A novel attention-based domain adaptation model for intelligent bearing fault diagnosis under variable working conditions
MEASUREMENT SCIENCE AND TECHNOLOGY(2022)
摘要
In recent years, transfer learning technology has developed rapidly and has been widely used in bearing fault diagnosis. Most existing methods mainly align the overall feature distribution of the signal samples across the source and target domains. However, the transferability of each signal and each segment of a signal sample is different. Therefore, in this paper, a novel attention-based domain adaptation model (ADA) is proposed. The ADA model consists of a feature extractor and an ADA module. The feature extractor is built by separable convolution with channel attention module and length attention module to improve the reliability of feature learning. The ADA module consists of two parts, the local ADA module and the global ADA module to enhance the model's domain adaptation ability by focusing on the signals and signal segments with better transferability. The experimental results show that the ADA model is superior to other intelligent fault diagnosis methods based on transfer learning under variable working conditions.
更多查看译文
关键词
domain adaptation, bearing fault diagnosis, adversarial network, attention mechanism
AI 理解论文
溯源树
样例
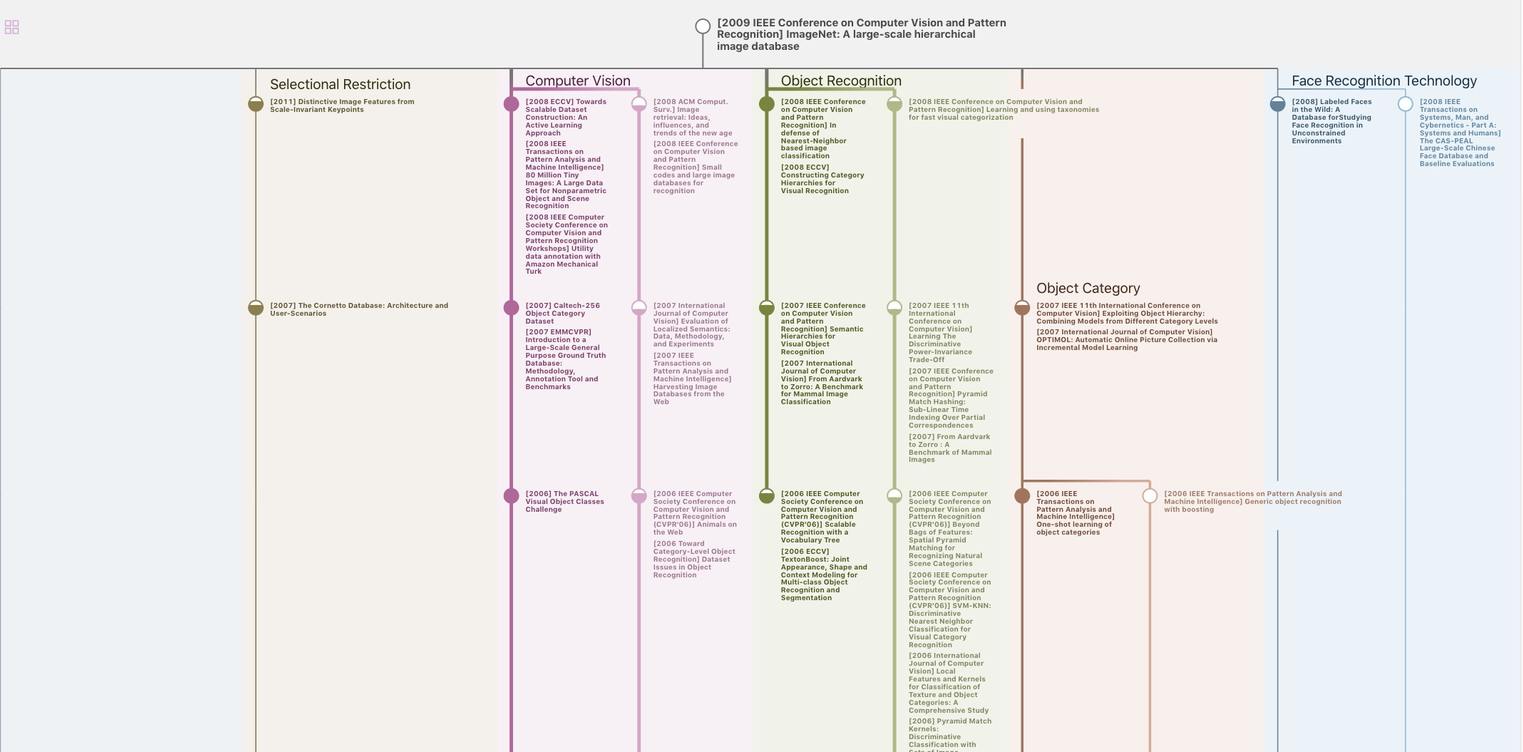
生成溯源树,研究论文发展脉络
Chat Paper
正在生成论文摘要