Comparing Performances of CNN, BP, and SVM Algorithms for Differentiating Sweet Pepper Parts for Harvest Automation
APPLIED SCIENCES-BASEL(2021)
摘要
For harvest automation of sweet pepper, image recognition algorithms for differentiating each part of a sweet pepper plant were developed and performances of these algorithms were compared. An imaging system consisting of two cameras and six halogen lamps was built for sweet pepper image acquisition. For image analysis using the normalized difference vegetation index (NDVI), a band-pass filter in the range of 435 to 950 nm with a broad spectrum from visible light to infrared was used. K-means clustering and morphological skeletonization were used to classify sweet pepper parts to which the NDVI was applied. Scale-invariant feature transform (SIFT) and speeded-up robust features (SURFs) were used to figure out local features. Classification performances of a support vector machine (SVM) using the radial basis function kernel and backpropagation (BP) algorithm were compared to classify local SURFs of fruits, nodes, leaves, and suckers. Accuracies of the BP algorithm and the SVM for classifying local features were 95.96 and 63.75%, respectively. When the BP algorithm was used for classification of plant parts, the recognition success rate was 94.44% for fruits, 84.73% for nodes, 69.97% for leaves, and 84.34% for suckers. When CNN was used for classifying plant parts, the recognition success rate was 99.50% for fruits, 87.75% for nodes, 90.50% for leaves, and 87.25% for suckers.
更多查看译文
关键词
NDVI, image processing, SURF, SIFT, SVM, BP algorithm, performance, sweet pepper, deep neural network
AI 理解论文
溯源树
样例
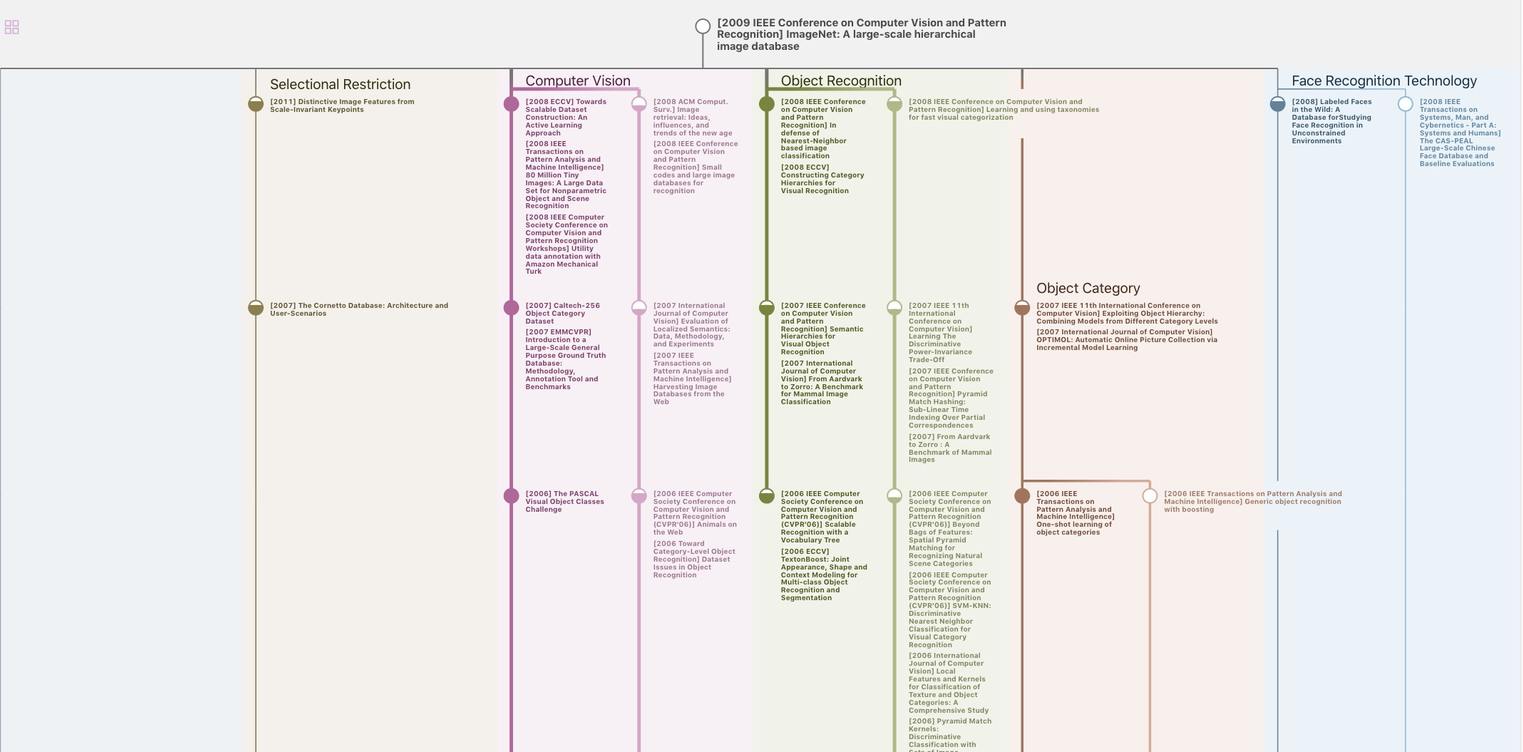
生成溯源树,研究论文发展脉络
Chat Paper
正在生成论文摘要