Autonomous Anomaly Detection and Handling of Spatiotemporal Railway Data
INTERNATIONAL CONGRESS AND WORKSHOP ON INDUSTRIAL AI 2021(2022)
摘要
Prognostics is a vital application for Industrial AI. However, data quality sometimes does not suit regular prediction models. For instance, data sampling procedures occasionally include irregular sampling, and many prognostic methods require evenly sampled time series. One example is measurement trains that travel along the railway line, measuring the geometry of the railway track. In the railway case, the time series often display discontinuities due to maintenance events and outliers. Such discontinuities must be handled differently for a prognostic model to work sufficiently. We have studied different approaches such as moving range statistics and Cook's distance statistics for outlier detection and removal and detection and model adjustments for maintenance. We also provide a practical solution that manages the autonomous anomaly detection and predictions of future track geometry condition through a developed R Shiny App to support users.
更多查看译文
关键词
Time series analysis, Data cleansing, Data filtering, Outlier detection, Prognostics
AI 理解论文
溯源树
样例
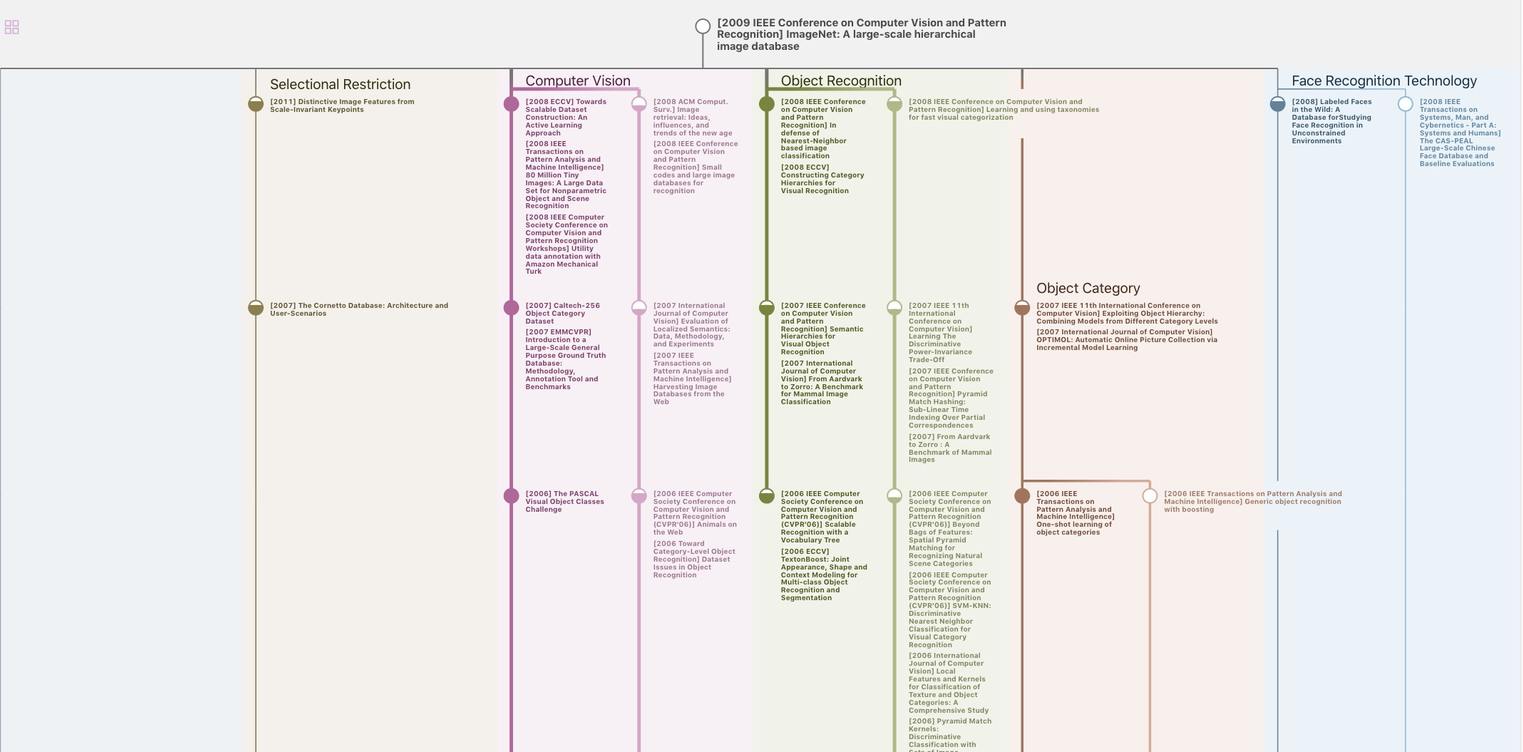
生成溯源树,研究论文发展脉络
Chat Paper
正在生成论文摘要