A stacking ensemble algorithm for improving the biases of forest aboveground biomass estimations from multiple remotely sensed datasets
GISCIENCE & REMOTE SENSING(2022)
摘要
Accurately quantifying the aboveground biomass (AGB) of forests is crucial for understanding global change-related issues such as the carbon cycle and climate change. Many studies have estimated AGB from multiple remotely sensed datasets using various algorithms, but substantial uncertainties remain in AGB predictions. In this study, we aim to explore whether diverse algorithms stacked together are able to improve the accuracy of AGB estimates. To build the stacking framework, five base learners were first selected from a series of algorithms, including multivariate adaptive regression splines (MARS), support vector regression (SVR), multilayer perceptron (MLP) model, random forests (RF), extremely randomized trees (ERT), stochastic gradient boosting (SGB), gradient-boosted regression tree (GBRT) algorithm, and categorical boosting (CatBoost), based on diversity and accuracy metrics. Ridge and RF were utilized as the meta learner to combine the outputs of base learners. In addition, six important features were selected according to the feature importance values provided by the CatBoost, ERT, GBRT, SGB, MARS and RF algorithms as inputs of the meta learner in the stacking process. We then used stacking models with 3-5 selected base learners and ridge or RF to estimate AGB. The AGB data compiled from plot-level forest AGB, high-resolution AGB data derived from field and lidar data and the corresponding predictor variables extracted from the satellite-derived leaf area index, net primary production, forest canopy height, tree cover data, and Global Multiresolution Terrain Elevation Data 2010, as well as climate data, were randomly split into groups of 80% for training the model and 20% for model evaluation. The evaluation results showed that stacking generally outweighed the optimal base learner and provided improved AGB estimations, mainly by decreasing the bias. All stacking models had relative improvement (RI) values in bias of at least 22.12%, even reaching more than 90% under some scenarios, except for deciduous broadleaf forests, where an optimal algorithm could provide low biased estimations. In contrast, the improvements of stacking in R-2 and RMSE were not significant. The stacking of MARS, MLP, and SVR provided improved results compared with the optimal base learner, and the average RI in R-2 was 3.54% when we used all data without separating forest types. Finally, the optimal stacking model was used to generate global forest AGB maps.
更多查看译文
关键词
Stacking, forest biomass, ensemble algorithm, remote sensing
AI 理解论文
溯源树
样例
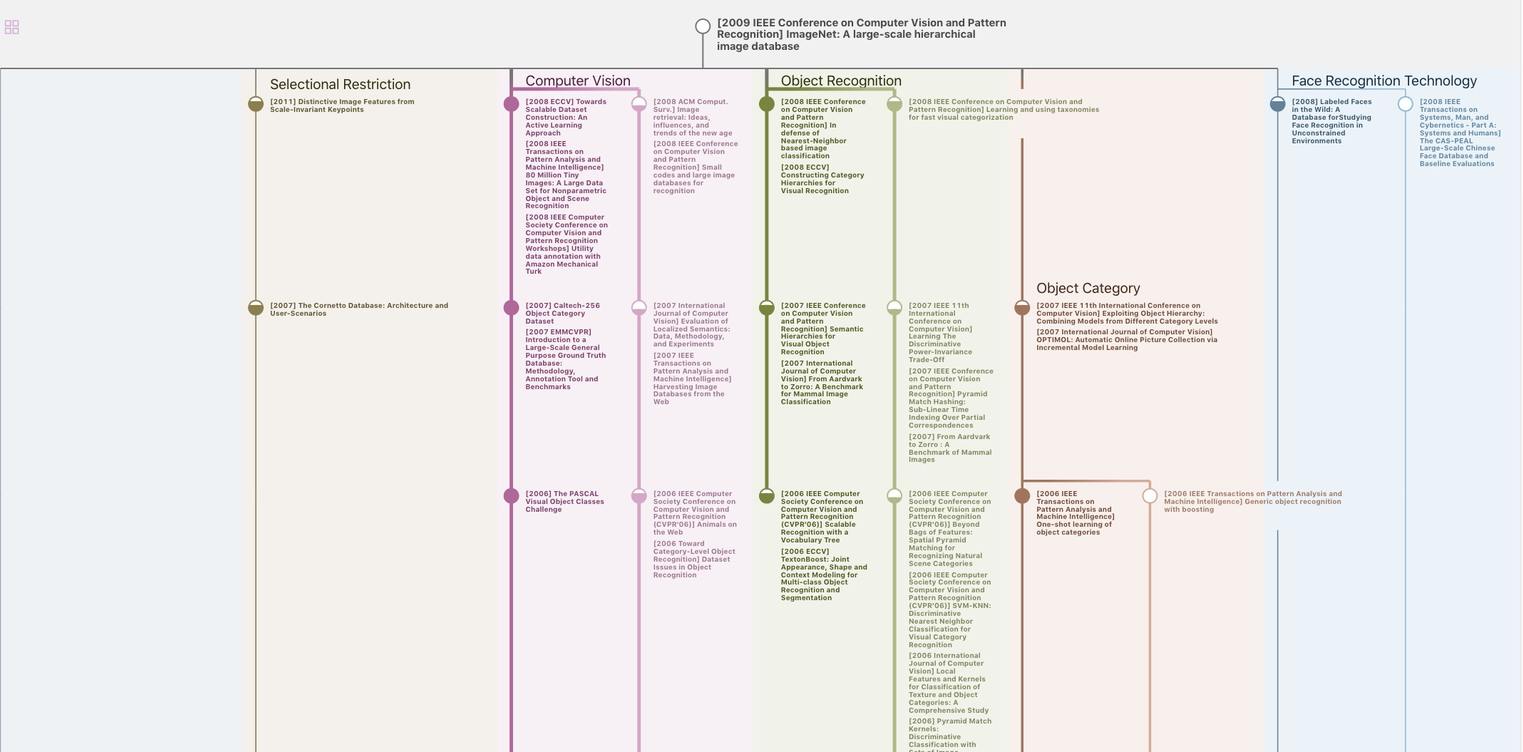
生成溯源树,研究论文发展脉络
Chat Paper
正在生成论文摘要