A Review of Data-Driven Machinery Fault Diagnosis Using Machine Learning Algorithms
Journal of Vibration Engineering & Technologies(2022)
摘要
Purpose This article aims to systematically review the recent research advances in data-driven machinery fault diagnosis based on machine learning algorithms, and provide valuable guidance for future research directions in this field. Methods This article reviews the research results of data-driven fault diagnosis methods of recent years, and it includes the application status and research progress of machinery fault diagnosis in three frameworks: shallow machine learning (SML), deep learning (DL), and transfer learning (TL). Many publications on this topic are classified and summarized. The related theories, application research, advantages, and disadvantages of several main algorithms under each framework are discussed. Results It has shown that SML-based diagnosis models are simple, reliable, and fast to train. For relatively uncomplicated systems, SML-based diagnosis models still have important applications. For diagnosis tasks with large amounts of training samples and the pursuit of higher accuracy, DL-based diagnosis models can provide end-to-end diagnostic services for complex systems as well as compound faults. TL-based diagnosis models can realize knowledge transfer across conditions, machines, and even fields to solve the problems of data scarcity and sample imbalance that often occur in fault diagnosis. However, in the face of increasingly complex engineering systems, the applications of machine learning algorithms in machinery fault diagnosis are still challenging. Conclusions In future research, the fusion of different machine learning frameworks could solve the problems of inadequate feature extraction and slow training of diagnostic models. Transformer neural network based on pure attention mechanism breaks through the shortcomings of LSTM neural network which cannot be computed in parallel, and it is a worthy research direction in the field of fault diagnosis. In addition, machinery fault diagnosis method based on machine learning algorithms also has great potential for improvement in transferability, federated transfer learning, and strong noise background. These proposed future research directions can provide new ideas for researchers to promote the development of machine learning algorithms in machinery fault diagnosis.
更多查看译文
关键词
Fault diagnosis, Data-driven, Machine learning, Deep learning, Transfer learning
AI 理解论文
溯源树
样例
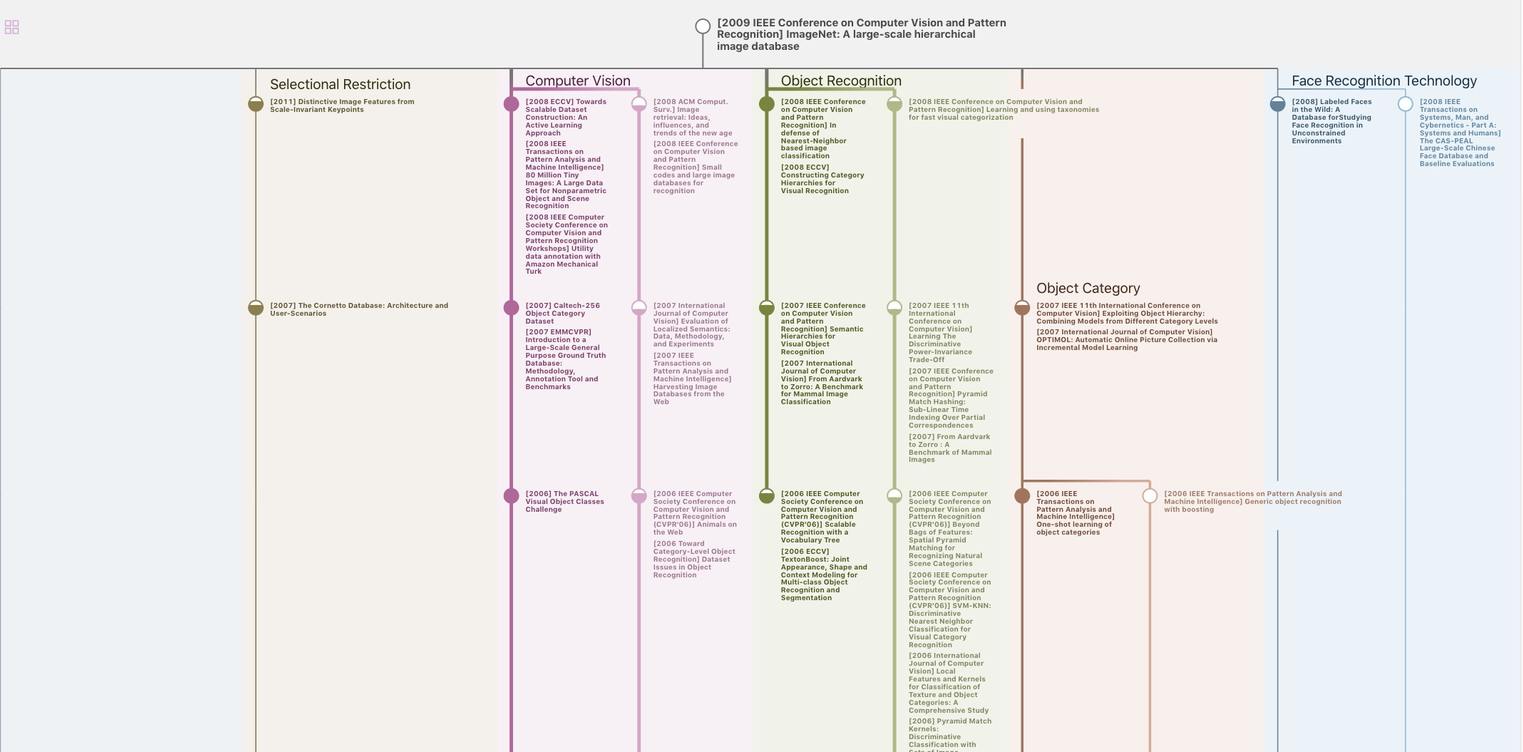
生成溯源树,研究论文发展脉络
Chat Paper
正在生成论文摘要