A Task Offloading Algorithm With Cloud Edge Jointly Load Balance Optimization Based on Deep Reinforcement Learning for Unmanned Surface Vehicles
IEEE ACCESS(2022)
摘要
Unmanned Surface Vehicles (USVs) generate a large amount of data that needs to be processed in real time when they work, but they are usually limited by computational and battery resources, so they need to offload their tasks to the edge for processing. However, when numerous USVs offload their tasks to the edge nodes, some offloaded tasks may be thrown due to queuing timeouts. Existing task offloading methods generally consider the latency or the overall system energy consumption caused by the collaborative processing at the edge and end layers, and do not consider the wasted energy when the tasks are thrown. Therefore, to address the above situation, this paper establishes a task offloading model to minimize long-term task latency and energy consumption by jointly considering the requirments of latency and energy-sensitive tasks and the overall load dynamics in the cloud, edge, and end layers. A deep reinforcement learning (DRL)-based Task Offloading with Cloud Edge Jointly Load Balance Optimization algorithm (TOLBO) is proposed to select the best edge server or cloud server for offloading. Simulation results show that the algorithm can improve the utilization of energy consumption of the cloud edge nodes compared with other algorithms. At the same time, it can significantly reduce the task throw rate, average latency, and energy consumption of end devices.
更多查看译文
关键词
Task analysis, Servers, Energy consumption, Computational modeling, Delays, Optimization, Cloud computing, USV, mobile edge computing, offloading delay, energy consumption, DRL
AI 理解论文
溯源树
样例
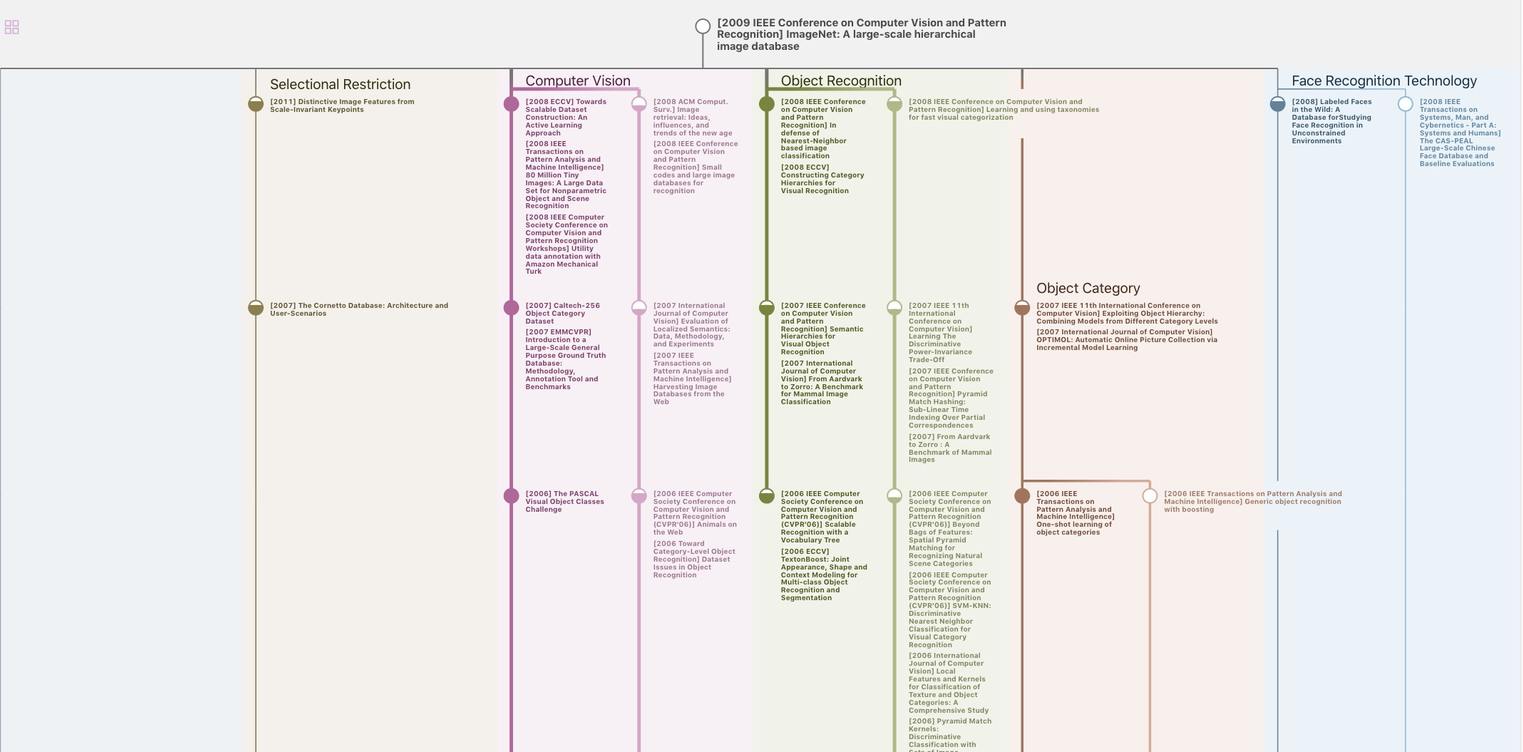
生成溯源树,研究论文发展脉络
Chat Paper
正在生成论文摘要