Grid Representation in Neural Networks for Automated Planning
ICAART: PROCEEDINGS OF THE 14TH INTERNATIONAL CONFERENCE ON AGENTS AND ARTIFICIAL INTELLIGENCE - VOL 3(2022)
摘要
Automated planning and machine learning create a powerful combination of tools which allows us to apply general problem solving techniques to problems that are not modeled using classical planning techniques. In real-world scenarios and complex domains, creating a standardized representation is often a bottleneck as it has to be modeled by a human. That often limits the usage of planning algorithms to real-world problems. The standardized representation is also not a suitable for neural network processing and often requires further transformation. In this work, we focus on presenting three different grid representations that are well suited to model a variety of classical planning problems which can be then processed by neural networks without further modifications. We also analyze classical planning benchmarks in order to find domains that correspond to our proposed representations. Furthermore, we also show that domains that are not explicitly defined on a grid can be represented on a grid with minor modifications that are domain specific. We discuss advantages and drawbacks of our proposed representations, provide examples for many planning benchmarks and also discuss the importance of data and its structure when training neural networks for planning.
更多查看译文
关键词
Classical Planning, Domain-independent Planning, Neural Networks, Problem Representation
AI 理解论文
溯源树
样例
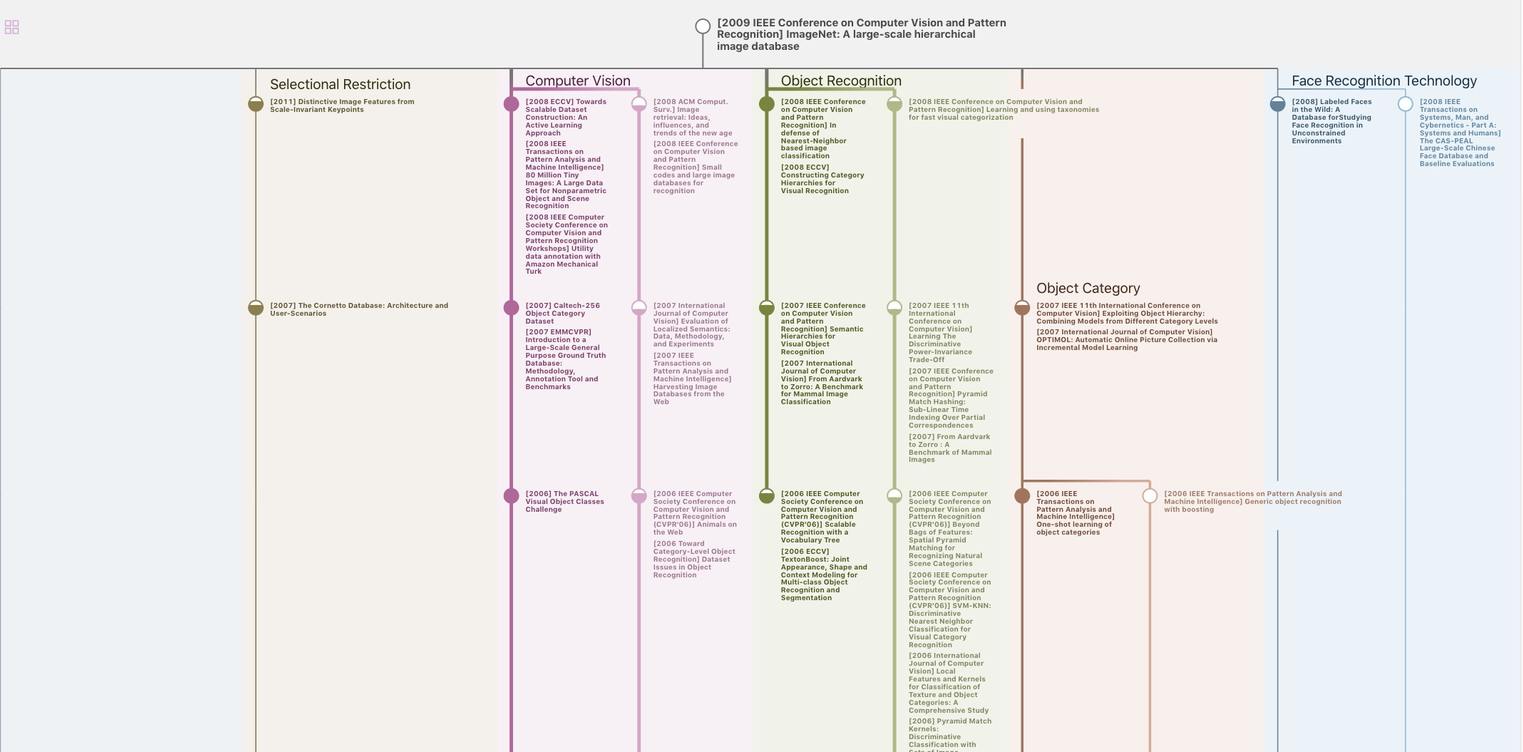
生成溯源树,研究论文发展脉络
Chat Paper
正在生成论文摘要