A Novel Discriminative Virtual Label Regression Method for Unsupervised Feature Selection
IEICE TRANSACTIONS ON INFORMATION AND SYSTEMS(2022)
摘要
Unsupervised Feature selection is an important dimensionality reduction technique to cope with high-dimensional data. It does not require prior label information, and has recently attracted much attention. However, it cannot fully utilize the discriminative information of samples, which may affect the feature selection performance. To tackle this problem, in this letter, we propose a novel discriminative virtual label regression method (DVLR) for unsupervised feature selection. In DVLR, we develop a virtual label regression function to guide the subspace learning based feature selection, which can select more discriminative features. Moreover, a linear discriminant analysis (LDA) term is used to make the model be more discriminative. To further make the model be more robust and select more representative features, we impose the l(2,1)-norm on the regression and feature selection terms. Finally, extensive experiments are carried out on several public datasets, and the results demonstrate that our proposed DVLR achieves better performance than several state-of-the-art unsupervised feature selection methods.
更多查看译文
关键词
feature selection, subspace learning, virtual label, linear discriminant analysis
AI 理解论文
溯源树
样例
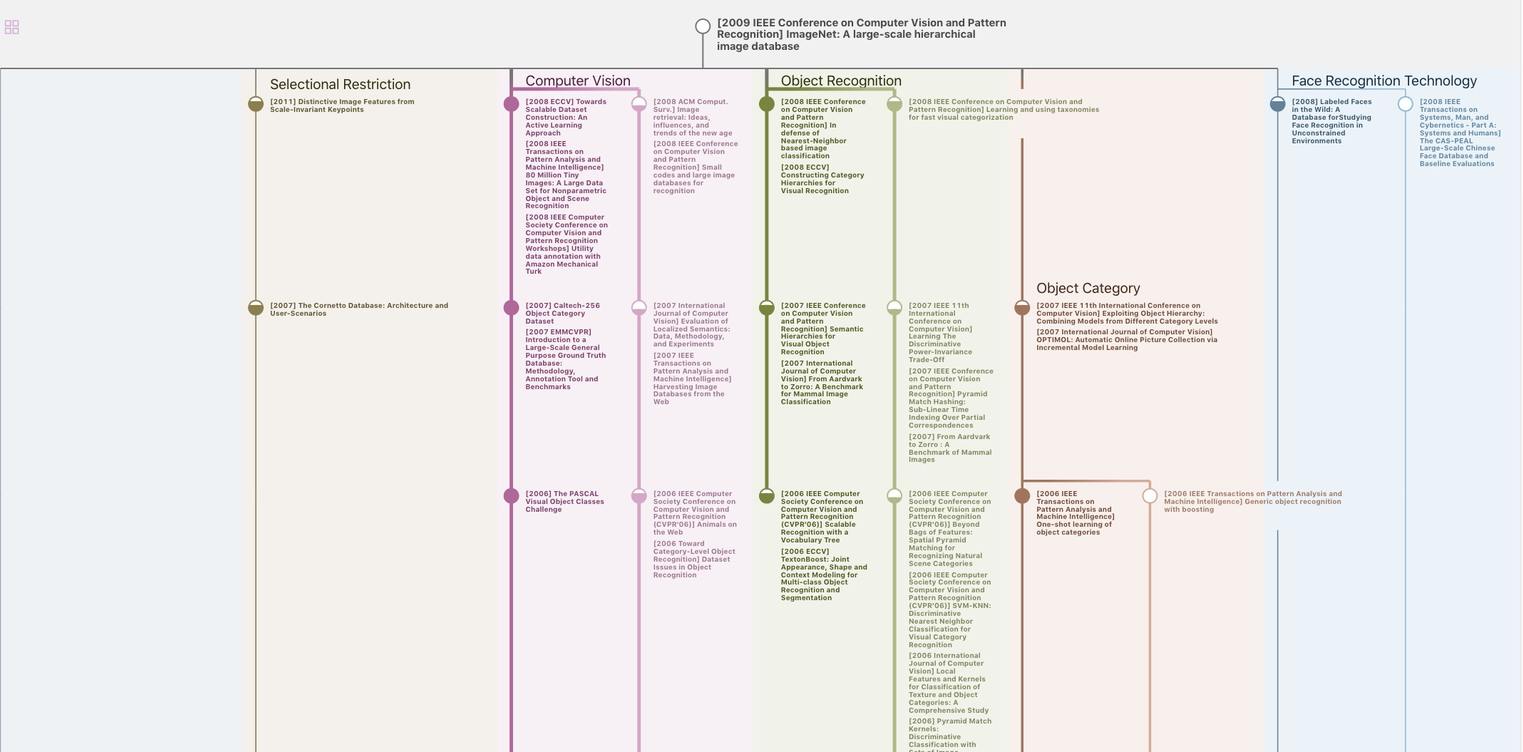
生成溯源树,研究论文发展脉络
Chat Paper
正在生成论文摘要