Unsupervised dam anomaly detection with spatial-temporal variational autoencoder
STRUCTURAL HEALTH MONITORING-AN INTERNATIONAL JOURNAL(2023)
摘要
The anomaly detection and health monitoring of dams have attracted increasing attention. To detect the temporal and spatial anomalies of the dam, a novel spatial-temporal variational autoencoder is proposed. The proposed model is based on the sequential variational autoencoder, and its backbone is fulfilled by the recurrent neural network and graph convolutional network to capture the temporal and spatial features in both the generative and inference models. To obtain a normal pattern, we made an assumption that the normal values should be temporally smooth and spatially similar. Then, the smoothness and similarity-inducing operations are used in the framework of the proposed model. Through the addition of smoothness and similarity losses in sequential variational autoencoder, the proposed model can obtain a temporally smooth and spatially similar pattern. For verification, an arch dam is taken as an example. Through comparison among six baseline models, the proposed model detects the temporal and spatial anomalies accurately and stably.
更多查看译文
关键词
dam engineering, sequential variational autoencoder, unsupervised anomaly detection, multivariate times series
AI 理解论文
溯源树
样例
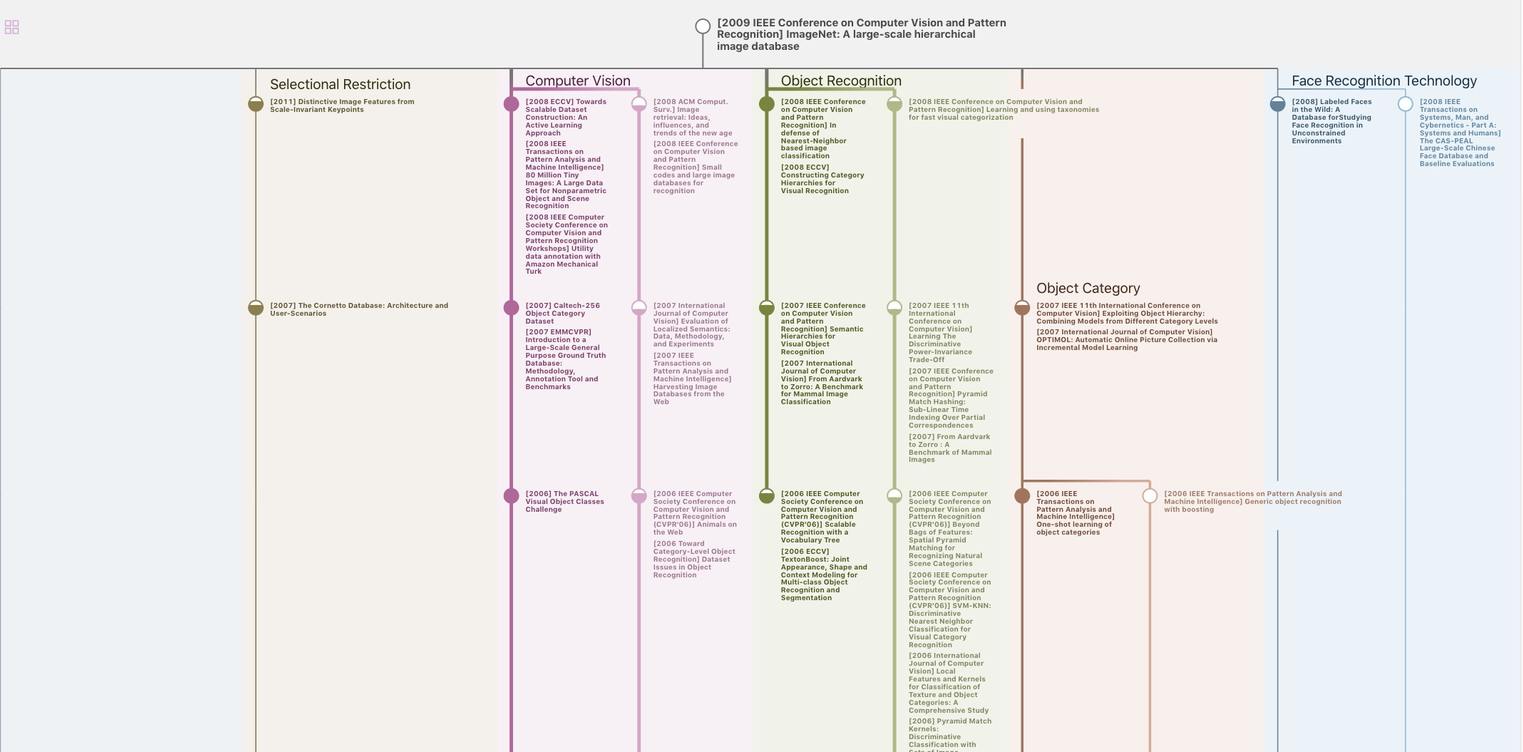
生成溯源树,研究论文发展脉络
Chat Paper
正在生成论文摘要