One-Class Classifier for Chest X-Ray Anomaly Detection via Contrastive Patch-Based Percentile
IEEE ACCESS(2021)
摘要
Given its low dose and compactness, chest radiography has been widely used as the first-line test to determine the presence of lung anomalies. Nevertheless, a high-performance diagnosis for initial screening to detect shadows in lungs due to general lung diseases is not available. During initial screening, chest radiography can be used to distinguish any diseased lung shadowing caused by lung diseases. Thus, chest radiography can contribute to the early diagnosis and prevention of novel lung infectious diseases if training for a specific disease is not required. Accordingly, we propose a deep-learning-based diagnostic system called contrast-shifted instances via patch-based percentile (CSIP) to automatically detect diseased lung shadowing via training only on chest X-ray data from healthy subjects. CSIP is the first application of a patch-based percentile approach to state-of-the-art one-class classifiers (OCCs). This application improves the sensitivity of the network to recognize shadowing density differences in each local area of the lung, thereby considerably improving the diagnostic performance of average area under the curve (AUC) by more than 20% and achieving a sufficiently high diagnostic performance (average AUC of 0.96 for various lung diseases), compared to the existing OCC case without applying our patch-based approach (average AUC of 0.74). Therefore, CSIP may contribute to the early detection of anomalies caused by novel infectious diseases such as variants of the coronavirus disease, for whom training data are scarce. The code is available at https://github.com/kskim-phd/CSIP.
更多查看译文
关键词
Lung,Diseases,Anomaly detection,Training,Computed tomography,COVID-19,Pulmonary diseases,Artificial intelligence,unsupervised learning,diagnostic radiography,X-ray detection,anomaly detection,contrastive learning,one-class classifier,patch-based percentile,shifted instances
AI 理解论文
溯源树
样例
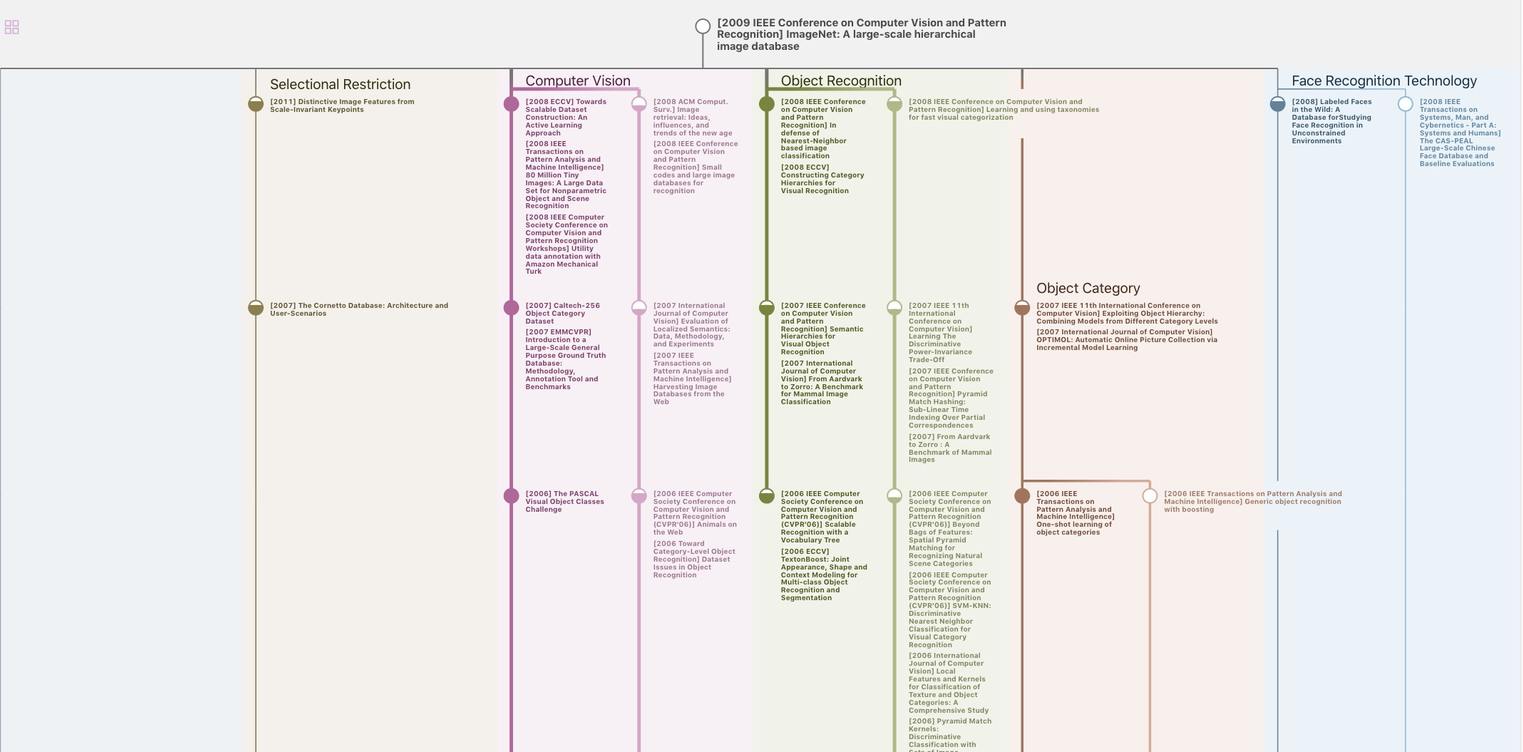
生成溯源树,研究论文发展脉络
Chat Paper
正在生成论文摘要