Rotating Machinery Fault Diagnosis Through a Transformer Convolution Network Subjected to Transfer Learning
IEEE TRANSACTIONS ON INSTRUMENTATION AND MEASUREMENT(2021)
摘要
Owing to complex operational and measurement conditions, the data available to realize the effective training of deep models are often inadequate. Compared with traditional deep networks, a Transformer exhibits a unique and excellent pattern recognition ability and has thus emerged as the de facto standard for processing tasks in many research fields. However, the application of Transformer architectures to fault diagnosis remains limited. To overcome these limitations and achieve highly accurate fault diagnosis, a novel Transformer convolution network (TCN) based on transfer learning is proposed. First, signal data are split into fixed-size patches, and the sequence of the linear embeddings of these patches is used as an input to a Transformer encoder. Subsequently, a convolutional neural network (CNN) with a classifier layer is constructed to decode and classify patterns. The TCN is pretrained in the source domain and fine-tuned in the target domain by using a transfer learning strategy. Experiments to diagnose rotating machinery faults are conducted using bearing and gearbox datasets. The average diagnostic results for four transfer experiments are 99.71%, 99.97%, 99.83%, and 100.00%, and the proposed approach significantly outperforms state-of-the-art methods. The results demonstrate the exceptional robustness and effectiveness of the proposed method.
更多查看译文
关键词
Convolutional neural network (CNN), deep learning (DL), fault diagnosis, transfer learning, Transformer
AI 理解论文
溯源树
样例
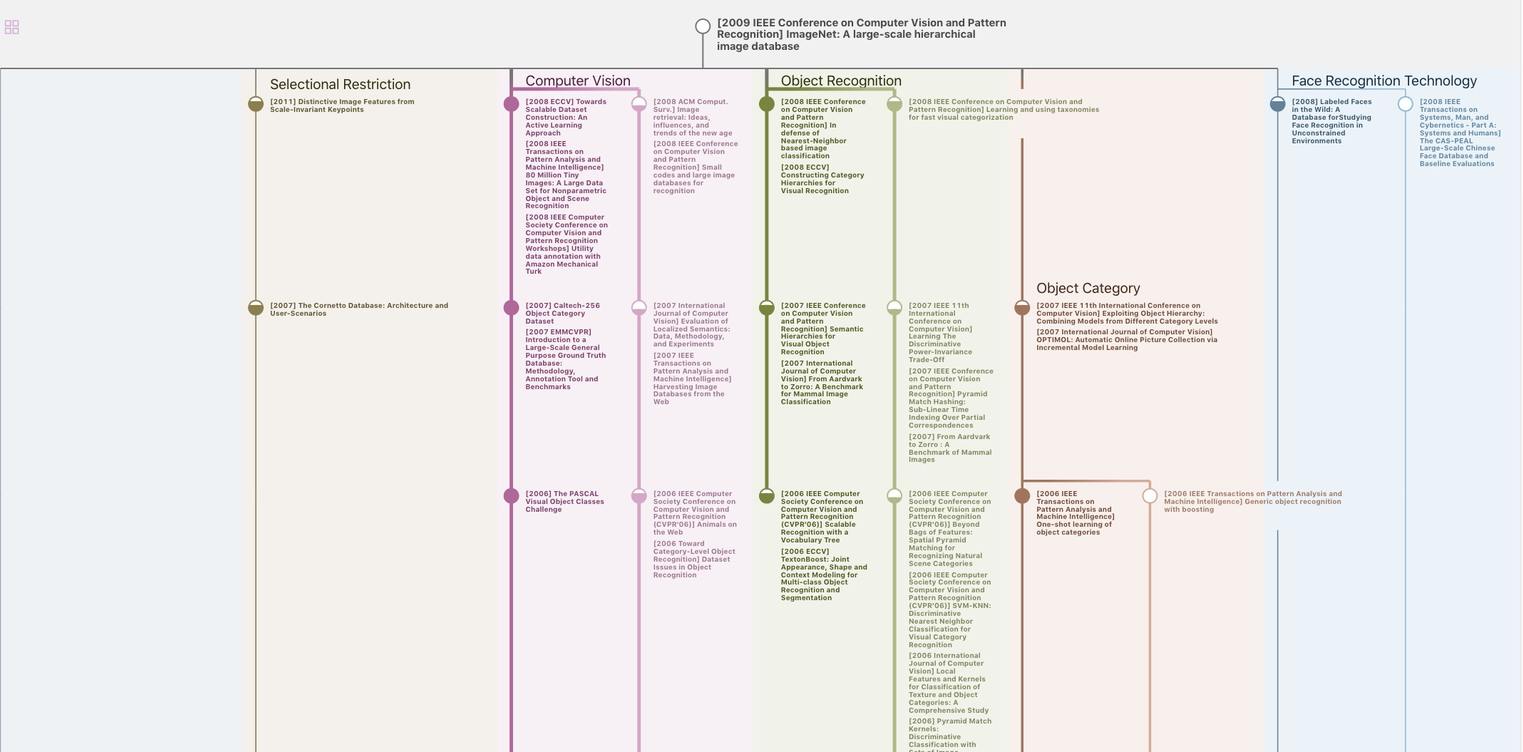
生成溯源树,研究论文发展脉络
Chat Paper
正在生成论文摘要