IFDS: An Intelligent Fault Diagnosis System With Multisource Unsupervised Domain Adaptation for Different Working Conditions
IEEE TRANSACTIONS ON INSTRUMENTATION AND MEASUREMENT(2021)
摘要
Intelligent fault diagnosis is an important subject of mechanical system maintenance. Domain adaptation is a method to solve the problem that the model trained on the training set (source domain) is not suitable for the test set (target domain) due to different working conditions in fault diagnosis. In industrial scenarios, there may be multiple-source domains. For this reason, we proposed an intelligent fault diagnosis system (IFDS) with multisource unsupervised domain adaptive network that adapts to single- or multiple-source domains. The proposed method considers the differences between sources and uses source domain data and a small amount of unlabeled target domain data to mine the feature information contained in the data. IFDS uses a feature extractor to learn the feature representations, constructs a domain discriminator for each source domain, and learns domain-invariant features through adversarial training to diagnose target domain faults. The validity of the method in fault diagnosis is verified by various transfer tasks of two public fault-bearing datasets.
更多查看译文
关键词
Domain adaptation (DA), intelligence fault diagnosis, transfer learning
AI 理解论文
溯源树
样例
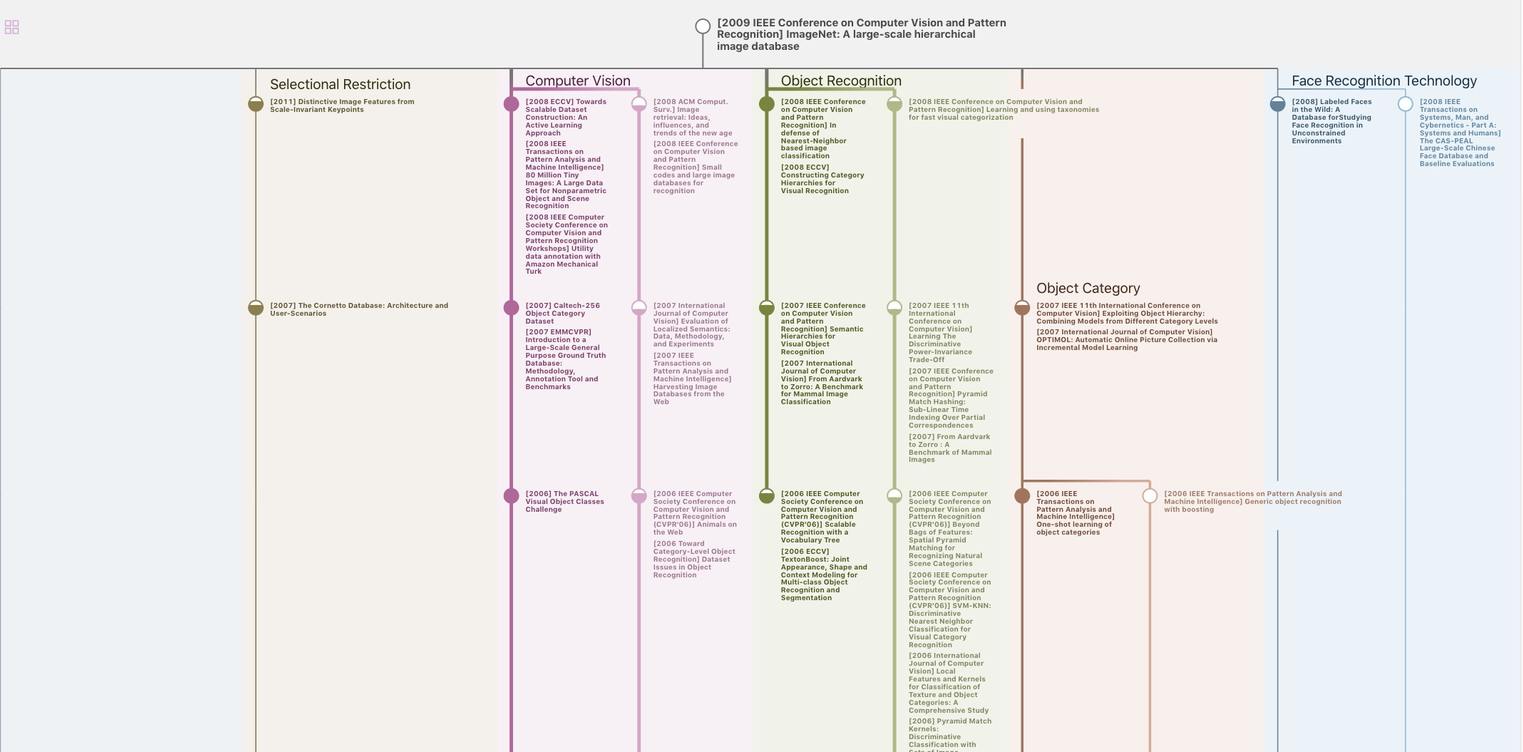
生成溯源树,研究论文发展脉络
Chat Paper
正在生成论文摘要