Image classification with parallel KPCA-PCA network
COMPUTATIONAL INTELLIGENCE(2022)
摘要
Principal component analysis (PCA) is widely used in computer vision for object detection. In this article, we take advantage of the algorithms of PCA and kernel principal component analysis (KPCA) to construct a deep learning model named parallel KPCA-PCA network (PK-PCANet). In the proposed model, both of the given PCA and KPCA algorithm are aiming to calculate the filters that will be used in the following convolution layers. The extracted features from PCANet and KPCANet are fused by the strategy of parallel feature fusion. With the aim of reducing the dimensionality of the learned features, the algorithm of compressed sensing is incorporated in the proposed network. According to the cooperative advantages of deep learning network and compressed sensing, the proposed PK-PCANet model obtains some improvements in several visual recognition tasks. Extensively experiments are performed on face recognition, hand-written digit recognition and object classification, and the experimental results on various image classification benchmarks such as Extended Yale B, AR, MNIST, CIFAR-10, and VOC 2007 validated the efficiency of the proposed method of PK-PCANet.
更多查看译文
关键词
image classification, PCANet, PK-PCANet, principal component analysis
AI 理解论文
溯源树
样例
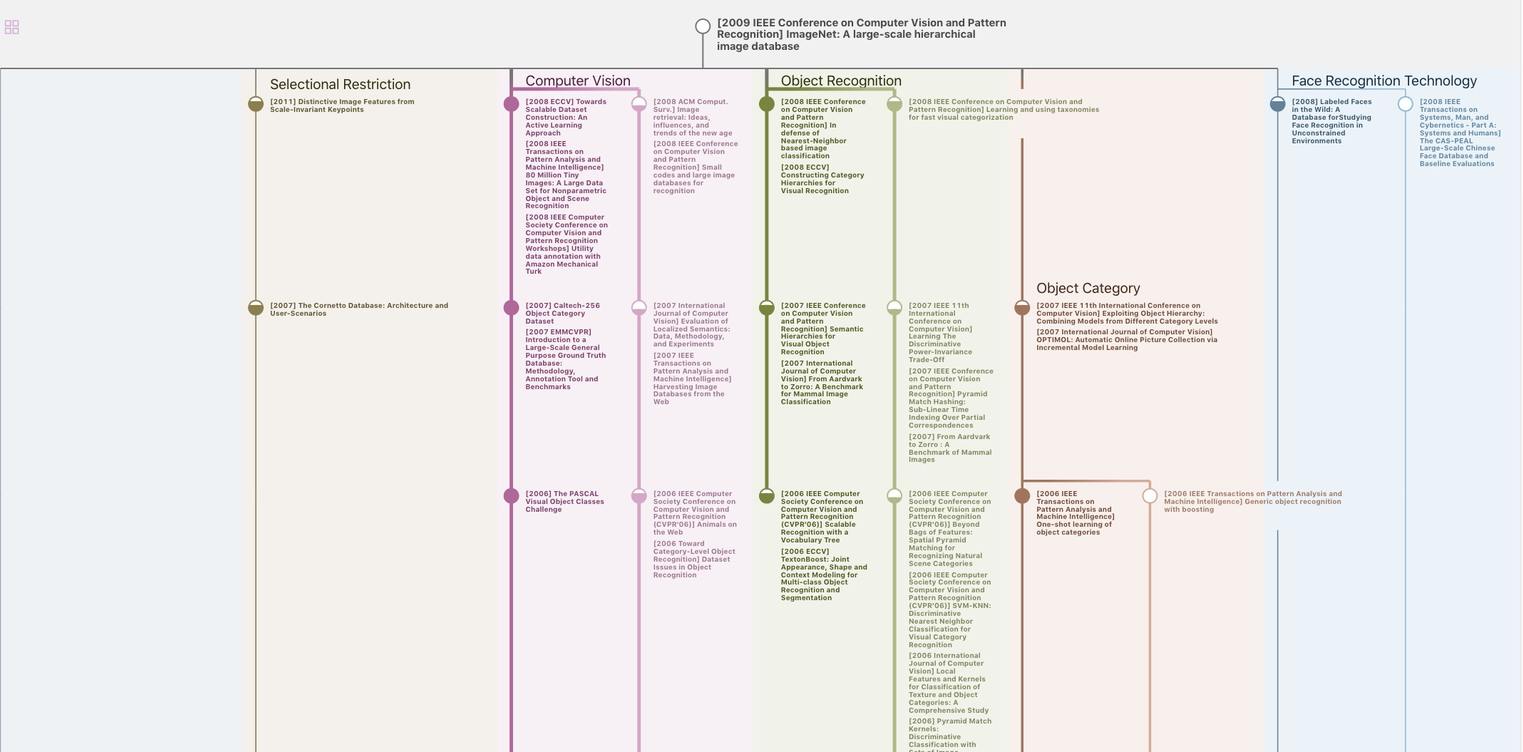
生成溯源树,研究论文发展脉络
Chat Paper
正在生成论文摘要