Convolutional Neural Network Based on Diverse Gabor Filters for Deepfake Recognition
IEEE ACCESS(2022)
摘要
Media synthesis and manipulation has reached unprecedented levels of realism owing to the proliferation of deep learning. Deepfake has been the de-facto tool for media manipulation. Although this technology has potential in the entertainment industry, its threats include political manipulation and bypassing biometric security systems. As a result, deepfake detection has garnered widespread attention among research communities. The intuition is to use deep learning to fix the problems created by deep learning. Although convolutional neural networks have shown their dominance in the filed of pattern recognition, the receptive field-model size dilemma still persists along with the lack of interpretation for such models. While the traditional Gabor function was proposed to fix these problems, it can only generate limited linear Gabor filters which makes it optimal for limited data and applications. The contribution of this paper is quadruple: (i) proposing a unified Gabor function capable of generating linear, elliptical, and circular Gabor filters. (ii) leveraging the back-propagation learning framework to incorporate the proposed function in convolutional neural networks and generate adaptive Gabor filters. (iii) presenting a dual scale large receptive field network for deepfake image recognition. (iv) demonstrating where the proposed model stands in terms of performance and architecture size compared to state-of-the-art models. The proposed model is evaluated on four benchmark datasets: Celeb-DF (v2), DeepFake Detection Challenge Preview, FaceForensics++ and Wilddeepfake. Experimental results show that the proposed adaptive Gabor filters reduce the model size by 64.9% compared to adaptive weighted filters without performance reduction.
更多查看译文
关键词
Gabor filters, Videos, Information integrity, Feature extraction, Face recognition, Adaptation models, Convolutional neural networks, Compact neural networks, image classification, image forensics, learnable filters, pattern recognition
AI 理解论文
溯源树
样例
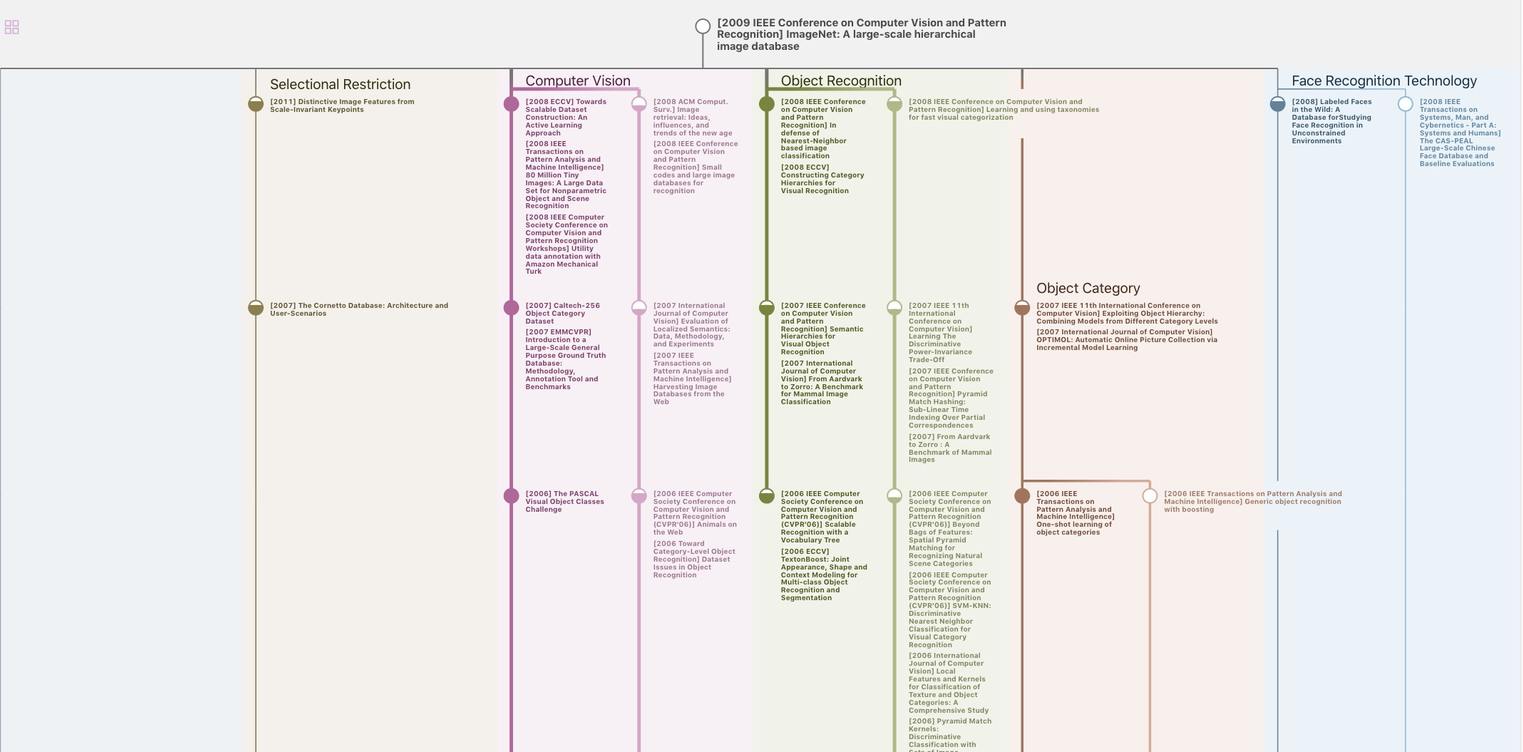
生成溯源树,研究论文发展脉络
Chat Paper
正在生成论文摘要