Sentiment-based masked language modeling for improving sentence-level valence–arousal prediction
Applied Intelligence(2022)
摘要
Sentiment indicator prediction is a crucial task in sentiment analysis or emotion recognition. Through the accurate quantification of sentiments expressed in a text, people’s sentiments can be better understood. Many studies have used mask language modeling to predict sentiment indicators. In this approach, every word has the same masking probability irrespective of its degree of influence on sentiments because a random masking mechanism is adopted. Therefore, the learned features do not strengthen the sentiment vocabulary in a sentence. Consequently, this paper proposes a sentiment-based masked language modeling method to predict sentence-level valence–arousal scores in Chinese. The proposed method is called dimensional valence–arousal based on bidirectional encoder representations from transformers (DVA-BERT), which combines the BERT model with specific sentiment word masking. The proposed method involves two learning tasks: valence–arousal intensity estimation, which is the major task, and random masked sentiment word prediction, which is the auxiliary task modify from mask language modeling, used to enhance the model performance. The experimental results indicate that the proposed DVA-BERT model can identify effective sentiment features by masking sentiment words and can outperform the original BERT model and other word masking methods.
更多查看译文
关键词
Deep learning, Masked language modeling, Emotion recognition, Sentiment analysis, Valence–arousal model
AI 理解论文
溯源树
样例
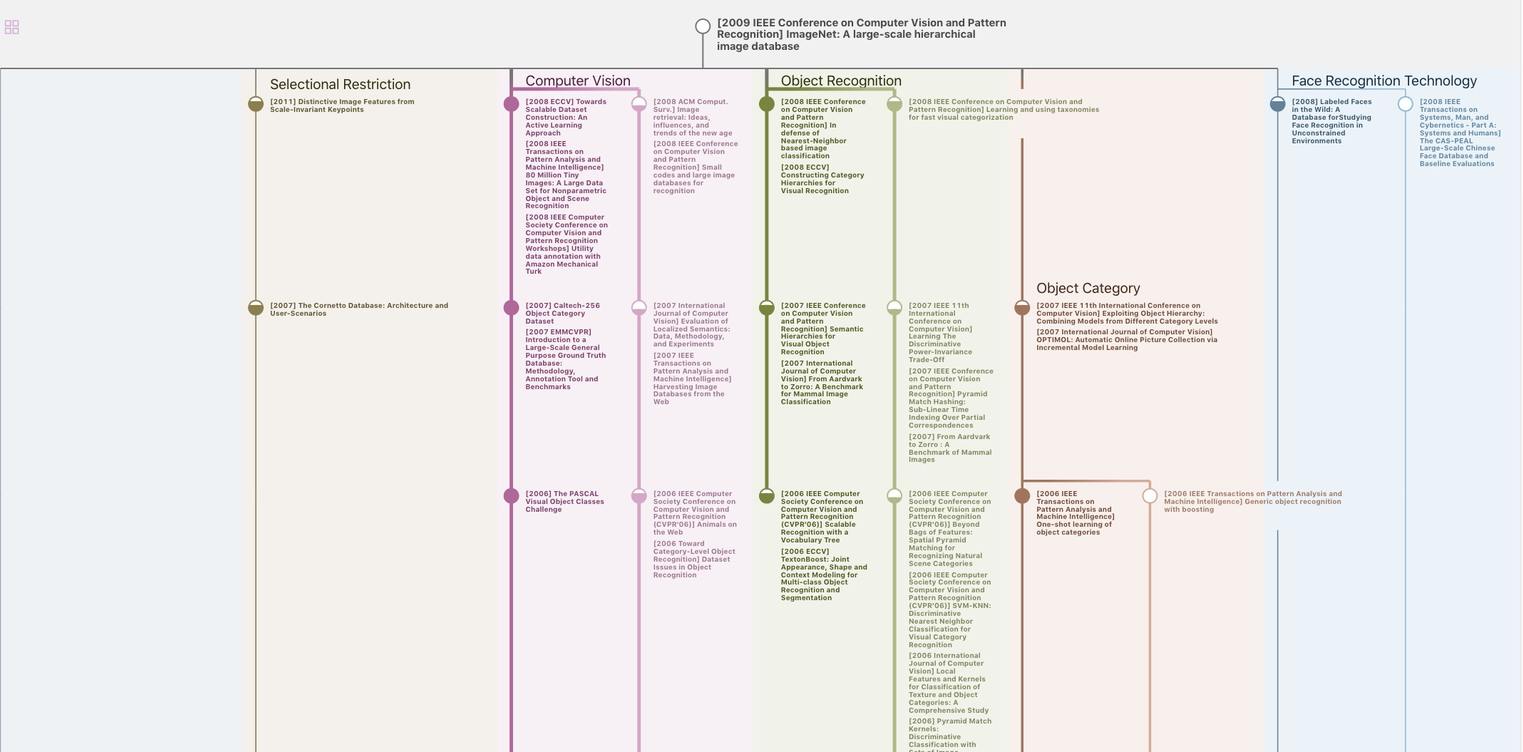
生成溯源树,研究论文发展脉络
Chat Paper
正在生成论文摘要