Semisupervised learning-based depth estimation with semantic inference guidance
Science China Technological Sciences(2022)
摘要
Depth estimation is a fundamental computer vision problem that infers three-dimensional (3D) structures from a given scene. As it is an ill-posed problem, to fit the projection function from the given scene to the 3D structure, traditional methods generally require mass amounts of annotated data. Such pixel-level annotation is quite labor consuming, especially when addressing reflective surfaces such as mirrors or water. The widespread application of deep learning further intensifies the demand for large amounts of annotated data. Therefore, it is urgent and necessary to propose a framework that is able to reduce the requirement on the amount of data. In this paper, we propose a novel semisupervised learning framework to infer the 3D structure from the given scene. First, semantic information is employed to make the depth inference more accurate. Second, we make both the depth estimation and semantic segmentation coarse-to-fine frameworks; thus, the depth estimation can be gradually guided by semantic segmentation. We compare our model with state-of-the-art methods. The experimental results demonstrate that our method is better than many supervised learning-based methods, which proves the effectiveness of the proposed method.
更多查看译文
关键词
depth estimation, semisupervised learning, semantic information, neural networks
AI 理解论文
溯源树
样例
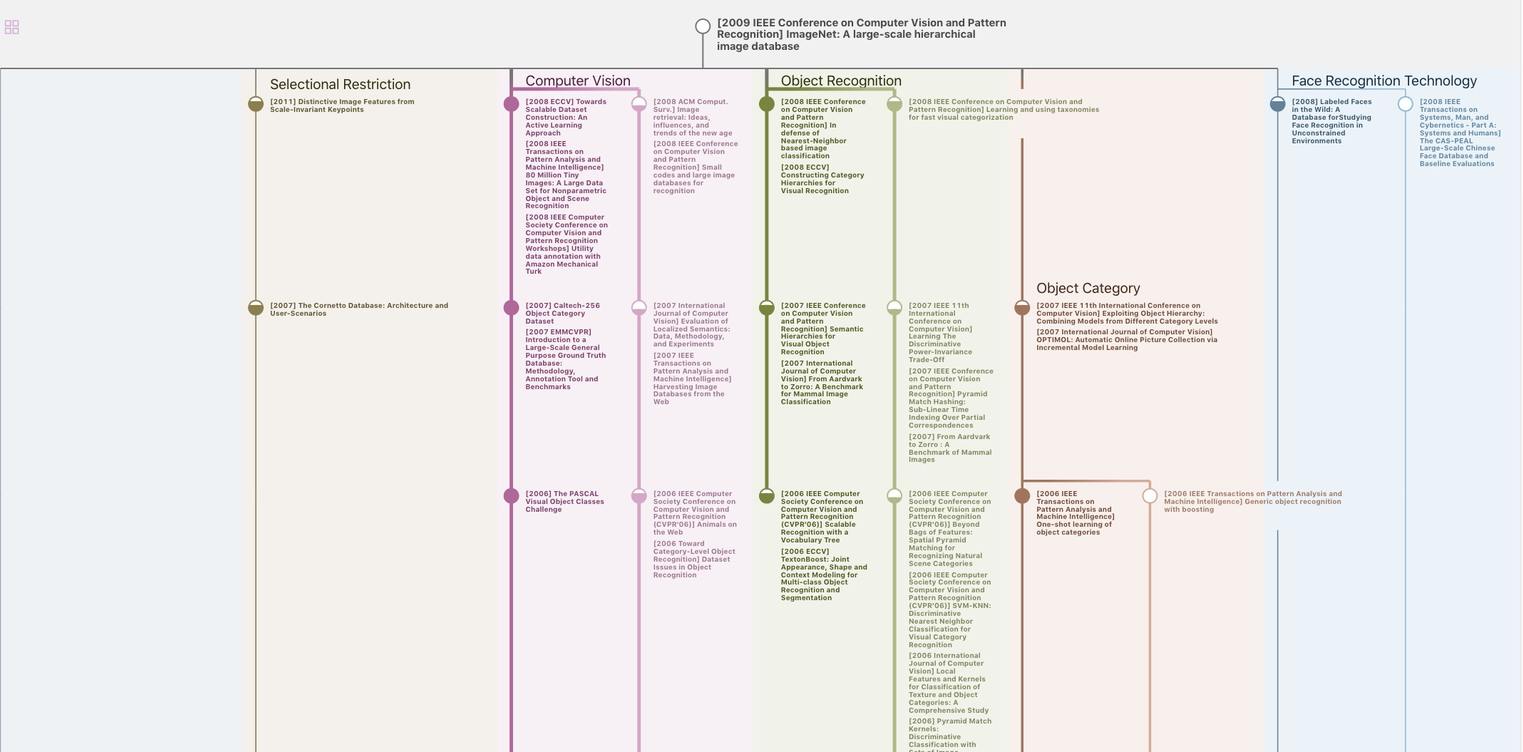
生成溯源树,研究论文发展脉络
Chat Paper
正在生成论文摘要