Machine-learning-enabled discrete element method: Contact detection and resolution of irregular-shaped particles
INTERNATIONAL JOURNAL FOR NUMERICAL AND ANALYTICAL METHODS IN GEOMECHANICS(2022)
摘要
This paper presents a machine learning (ML)-enabled discrete element method (DEM) for the computational mechanics of irregular-shaped particles. ML-enabled DEM, as with most conventional DEMs, encompasses four main steps in one typical calculation cycle, namely, (1) the detection and resolution of contacts, (2) the evaluation of contact behavior, (3) the calculation of particle motion, and (4) the updating of particle geometric descriptions. Unlike conventional DEMs, the proposed method constructs and employs neural networks to detect particle contacts and resolve contact geometric features. Neural networks take particle geometric descriptors as inputs and output the contact status and contact geometric features. Using two-dimensional elliptical particles as an example, the performance of the ML-enabled DEM is investigated through five numerical experiments and compared with analytical solutions or conventional DEM methods. A sixth numerical experiment involving irregular-shaped particles is also presented to showcase the potential and applicability of the proposed method for other particle shapes. ML-enabled DEM can accurately capture the trajectory and energy evolution of individual particles, the fabric characteristics of dense packing, and the mechanical behavior of packing under large loads, while demonstrating computational efficiency over conventional methods.
更多查看译文
关键词
contact detection and resolution, discrete element method, machine learning, neural network, particle shape
AI 理解论文
溯源树
样例
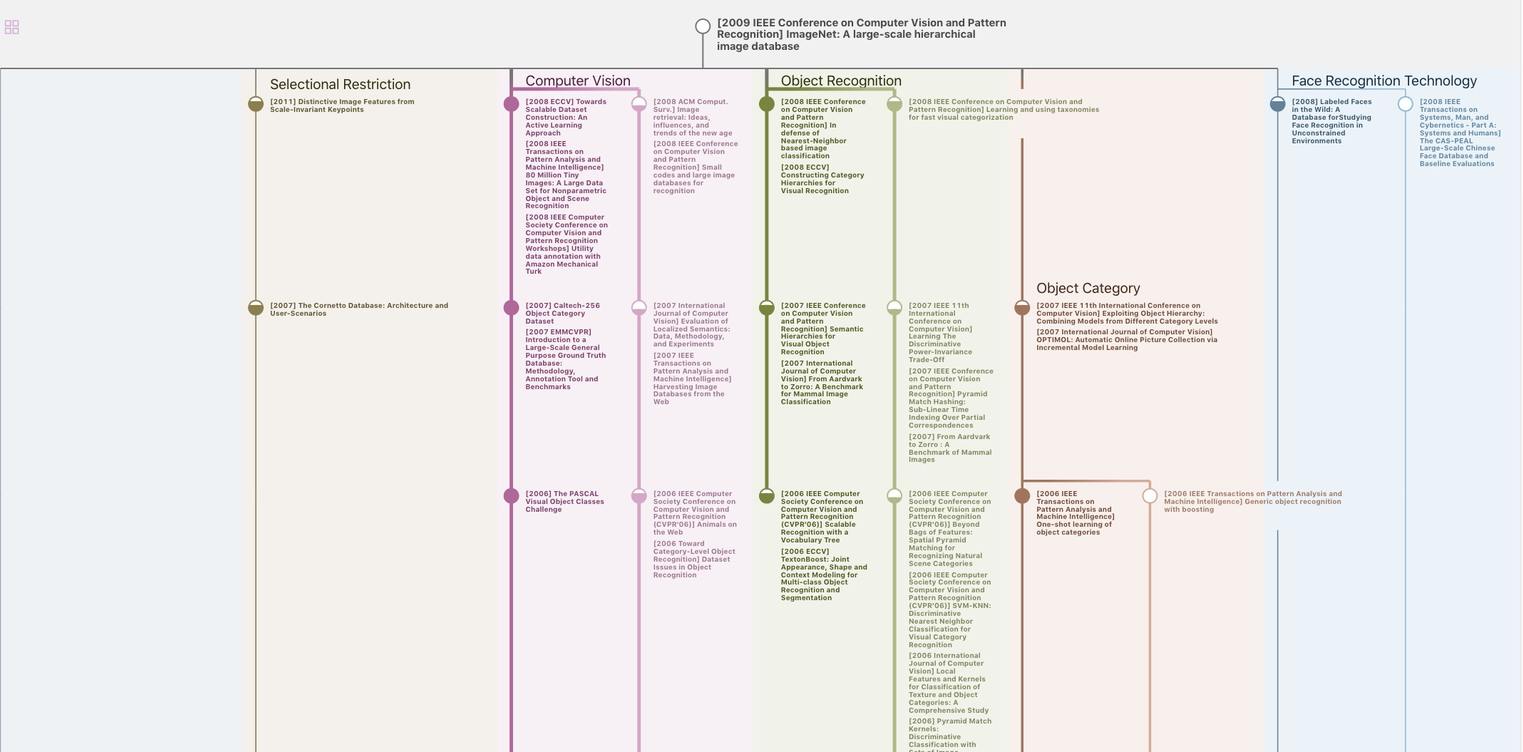
生成溯源树,研究论文发展脉络
Chat Paper
正在生成论文摘要