Designing Monte Carlo Simulation and an Optimal Machine Learning to Optimize and Model Space Missions
IEEE ACCESS(2022)
摘要
This paper investigates applying artificial intelligence (AI) algorithms to attitude control system of satellites to optimally tune the controller using high performance computing. This methodology is applied to the Virtual Telescope for X-ray Observation mission, which is a precise formation of two separate spacecraft observing multiple objects in the space in the X-ray domain. The mission is divided into phases based on the instrumentation and the mission goal. To reach an stable precise formation robust to stochastic slew and slew rate (i.e., Euler angles and angular velocities) in a minimal constrained time T, consumed energy of the attitude control system, denoted as E, and root-mean-square state error of attitude control system, denoted as e, are minimized. Monte-Carlo simulation is used for the sensitivity analysis of optimization and designing a controller. Deep neural networks (DNN), Gaussian processes (GP), and support vector regression (SVR) learn this optimization as a surrogate model, while their hyperparameters are optimized in a novel approach. THETA supercomputer at Argonne Leadership Computing Facility (ALCF) is used for optimizing the hyperparameters of DNN. The surrogate model meets the requirements of the mission, and it shows a better performance over the optimization and Monte-Carlo. The optimal DNN can satisfy the mission requirements e and T while reducing E for 90% compared to the other given methods.
更多查看译文
关键词
Virtual telescope for X-ray observation (VTXO), space mission design, Monte-Carlo simulation, optimal control, machine learning, optimization, satellites, real-time control, surrogate models, high performance computing, hyperparameters optimization
AI 理解论文
溯源树
样例
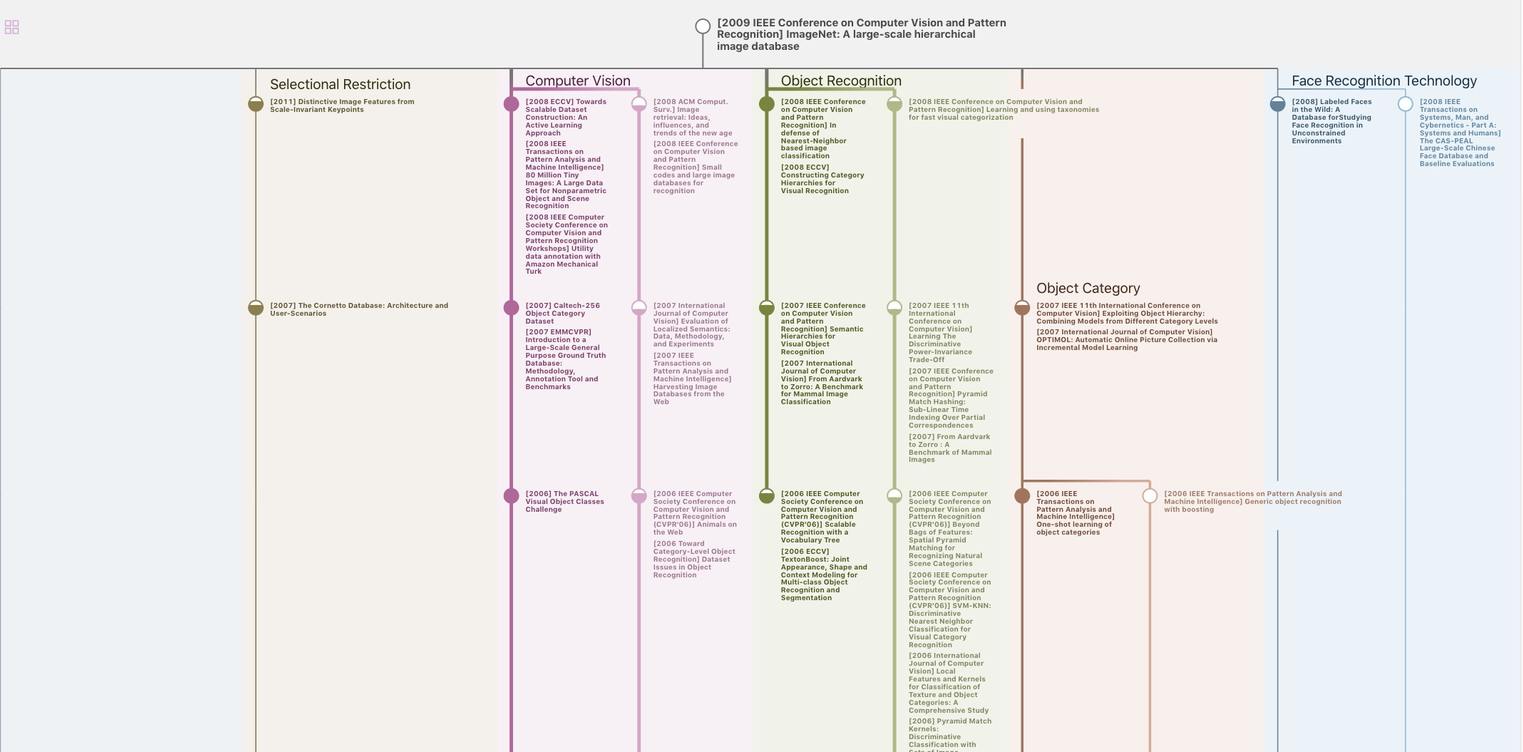
生成溯源树,研究论文发展脉络
Chat Paper
正在生成论文摘要