Machine learning-based regression analysis for estimating Cerchar abrasivity index
GEOMECHANICS AND ENGINEERING(2022)
摘要
The most widely used parameter to represent rock abrasiveness is the Cerchar abrasivity index (CAI). The CAI value can be applied to predict wear in TBM cutters. It has been extensively demonstrated that the CAI is affected significantly by cementation degree, strength, and amount of abrasive minerals, i.e., the quartz content or equivalent quartz content in rocks. The relationship between the properties of rocks and the CAI is investigated in this study. A database comprising 223 observations that includes rock types, uniaxial compressive strengths, Brazilian tensile strengths, equivalent quartz contents, quartz contents, brittleness indices, and CAIs is constructed. A linear model is developed by selecting independent variables while considering multicollinearity after performing multiple regression analyses. Machine learning-based regression methods including support vector regression, regression tree regression, k-nearest neighbors regression, random forest regression, and artificial neural network regression are used in addition to multiple linear regression. The results of the random forest regression model show that it yields the best prediction performance.
更多查看译文
关键词
Cerchar abrasivity index (CAI), machine learning, regression, rock abrasiveness, wear
AI 理解论文
溯源树
样例
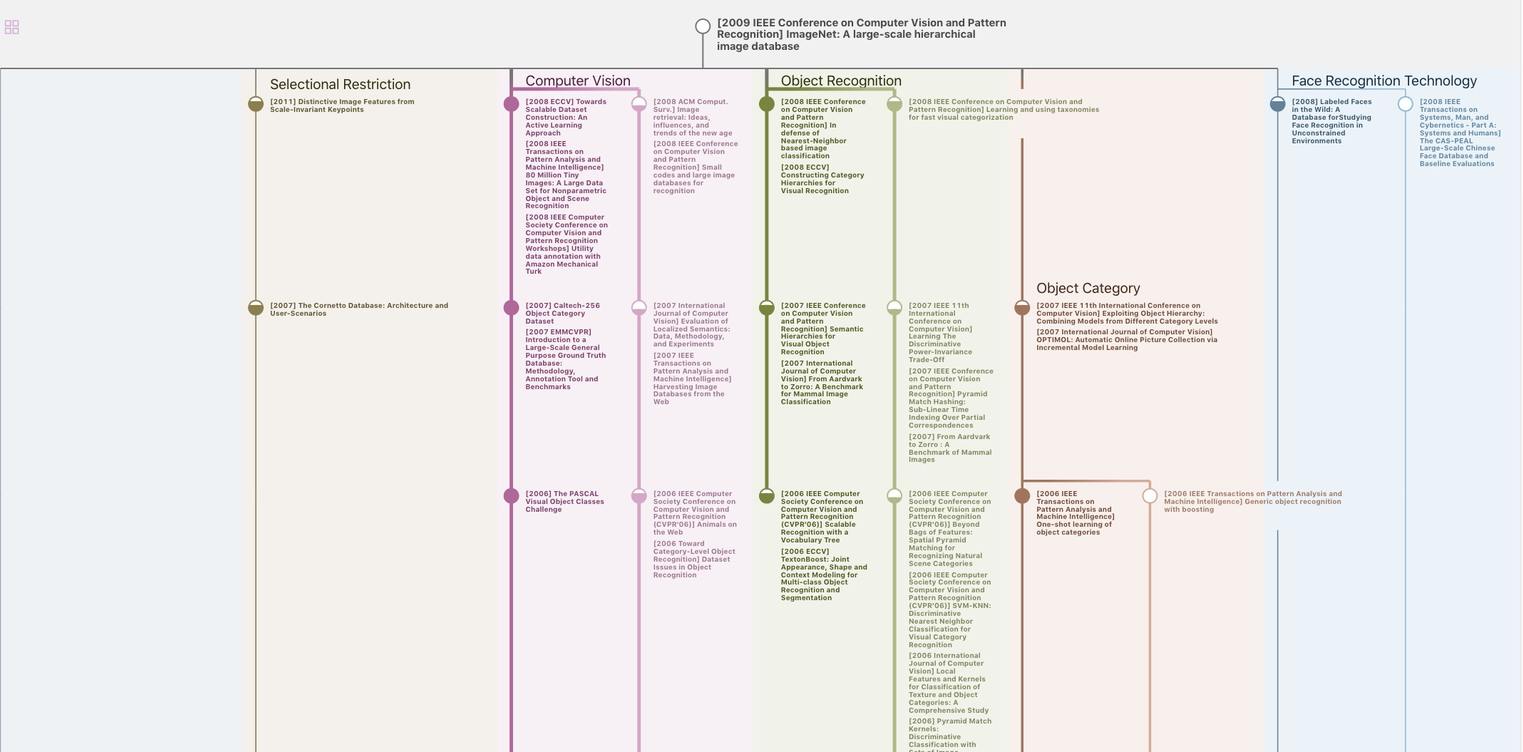
生成溯源树,研究论文发展脉络
Chat Paper
正在生成论文摘要