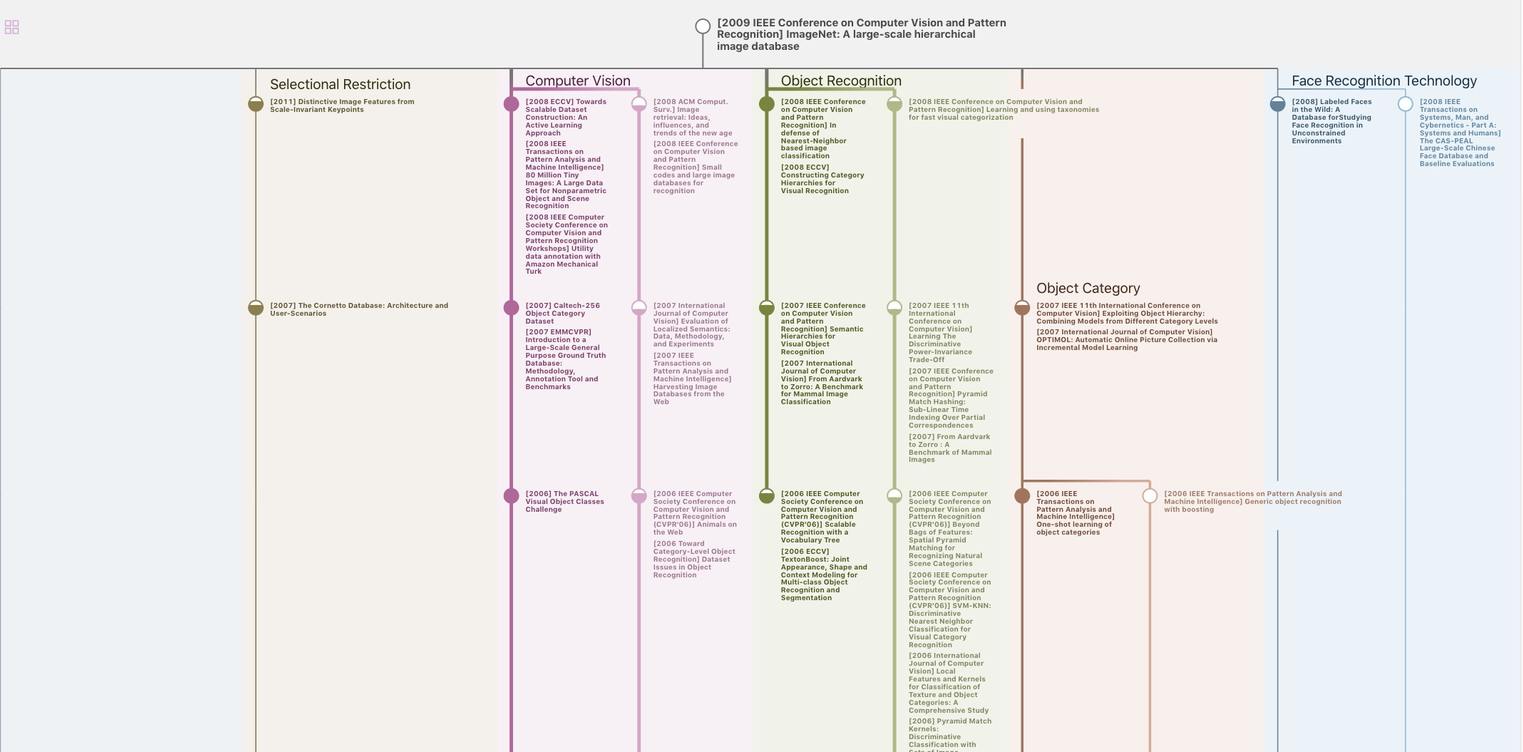
生成溯源树,研究论文发展脉络
Chat Paper
正在生成论文摘要