Reinforced model predictive control (RL-MPC) for building energy management
Applied Energy(2022)
摘要
Buildings need advanced control for the efficient and climate-neutral use of their energy systems. Model predictive control (MPC) and reinforcement learning (RL) arise as two powerful control techniques that have been extensively investigated in the literature for their application to building energy management. These methods show complementary qualities in terms of constraint satisfaction, computational demand, adaptability, and intelligibility, but usually a choice is made between both approaches. This paper compares both control approaches and proposes a novel algorithm called reinforced predictive control (RL-MPC) that merges their relative merits. First, the complementarity between RL and MPC is emphasized on a conceptual level by commenting on the main aspects of each method. Second, the RL-MPC algorithm is described that effectively combines features from each approach, namely state estimation, dynamic optimization, and learning. Finally, MPC, RL, and RL-MPC are implemented and evaluated in BOPTEST, a standardized simulation framework for the assessment of advanced control algorithms in buildings. The results indicate that pure RL cannot provide constraint satisfaction when using a control formulation equivalent to MPC and the same controller model for learning. The new RL-MPC algorithm can meet constraints and provide similar performance to MPC while enabling continuous learning and the possibility to deal with uncertain environments.
更多查看译文
关键词
Model predictive control,Reinforcement learning,Reinforced model predictive control,Building automation,BOPTEST
AI 理解论文
溯源树
样例
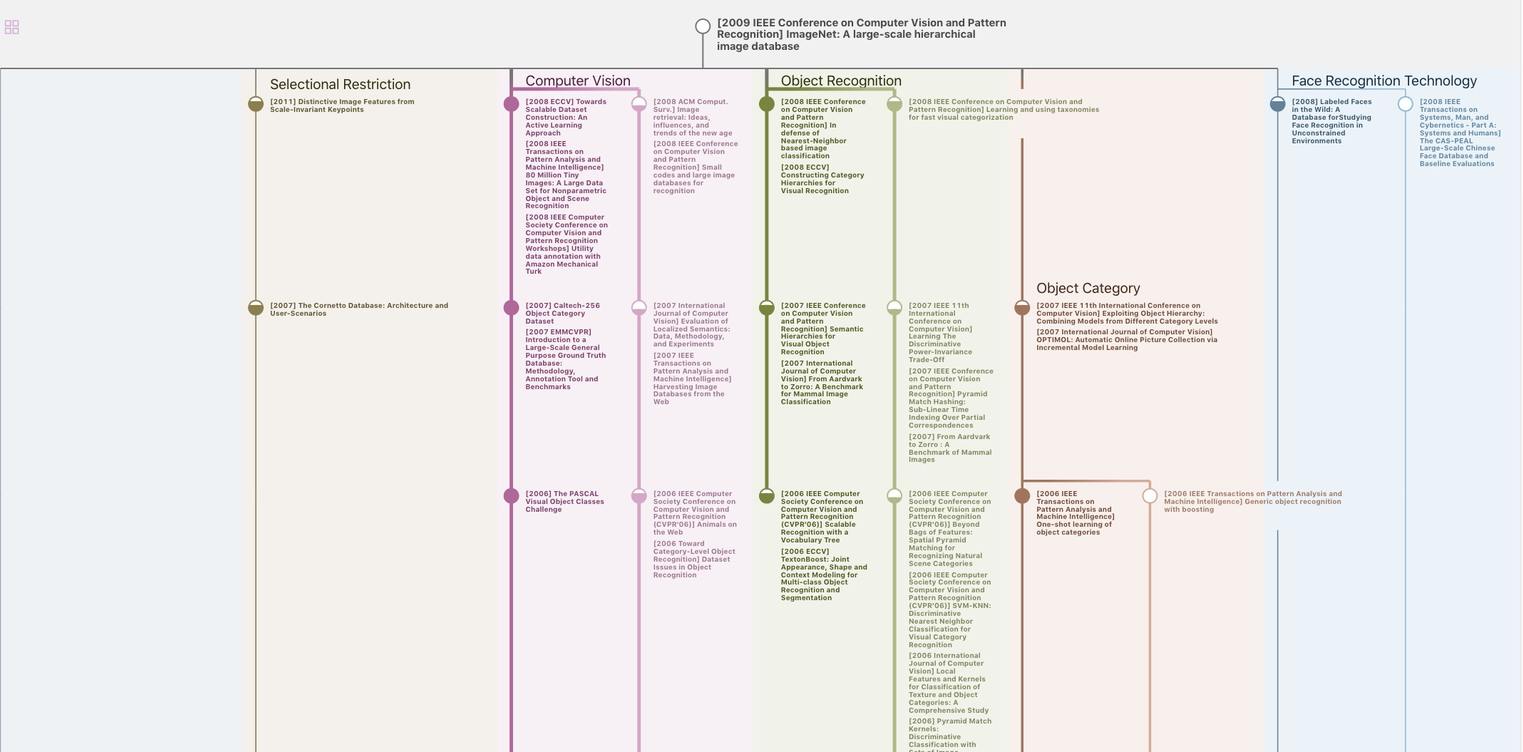
生成溯源树,研究论文发展脉络
Chat Paper
正在生成论文摘要