Popularity Prediction for Consumers' Product Recommendation Articles
JOURNAL OF GLOBAL INFORMATION MANAGEMENT(2022)
摘要
Consumer' product recommendation articles posted in social shopping communities (SSC) have become an important source of purchase information for other potential consumers. owever, less effort has been put into understanding and predicting the popularity of such a distinctive form of consumer-generated content. In this study, the authors built a rich and comprehensive dataset comprising author-related features, article-related features, and engagement behavior information collected from post. smzdm.com. They constructed machine learning models to predict article popularity and used the Shapley additive explanations (SHAP) approach to visualize and explain feature importance in the prediction. The results show that with all identified features, LGBMClassifier gives best results with most evaluation metrics, and that the author-related feature set has better predictive capability than the article-related one. To the best of the authors' knowledge, this is the first study to investigate the popularity of consumers' product recommendation articles in SSCs.
更多查看译文
关键词
Engagement Behavior, Feature Importance, Machine Learning, Popularity Prediction, Product Recommendation, Social Shopping Community
AI 理解论文
溯源树
样例
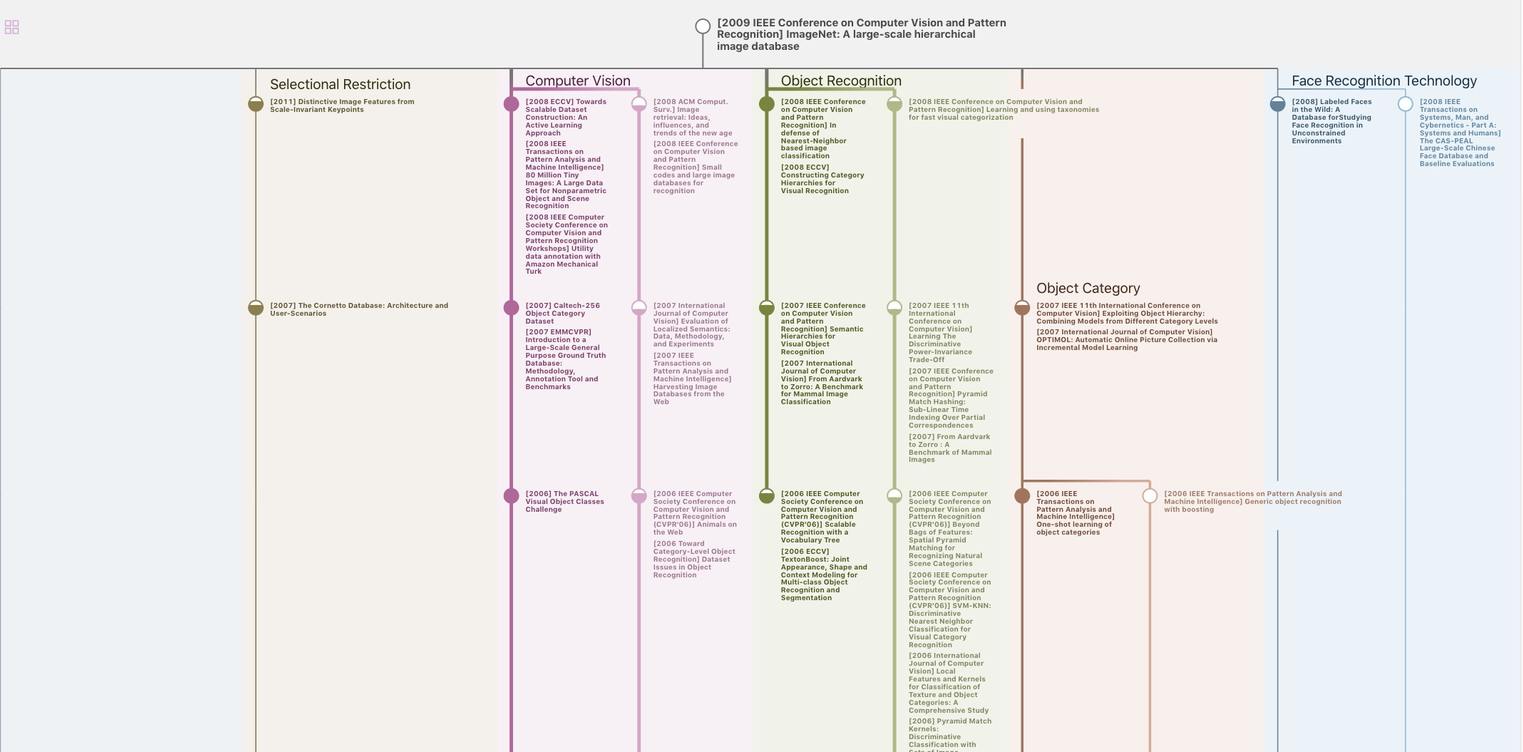
生成溯源树,研究论文发展脉络
Chat Paper
正在生成论文摘要