Machine Learning to Predict Area Fugitive Emission Fluxes of GHGs from Open-Pit Mines
ATMOSPHERE(2022)
摘要
Greenhouse gas (GHG) emissions from open-pit mines pose a global climate challenge, which necessitates appropriate quantification to support effective mitigation measures. This study considers the area-fugitive methane advective flux (as a proxy for emission flux) released from a tailings pond and two open-pit mines, denominated "old " and "new ", within a facility in northern Canada. To estimate the emission fluxes of methane from these sources, this research employed near-surface observations and modeling using the weather research and forecasting (WRF) passive tracer dispersion method. Various machine learning (ML) methods were trained and tested on these data for the operational forecasting of emissions. Predicted emission fluxes and meteorological variables from the WRF model were used as training and input datasets for ML algorithms. A series of 10 ML algorithms were evaluated. The four models that generated the most accurate forecasts were selected. These ML models are the multi-layer perception (MLP) artificial neural network, the gradient boosting (GBR), XGBOOST (XGB), and support vector machines (SVM). Overall, the simulations predicted the emission fluxes with R-2 (-) values higher than 0.8 (-). Considering the bias (Tonnes h(-1)), the ML predicted the emission fluxes within 6.3%, 3.3%, and 0.3% of WRF predictions for the old mine, new mine, and the pond, respectively.
更多查看译文
关键词
emission flux, machine learning (ML) method, open-pit mines, weather research and forecasting (WRF)
AI 理解论文
溯源树
样例
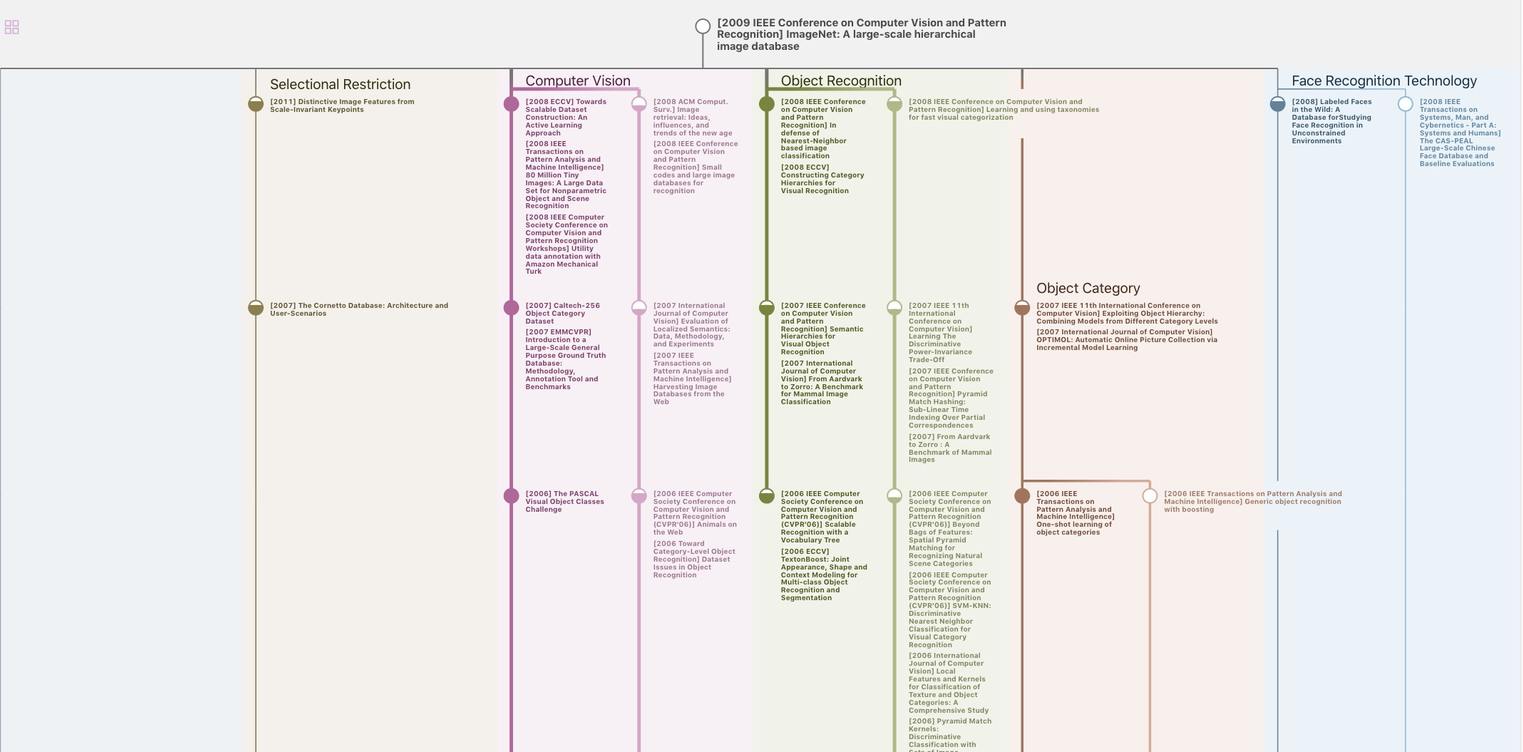
生成溯源树,研究论文发展脉络
Chat Paper
正在生成论文摘要