Few-shot learning with hierarchical pooling induction network
Multimedia Tools and Applications(2022)
摘要
Learning to recognize new concepts from few-shot examples is a long-standing challenge in modern computer vision. Metric based few-shot learning is a prevalent way towards this goal where new query instances are classified to the support classes by comparing the query instance with each class prototype. To this end, a representative and discriminative class prototype should necessarily be induced from several support example features. In this work, we propose a simple but effective hierarchical pooling induction method to learn such generalized class-level representations, by concatenating the max pooling and mean pooling operations. The proposed induction method could form a representative prototype for given few-shot samples, enhancing both the discrimination of the intermediate features and the final classification performance. The benchmark miniImageNet dataset and some other practical Remote Sensing Image Scene Classification (RESISC) datasets are employed for generalization investigation and it shows that the proposed induction module could improve the performance of state-of-the-art method and outperforms other alternative induction methods. Qualitative visualization and quantitative analysis are also provided to demonstrate the effectiveness and robustness of the proposed method.
更多查看译文
关键词
Few-shot learning,Transfer learning,Hierarchical pooling induction,Remote sensing image scene classification
AI 理解论文
溯源树
样例
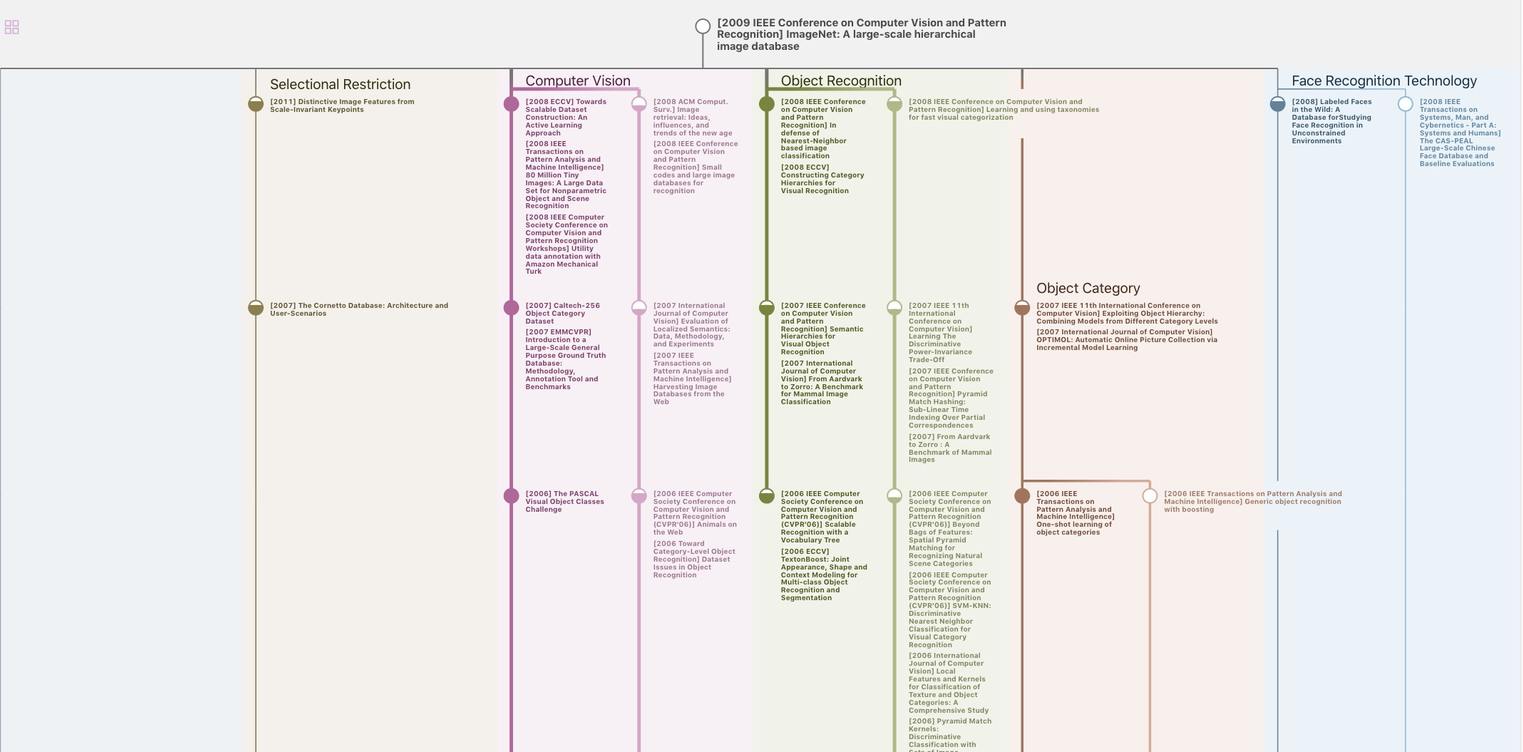
生成溯源树,研究论文发展脉络
Chat Paper
正在生成论文摘要