Modified residual networks for severity stage classification of diabetic retinopathy
EVOLVING SYSTEMS(2022)
摘要
Diabetic Retinopathy is a common microvascular complication associated with diabetes and also one of the main reason for blindness globally. Manual diagnosis of diabetic retinopathy (DR) by ophthalmologists is time consuming and tedious task. This paper proposes modified deep residual networks for binary and multistage classification of DR. The proposed models have been evaluated on publicly available MESSIDOR dataset. For binary classification, the modified ResNet18, ResNet34 and ResNet50 models show an accuracy of 99.47%, 99.47% and 99.87% respectively. The Multistage classification accuracy obtained using modified ResNet18 is 99.37%, modified Resnet34 is 99.16% and modified ResNet50 is 99.37%. Further, a comparison of the proposed model with the models in literature shows an overall accuracy improvement by at least 0.83% and more than 5% for binary and multistage classification respectively. It has been observed that the proposed DR classification models outperform the existing methods on the benchmark dataset.
更多查看译文
关键词
Diabetic retinopathy,Computer-aided diagnosis,CNN,Deep learning,Residual networks
AI 理解论文
溯源树
样例
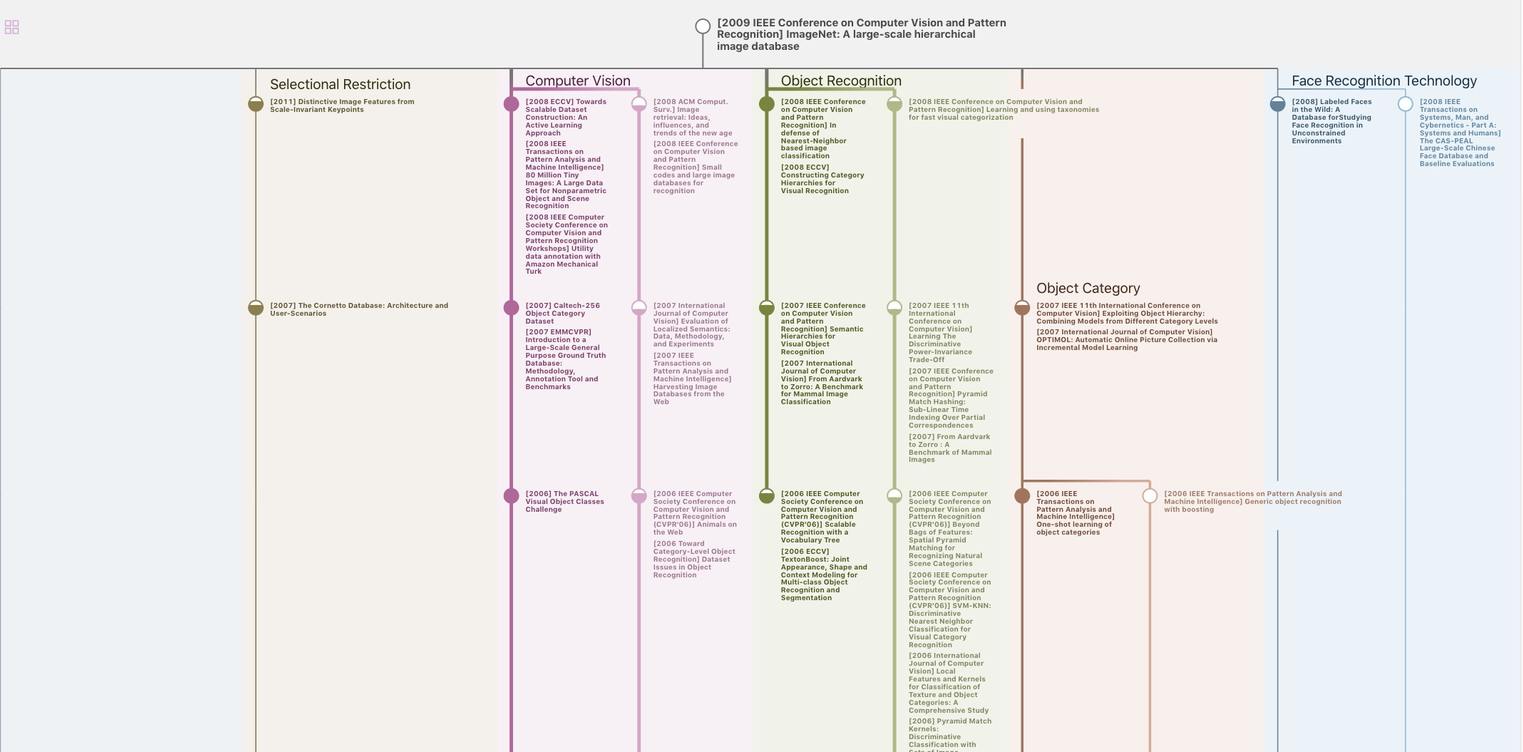
生成溯源树,研究论文发展脉络
Chat Paper
正在生成论文摘要