Deep Neural Network Based Reconciliation for CV-QKD
PHOTONICS(2022)
摘要
High-speed reconciliation is indispensable for supporting the continuous-variable quantum key distribution (CV-QKD) system to generate the secure key in real-time. However, the error correction process's high complexity and low processing speed limit the reconciliation speed. Therefore, reconciliation has also become the bottleneck of system performance. In this paper, we proposed a high-speed reconciliation scheme that uses the deep neural network to optimize the decoding process of the low-density parity-check (LDPC) code. We first introduced a network structure of decoding implementation based on the deep neural network, which can be applied to decoding algorithms of parallel strategy and significantly reduce the decoding complexity. Subsequently, we proposed two improved decoding algorithms based on this structure, named linear fitting algorithm and deep neural network-assisted decoding algorithm. Finally, we introduced a high-speed reconciliation scheme based on the CPU-GPU hybrid platform. Simulation results show that the proposed reconciliation scheme reduces the complexity and enables us to realize the high-speed CV-QKD system. Furthermore, the improved decoding algorithm can also reduce the FER, thereby increasing the secret key rate.
更多查看译文
关键词
CV-QKD, high-speed reconciliation, LDPC code, deep neural network, CPU-GPU hybrid platform
AI 理解论文
溯源树
样例
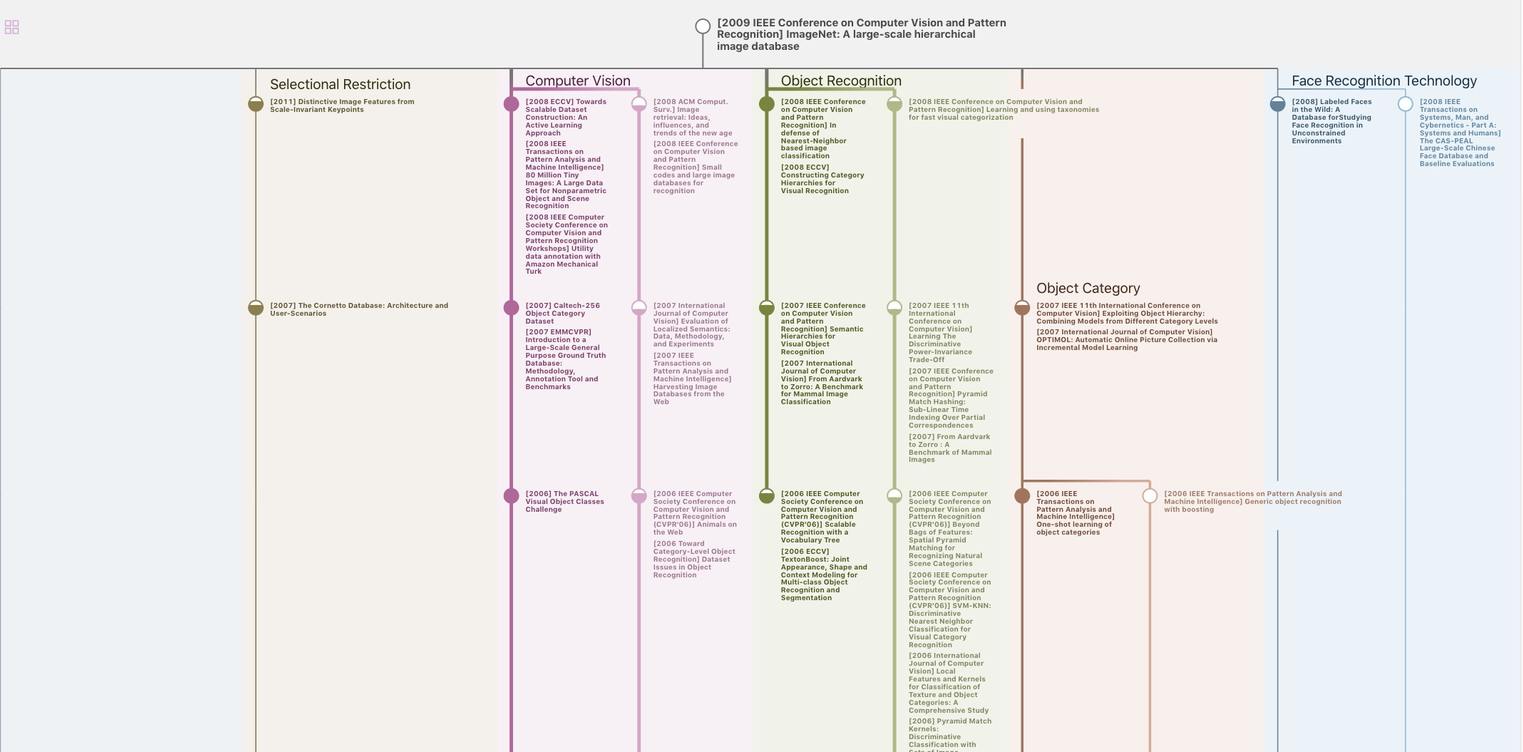
生成溯源树,研究论文发展脉络
Chat Paper
正在生成论文摘要