Granular-Based Multilayer Spatiotemporal Network With Control Gates for Energy Prediction of Steel Industry
IEEE TRANSACTIONS ON INSTRUMENTATION AND MEASUREMENT(2021)
摘要
Spatiotemporal analysis has drawn a lot of attentions recently on industrial time series prediction. Most of the existing methods cannot consider the production semantics or recognize the time-order characteristic of different processes in spatial dimension. In this study, a novel multilayer spatiotemporal network based on granular modeling and long short-term memory (LSTM) network is proposed. Regarding the energy data that contain semantic characteristics, a number of information granules are first partitioned based on the process or phase semantics. For representing the spatiotemporal dependencies among the granular sequences, a spatiotemporal topology network is established, where the units are defined specifically by their spatial layers located. To reduce the network complexity, a new gating mechanism is further put forward within the units, with which the network can understand the time-order characteristics of spatial granules at each time step and adjust the impact of input data on the updating procedure accordingly. The performance of the proposed method is validated on the benchmark dataset and the practical energy data with different fluctuation characteristics, using the state-of-the-art comparative algorithms. The results indicate that the proposed method can recognize the spatiotemporal relationship effectively and outperform the comparative ones on prediction accuracy.
更多查看译文
关键词
Energy prediction, granular computing, long short-term memory (LSTM), spatiotemporal series analysis, steel industry
AI 理解论文
溯源树
样例
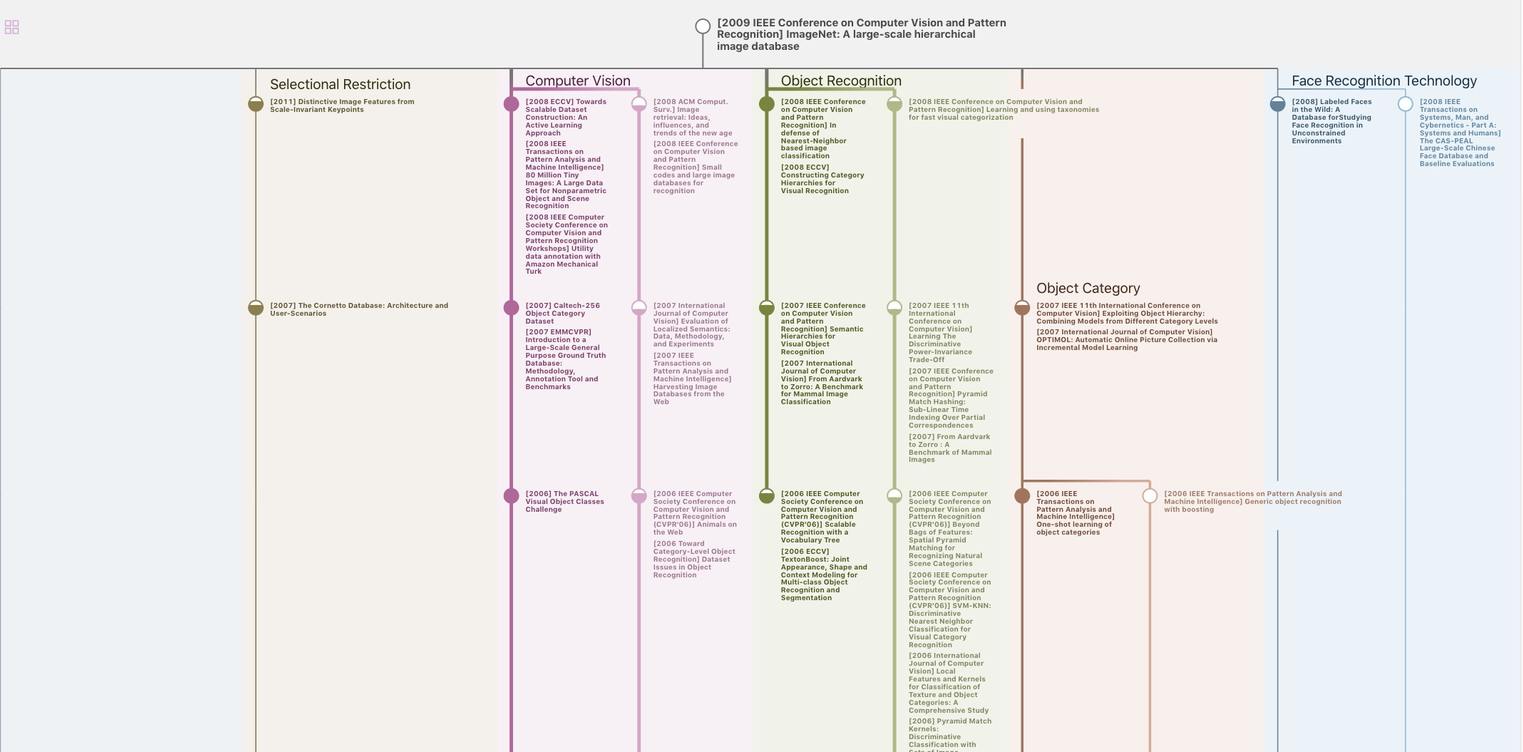
生成溯源树,研究论文发展脉络
Chat Paper
正在生成论文摘要